Document processing
9 min read
—
Dec 13, 2024
Learn about the future of AI in underwriting and its real-world applications, from streamlining submission ingestion to automated claims processing.
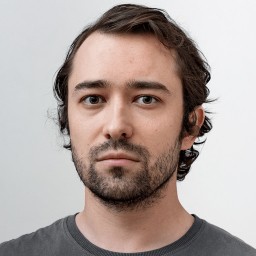
Content Creator
In 1693, Edmond Halley created one of the earliest life tables, transforming raw demographic records into predictive insights that could underpin life annuities. His work did not merely advance mathematics—it established the principles of modern actuarial science, embedding calculation into the fabric of risk management.
Today, the industry stands on the brink of another revolution of similar magnitude. Just as Halley’s tables harnessed probability to illuminate the unknown, artificial intelligence promises to unlock new dimensions of precision, speed, and personalization in insurance underwriting. But as with any breakthrough, the real story lies not in the promise of technology but in its application.
In this article:
The impact of generative AI on the industry
Examples of how AI is used in insurance underwriting
Key technologies, platforms, and tools for claims processing
Challenges of practical implementation of underwriting AI
Can AI truly deliver on its transformative potential without losing the trust and nuance that underpin the insurance business?
Beneath the headlines and hype lies a more nuanced reality: one where AI's promise meets practical limitations, and where success depends not on wholesale automation but on thoughtful integration with human expertise.
Understanding the role of AI in insurance underwriting
Machine learning has quietly powered the insurance industry for years, albeit in a largely “unsexy” way. Behind the scenes, it has been the workhorse for complex calculations, predictive modeling, and risk analysis—useful but hardly groundbreaking.
Now, generative AI changed the game.
Unlike traditional ML, generative AI isn’t just about crunching numbers. It's about understanding and generating language. This new wave of AI models like GPT and Claude can understand words, sentences, and even entire books. And, incidentally, it can read and understand actuarial reports, medical records, claims histories, and policy wordings. With generative AI, document automation isn’t just faster—it sets new benchmarks for what’s possible.
Do insurance companies actually use AI?
Recent data from Conning reveals that 69% of underwriting teams are piloting large language models. These new AI models have already surpassed the adoption rates of process automation and intelligent document processing software, which were considered state-of-the-art before the advent of generative AI and LLMs. This reflects a significant shift in how AI is being integrated into the insurance industry.
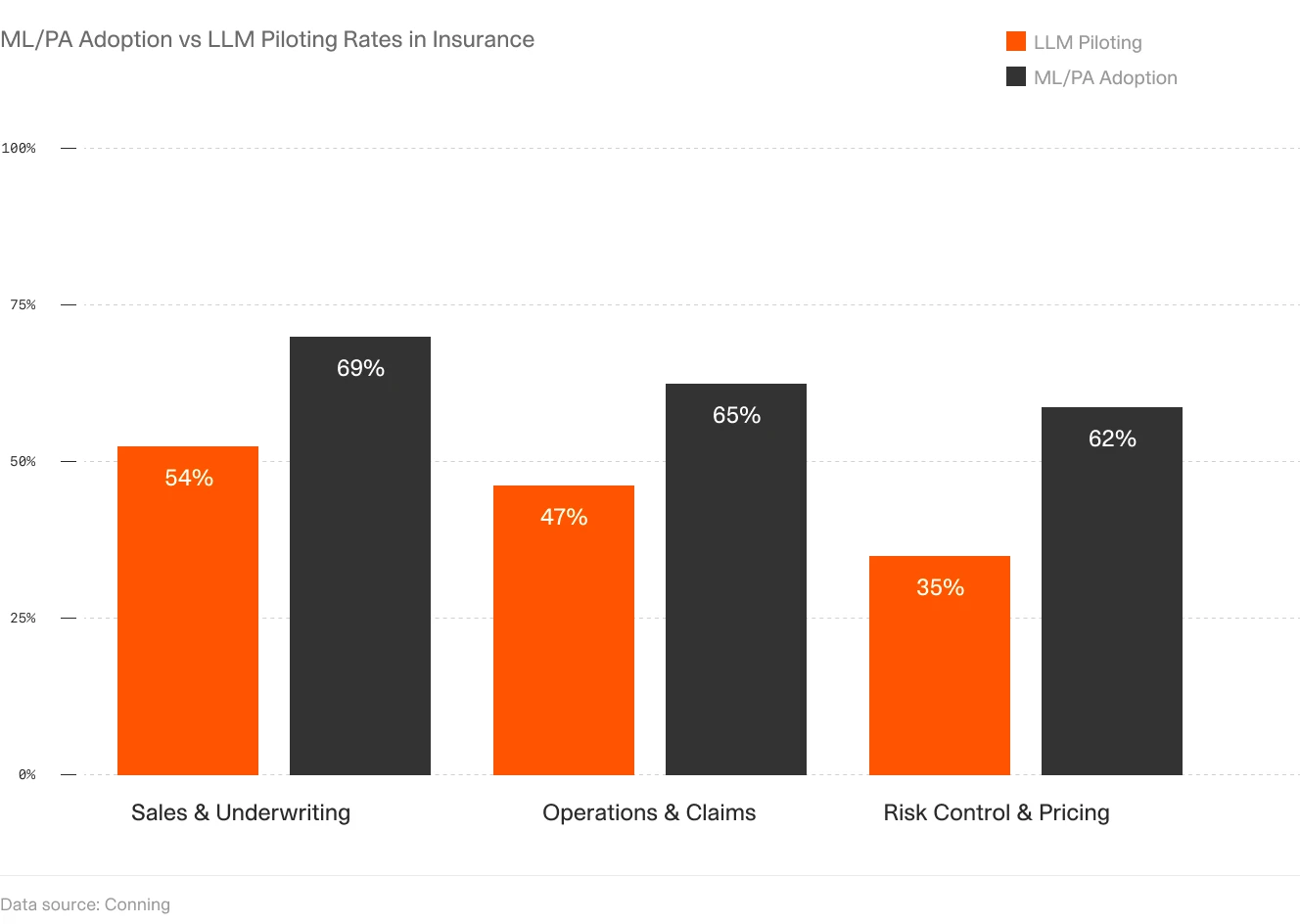
But these figures only scratch the surface. Among the top players, the adoption rate is at 100%.
Yes, you read that right.
Every one of the top 25 insurance carriers are either actively deploying at least one AI application or have ongoing AI development projects in progress. This level of engagement underscores a critical point: for major insurers, AI is no longer optional—it’s a cornerstone of their strategy.
The global market for AI in insurance, valued at $2.74 billion in 2021, is projected to reach $45.74 billion by 2031. This trajectory reflects not just growing adoption but a fundamental reimagining of how insurance risk is assessed and managed.
What does AI bring to the table and why are insurance companies adopting it so eagerly?
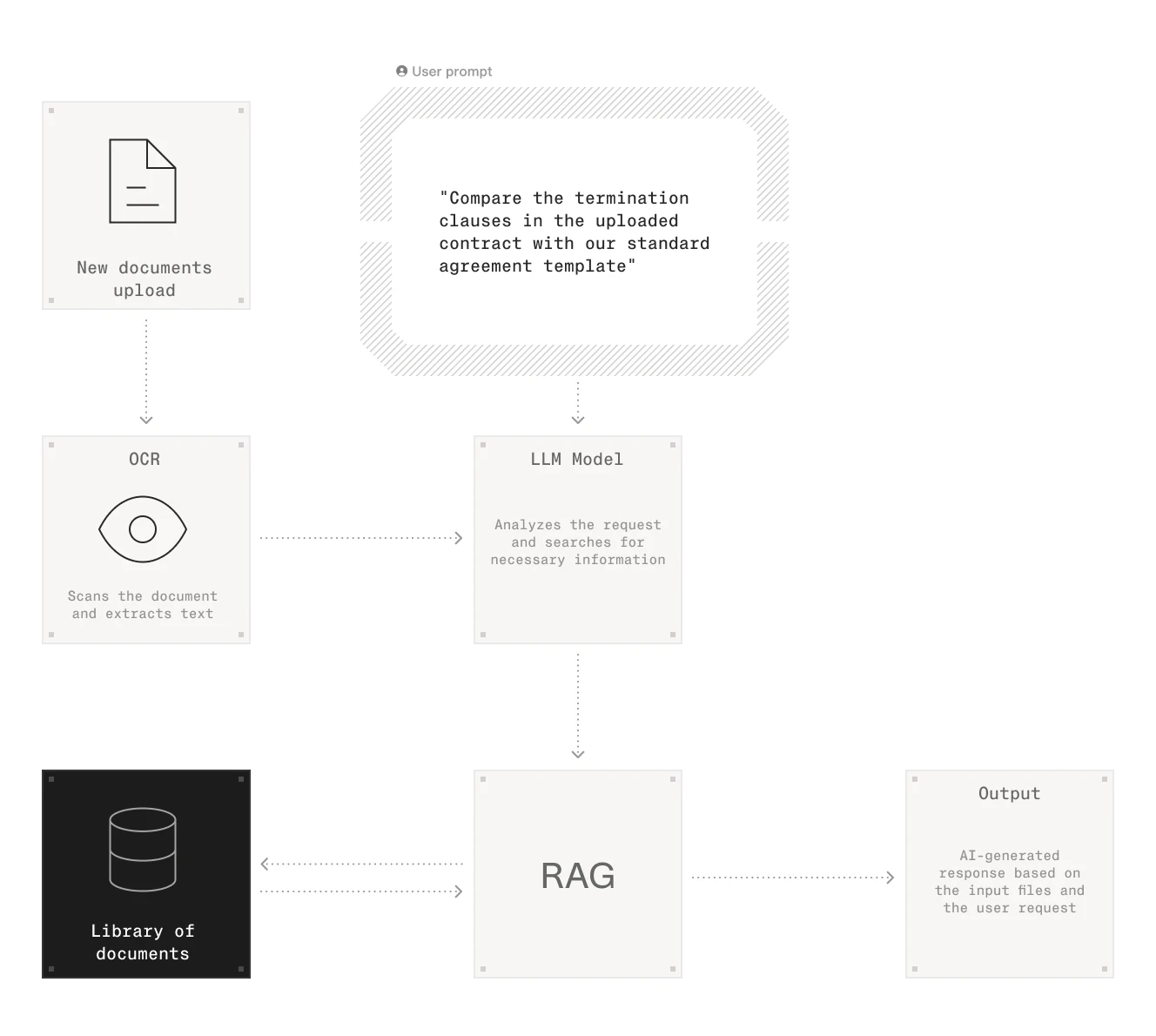
At its core, AI enables insurers to process vast quantities of structured and unstructured data with unparalleled speed and precision. Technologies like natural language processing (NLP) and generative AI are stepping in to automate the tedious tasks underwriters once performed manually. From scanning thousands of pages in an application to evaluating actuarial data for risk predictions, AI streamlines operations to unprecedented levels.
So, one of the big questions in the back of every insurance professional head is: can AI replace actuaries or brokers?
While AI can automate repetitive and data-heavy tasks, it lacks the human ability to consider ethical implications or navigate ambiguous cases. Actuaries, brokers, and underwriters are vital cogs in the insurance wheel, and their expertise lies in applying nuanced judgment to complex scenarios.
To borrow a quote and make it more relevant to our industry: AI won’t replace underwriters, but underwriters who use AI will outcompete those who don’t. The focus, then, is on creating a symbiotic relationship where AI handles the grunt work, leaving professionals to focus on strategy, client interaction, and regulatory compliance.
AI insurance underwriting examples and key use cases
While the potential sounds promising, the real value of AI emerges in its practical applications. Here are seven key areas where artificial intelligence is already transforming insurance operations, delivering measurable improvements in efficiency and accuracy.
1. Submission ingestion
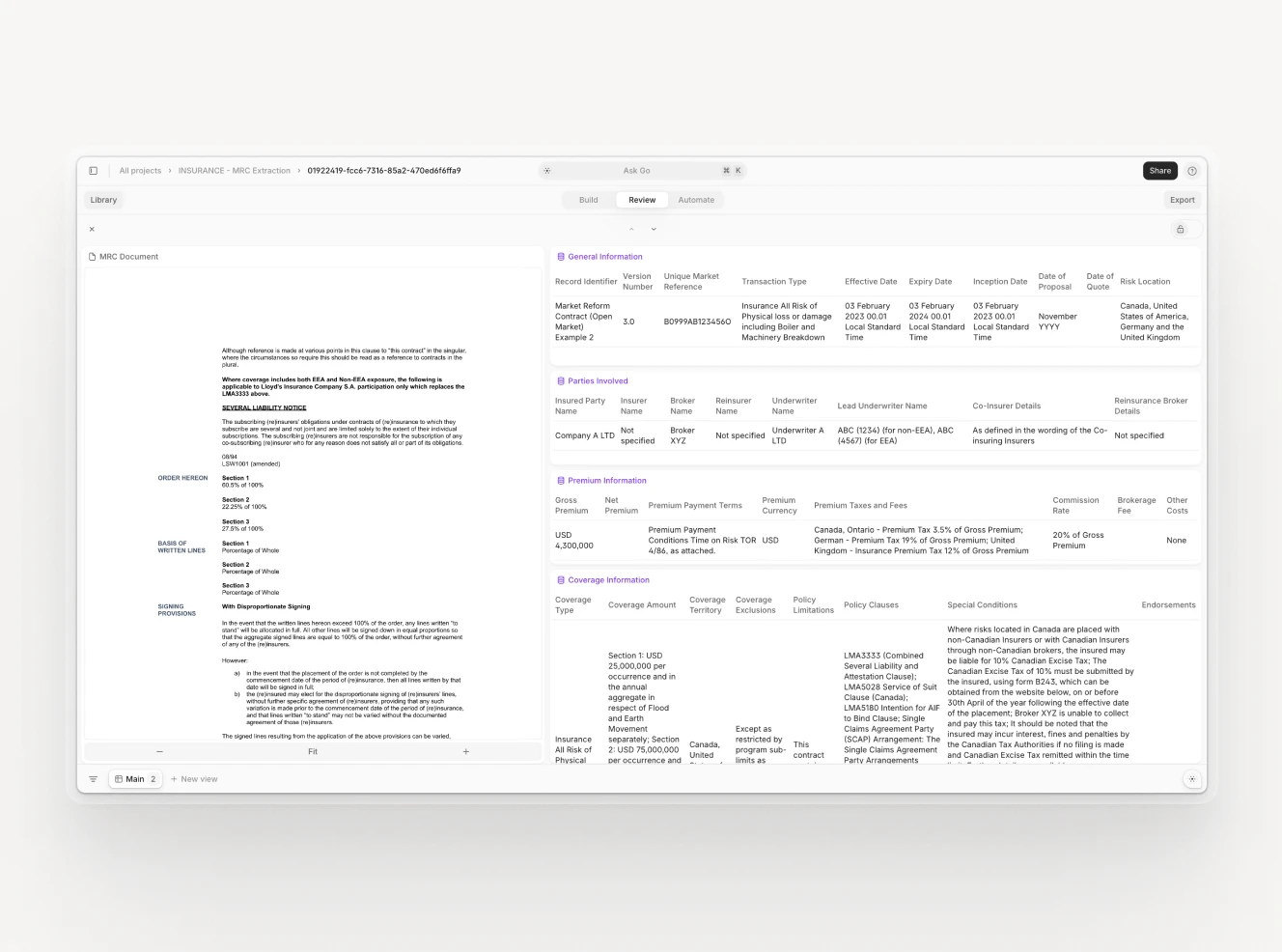
Challenge:
Insurers receive submissions from brokers in varying formats, such as Market Reform Contracts (MRCs), Schedules of Values (SOVs), and loss runs. Processing these manually is slow and error-prone, requiring underwriters to sift through unstructured data, input details into multiple systems, and normalize formats. This bottleneck often delays quotes and hampers competitiveness.
What AI Can Do:
AI platforms like V7 Go use advanced document automation technologies, including natural language processing, large language models, and optical character recognition, to extract critical data points from documents. The data is standardized, validated, and seamlessly integrated into downstream systems like policy administration software (PAS). AI also triages submissions, prioritizing those aligned with the insurer’s risk appetite.
Impact:
Reduces manual data entry and processing times by up to 80%.
Increases data accuracy, minimizing errors caused by manual oversight.
Accelerates quote turnaround times, enhancing competitiveness in winning new business.
Example:
AI enables insurers to process MRCs and associated documents in seconds, extracting essential data such as policy terms, limits, and exclusions. Insurers using this technology report significant reductions in operational bottlenecks and increased client satisfaction.
2. Asset and collateral valuation
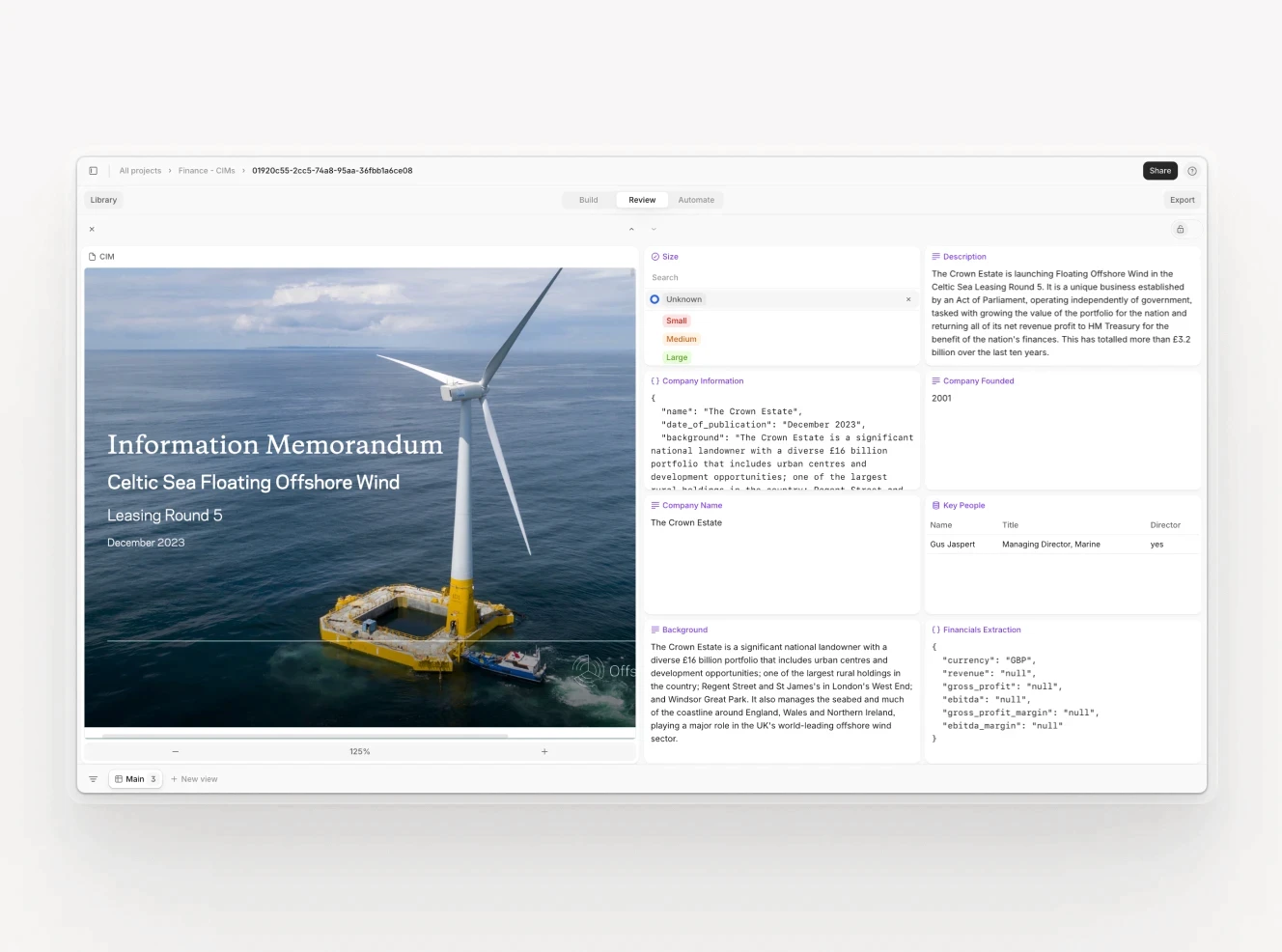
Challenge:
Valuing insured assets such as properties or high-value items requires detailed assessments, often combining geospatial data, historical risk patterns, and current market conditions. Traditional methods are labor-intensive and prone to inaccuracies.
What AI Can Do:
AI integrates geospatial data, satellite imagery, and IoT sensor inputs to evaluate asset conditions and exposures in real time. Advanced analytics models quantify risks, standardize valuation metrics, and highlight high-risk assets.
Impact:
Produces faster and more accurate valuations, reducing underwriting delays.
Enhances portfolio risk visibility, helping insurers optimize pricing.
Identifies anomalies or trends, enabling proactive risk management.
Example:
AI solutions can leverage satellite imagery and environmental data to deliver precise property valuations, allowing underwriters to refine their pricing strategies and avoid exposure blind spots.
3. Pricing and actuarial analysis
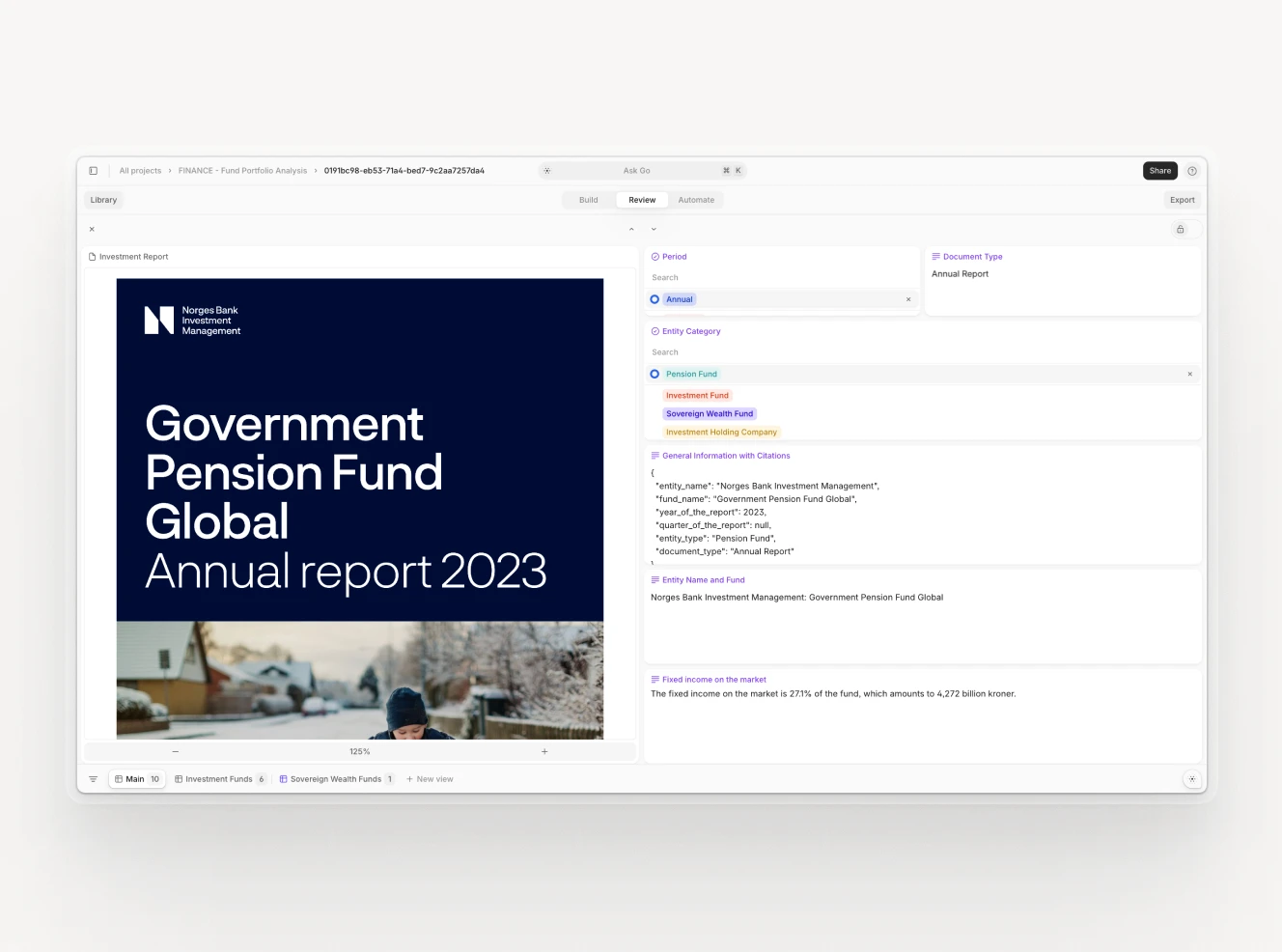
Challenge:
Actuarial pricing relies on processing vast datasets, including historical claims and predictive variables such as weather and customer behavior. Conventional methods struggle to adjust dynamically, leading to inefficient pricing models.
What AI Can Do:
AI employs machine learning algorithms to analyze historical claims data alongside external factors like demographic trends and natural disasters. These models generate dynamic pricing strategies and run simulations to optimize premium structures based on real-time risk factors.
Impact:
Improves pricing accuracy, minimizing loss ratios.
Enables hyper-personalized policies, increasing customer retention.
Reduces reliance on outdated, static pricing models.
Example:
AI-driven pricing solutions adjust premiums dynamically, factoring in behavior-based risk assessments to deliver competitive, fair pricing.
4. Endorsements processing
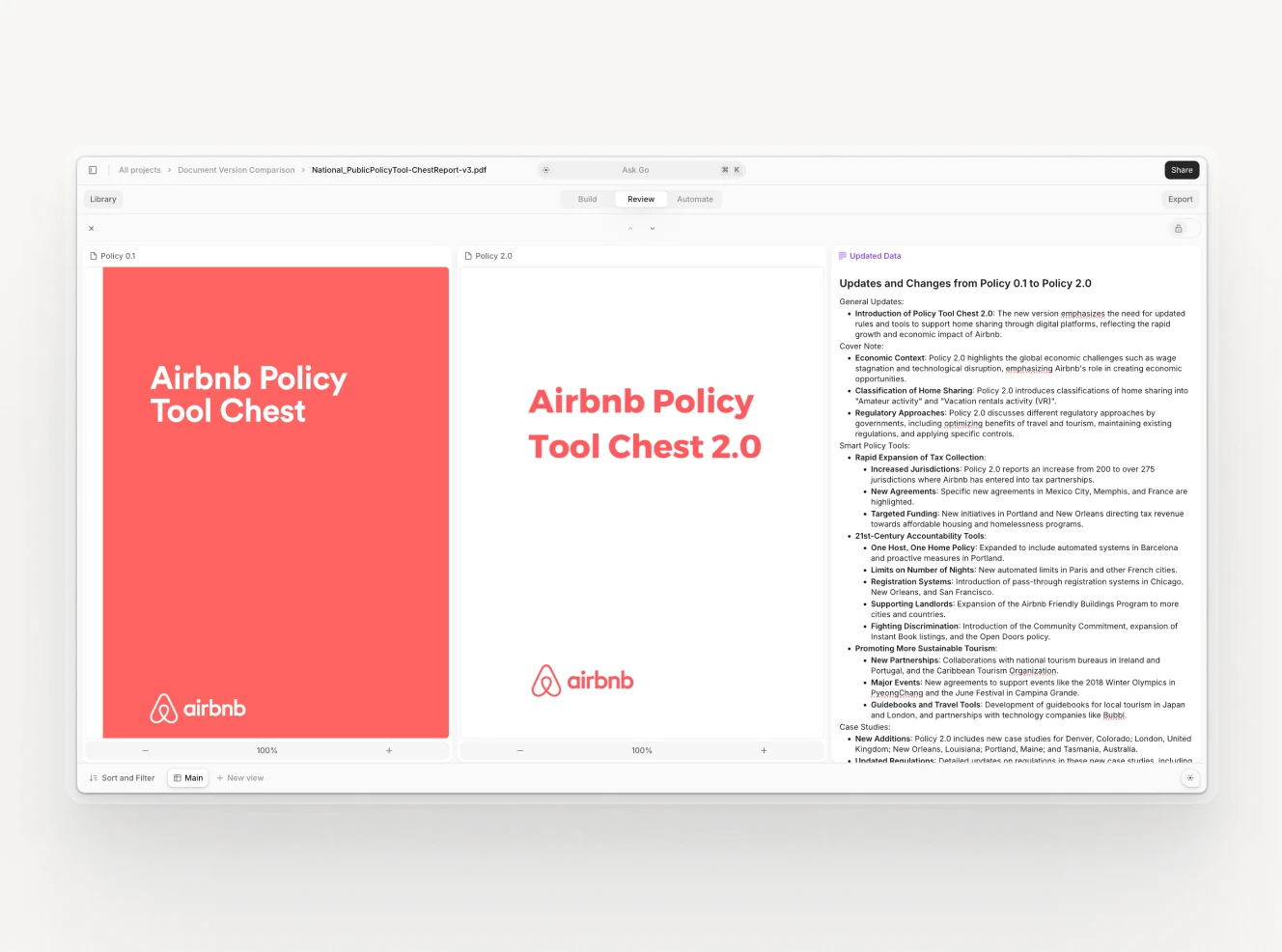
Challenge:
Policy changes, such as adding coverage or adjusting terms, generate endorsements that must be manually processed. This can delay billing for premium adjustments and increase administrative workload.
What AI Can Do:
AI automates the identification, extraction, and categorization of endorsements. Tools like V7 Go can use LLMs to analyze and update policy systems with changes in real time, eliminating redundant manual processes and ensuring timely premium adjustments.
Impact:
Reduces processing time for endorsements by up to 60%.
Ensures accurate and timely premium billing.
Frees up underwriters for higher-value tasks.
Example:
AI-powered solutions have streamlined endorsements workflows, allowing insurers to rapidly implement changes while maintaining operational accuracy and efficiency.
5. Risk engineering reports
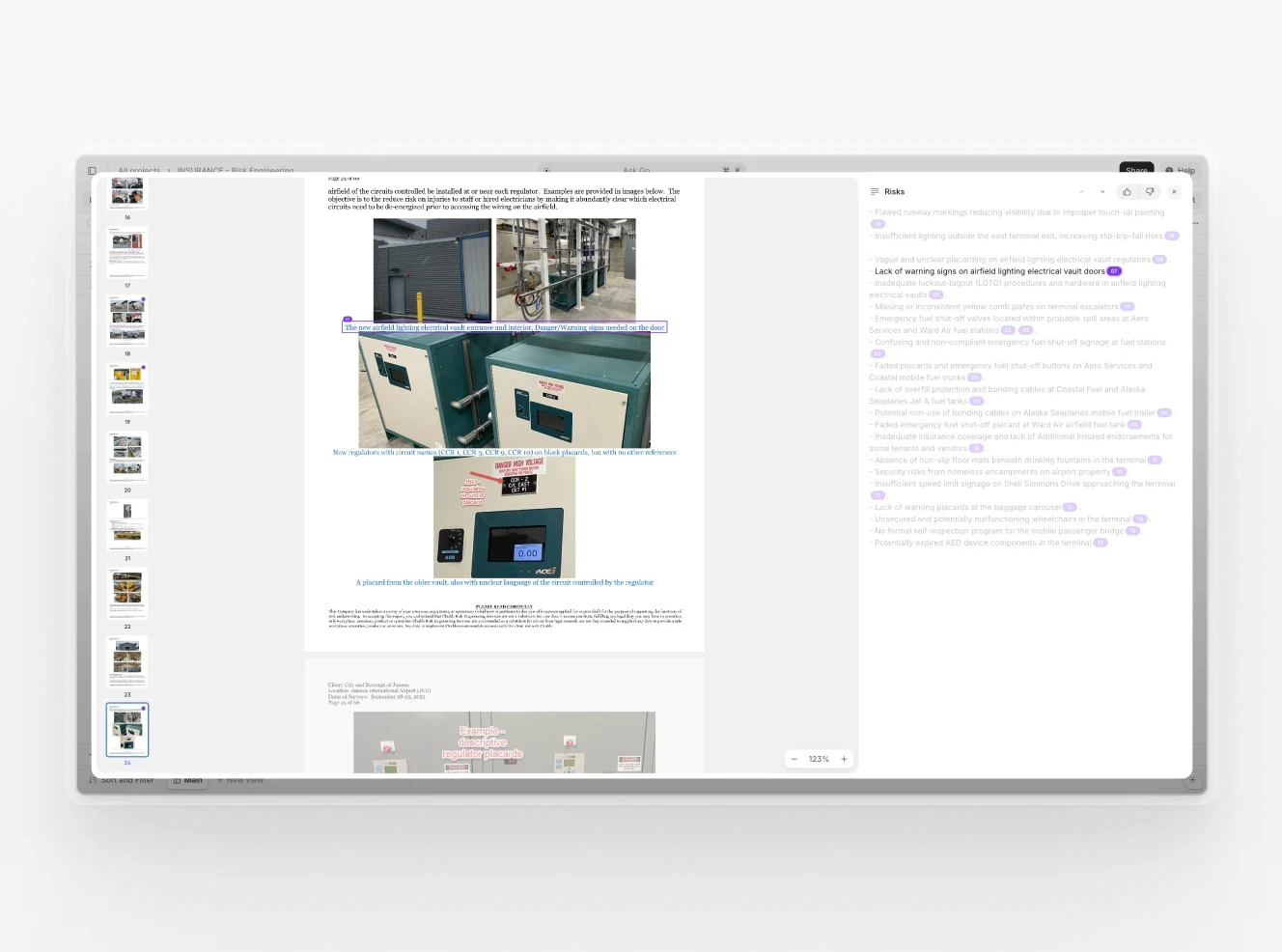
Challenge:
Risk engineering reports, which provide in-depth analyses of insured properties, are often lengthy and dense with technical details. Underwriters may overlook valuable insights due to time constraints or inconsistent report formats.
What AI Can Do:
AI-powered platforms extract, summarize, and standardize key insights from risk engineering reports. This includes identifying critical risk factors, translating diverse grading systems, and presenting actionable data for underwriters.
Impact:
Saves underwriters hours of manual review time.
Standardizes diverse grading methodologies for better decision-making.
Improves utilization of engineering insights, uncovering pricing opportunities.
Example:
AI systems process risk reports quickly, summarizing key data points and aligning them with reinsurance compliance requirements.
6. Reinsurance processing
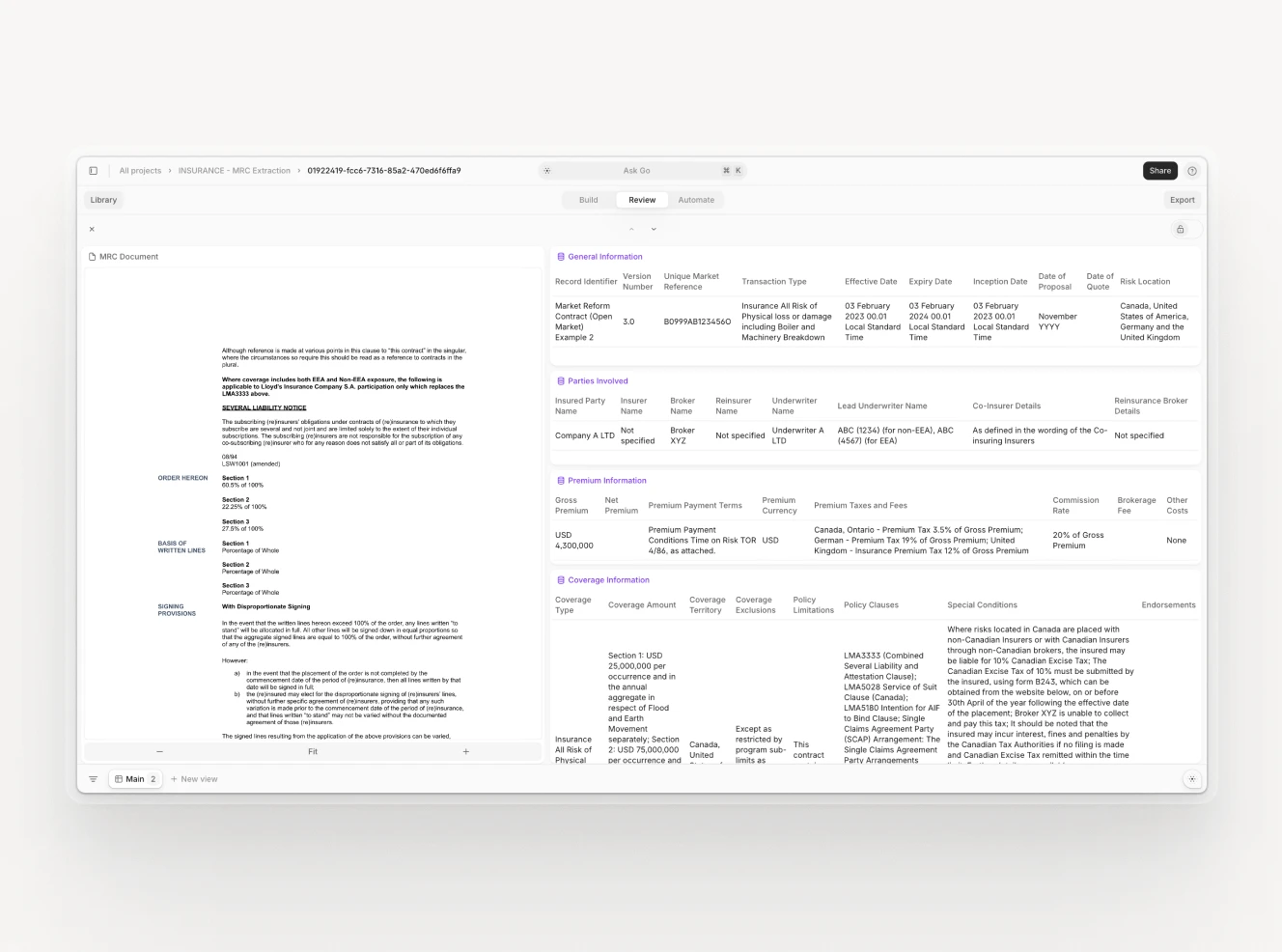
Challenge:
Reinsurers face inefficiencies in processing treaty documents and bordereaux due to non-standardized formats and repetitive manual data entry.
What AI Can Do:
AI automates the ingestion, normalization, and reconciliation of reinsurance data. Intelligent platforms integrate seamlessly with reinsurance management systems, enabling faster processing and enhanced accuracy.
Impact:
Standardizes data from diverse sources, improving operational efficiency.
Enhances reconciliation accuracy, reducing errors in treaty management.
Streamlines workflows, reducing overhead costs.
Example:
Reinsurers leveraging AI solutions process thousands of bordereaux monthly with fewer manual interventions, significantly cutting operational expenses.
7. Claims automation
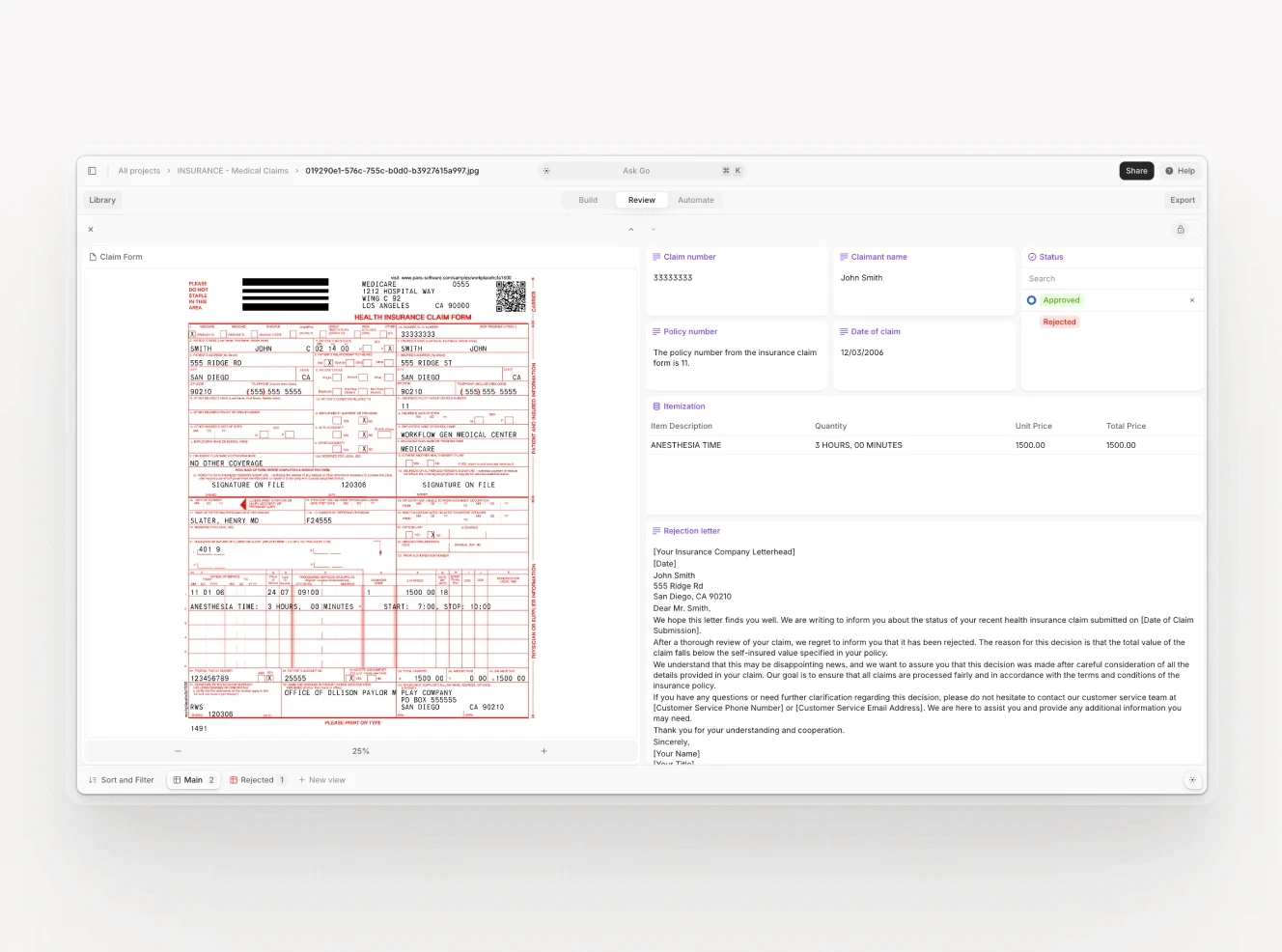
Challenge:
Claims handling involves reviewing multiple document types—claim forms, medical reports, police narratives—and often leads to bottlenecks in resolution times, frustrating customers.
What AI Can Do:
AI systems triage claims by severity, extract relevant information from documents, and create summaries for review. They integrate directly with claims management systems, routing claims efficiently and flagging potential fraud.
Impact:
Reduces claim resolution times by up to 50%.
Enhances customer satisfaction through faster settlements.
Improves accuracy in detecting fraudulent claims.
Example:
Using V7 Go’s automation for claims processing, insurers have expedited settlements while reducing operational costs and improving customer retention.
Understanding these use cases naturally raises a question: which tools can actually deliver on these promises? Several platforms have emerged as leaders in this space, each bringing unique capabilities to address different aspects of the underwriting process.
Best underwriting AI solutions
Several AI solutions have emerged as leaders in enhancing the underwriting process, making it more efficient, accurate, and customer-friendly. Here are the best AI solutions for insurance underwriting this year:
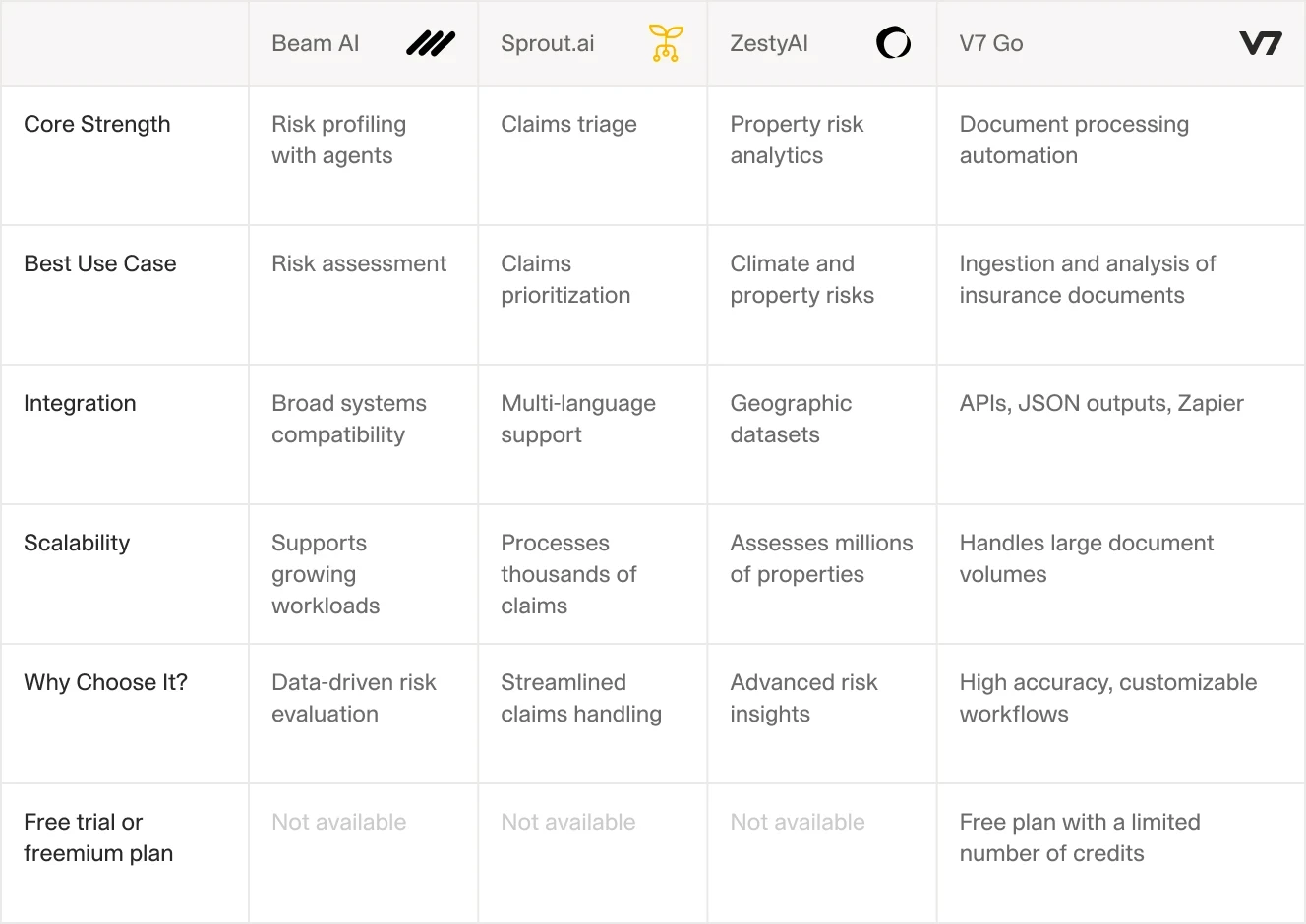
Understanding these platforms at a high level provides a useful starting point, but the real value lies in examining how each solution addresses specific insurance challenges.
Let's take a deeper look at how these leading platforms are transforming different aspects of insurance and underwriting operations.
V7 Go: the new standard in AI claims processing
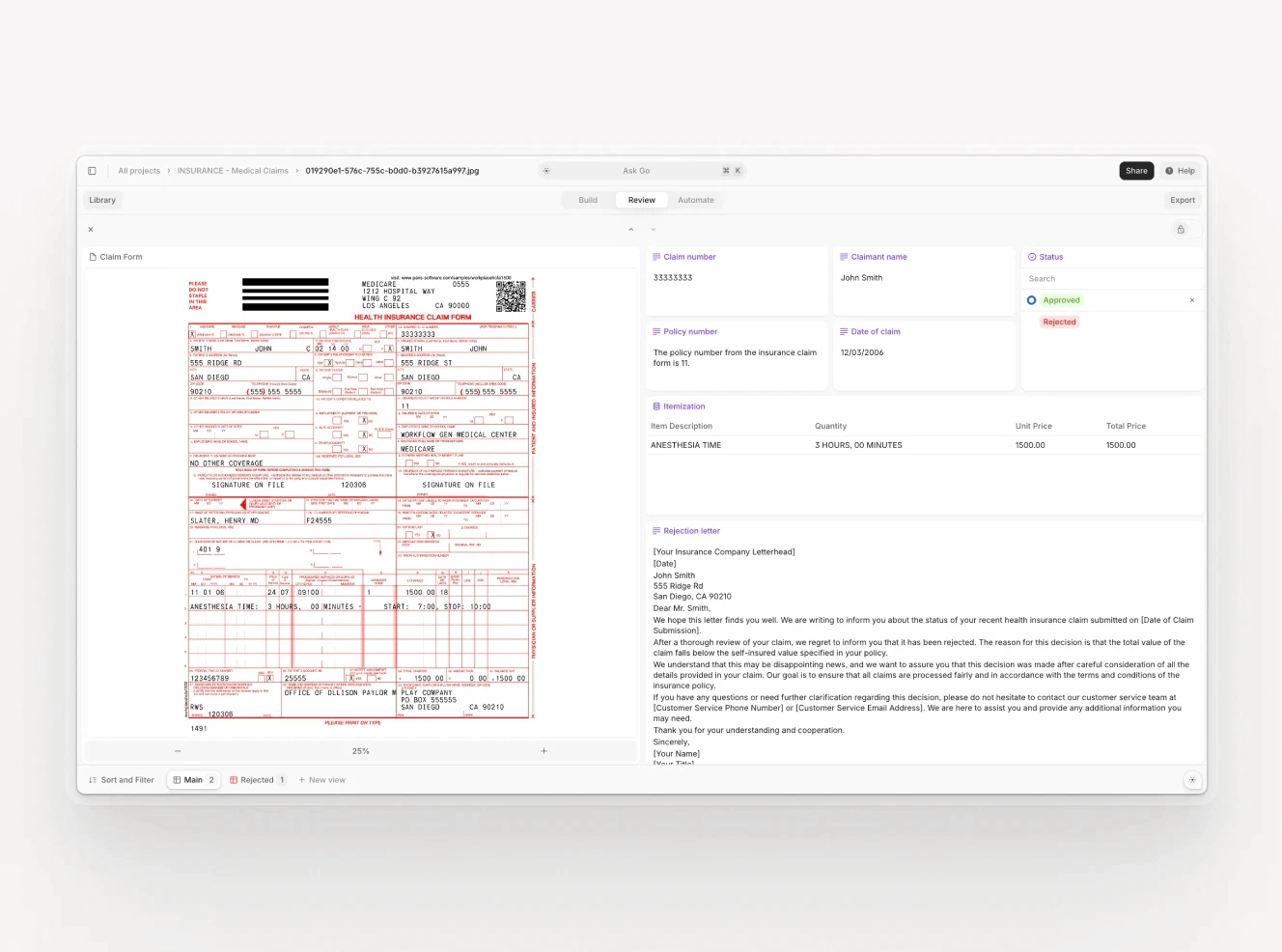
Launched in April 2024, V7 Go represents the latest evolution in insurance document automation. Born from V7's expertise in ML data labeling since 2018, this platform has quickly established itself as a comprehensive solution for insurance document processing and data extraction.
V7 Go's standout feature is its AI Citations system, which provides unprecedented transparency by linking every extracted data point to its source document. This addresses one of the fundamental challenges in AI adoption: trust and verification. The platform processes everything from complex claims files to multi-party legal documents, maintaining contextual understanding throughout.
Key features:
Document processing. Handles complex claims, including legal files and multi-party cases, with detailed summaries and analysis.
AI Citations. Ensures transparency by linking data points to sources like medical reports or police files.
Data extraction. Extracts structured data from tables and graphs, preserving relationships between data points.
Security. Certified for SOC2, HIPAA, and ISO standards, ensuring safe handling of sensitive claims.
Access control. Offers customizable roles for managing team access across regions and departments.
Quick integration. Connects to claims systems via API, enabling setup within days.
For insurers seeking rapid deployment, V7 Go offers a compelling proposition: operational readiness within seven days. Its tiered pricing structure, starting with a free plan that includes 250 fields and $15 worth of tokens, makes it accessible to organizations of all sizes. The self-serve plan at $249 monthly provides expanded capabilities with 25,000 fields, while the enterprise tier offers custom configurations with dedicated AI engineering support.
ZestyAI: pioneering AI risk assessment
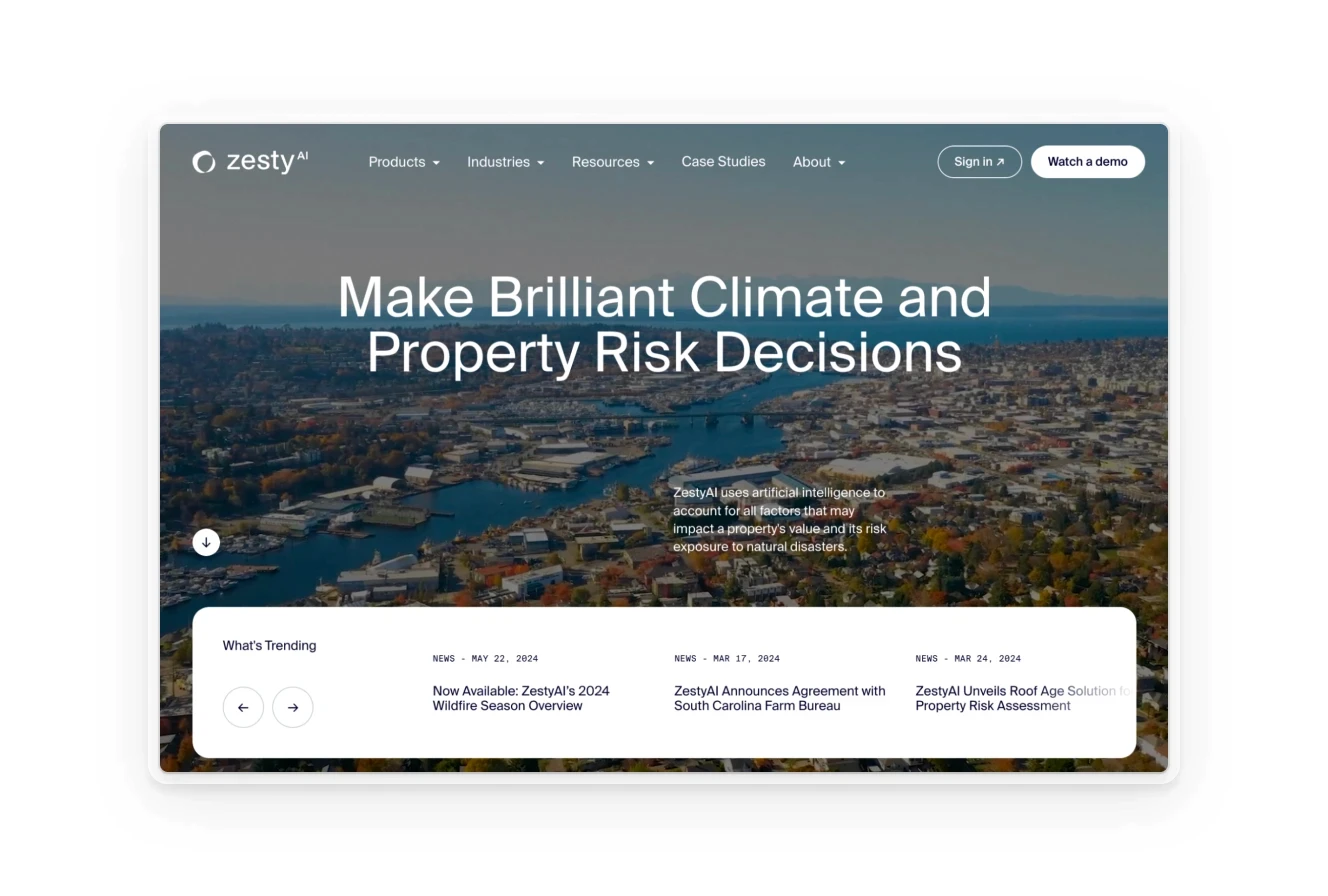
Founded in 2015, ZestyAI has transformed from its PowerScout origins into a powerhouse in property and climate risk analytics. The platform's ability to process over 200 billion data points sets it apart in the industry. ZestyAI combines aerial imagery analysis, weather pattern data, and historical loss information to deliver granular property-level risk insights.
The company's expertise in climate-related perils—from wildfires to floods—has attracted major industry players. Their success story includes a $23 million Series B funding round in 2022, validating their approach to risk assessment. While pricing isn't publicly disclosed, their platform offers customizable solutions based on insurer needs.
Sprout.ai: accelerating claims automation
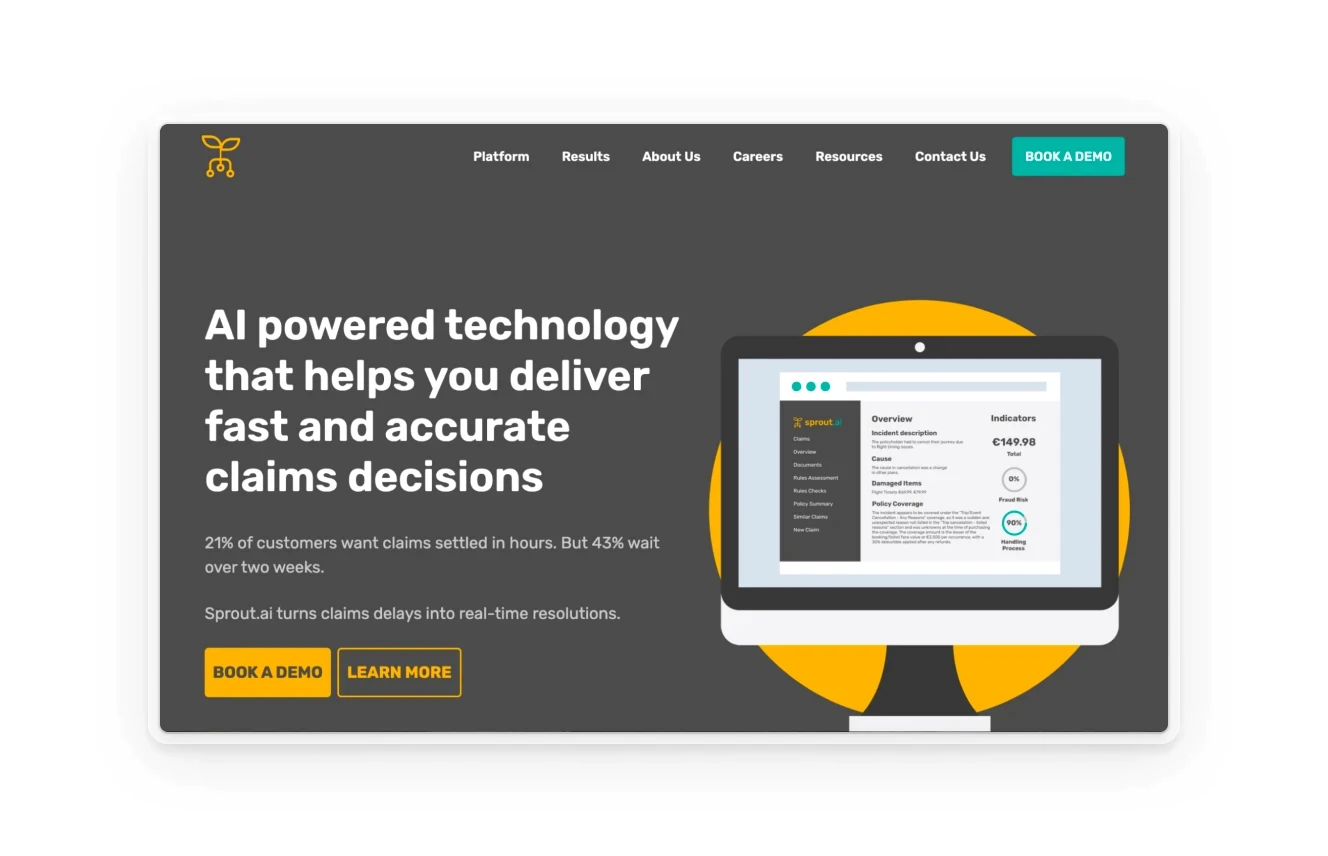
Since its founding in 2018 (initially as Blockclaim Ltd), Sprout.ai has focused on revolutionizing claims processing through AI. The London-based company's platform stands out for its ability to reduce claims settlement times from weeks to seconds, supported by robust multilingual capabilities that include complex languages like Japanese.
Following an £8 million Series A funding round led by Octopus Ventures in 2021, Sprout.ai has expanded its feature set to include advanced fraud detection and real-time processing capabilities. The platform's seamless integration with existing Claims Management Systems helps insurers modernize their operations without disrupting existing workflows.
Beam AI: streamlining operational workflows
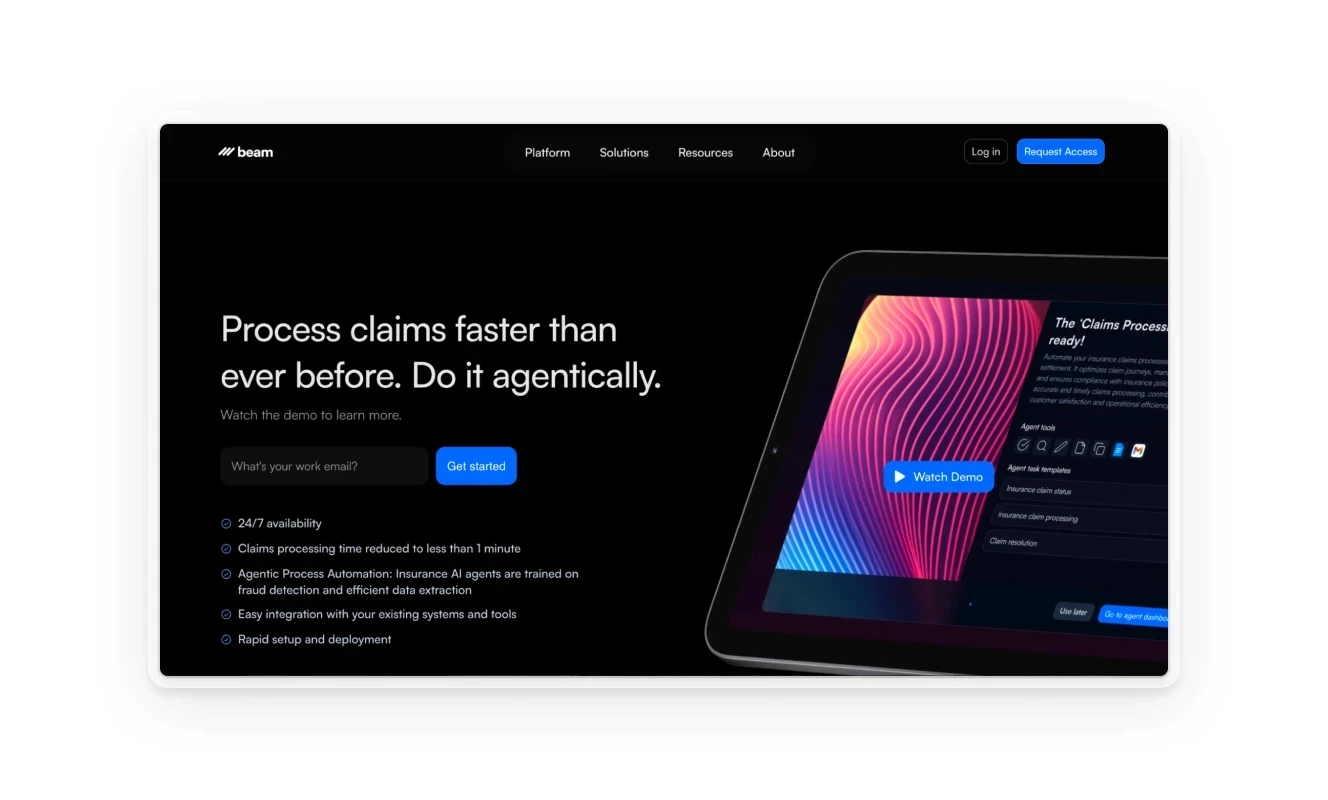
Beam AI has positioned itself as a comprehensive solution for insurance workflow automation. Their platform specializes in creating AI agents that handle specific insurance tasks, from First Notice of Loss (FNOL) processing to customer service interactions.
With pricing starting at $499 monthly for handling 2,000 orders, Beam AI offers a structured approach to automation. Their custom AI agent setups, beginning at $10,000, allow insurers to tailor solutions to their specific needs. The platform's strength lies in its ability to manage end-to-end insurance processes, from initial customer contact to claims resolution.
Each of these platforms brings unique capabilities to the insurance industry, addressing different aspects of the underwriting and claims process. Still, having powerful tools doesn't guarantee successful implementation.
AI and insurance: best practices and adoption challenges
OK, we’ve discussed mind-blowing adoption rates and amazing use cases that are already being implemented.
But—
Here’s the catch: piloting AI tools does not equal full-scale implementation or meaningful ROI. Many of these projects remain stuck in pilot purgatory, plagued by integration issues, regulatory hurdles, and resistance from seasoned underwriters who—rightly—don’t trust black-box systems.
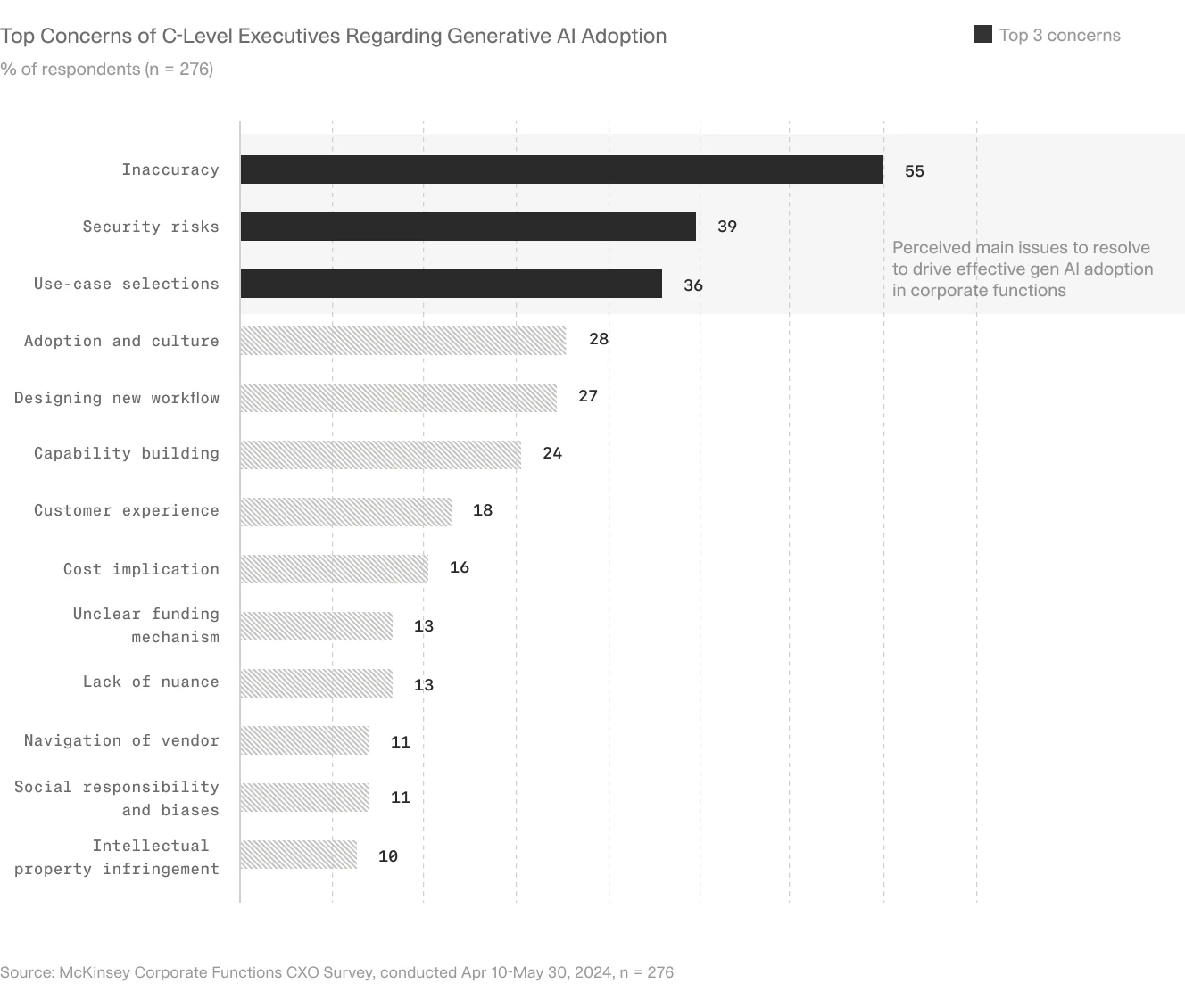
Many insurers find themselves stuck in pilot purgatory, with promising tests that never scale to production. According to McKinsey’s 2024 report on generative AI in corporate functions, the reality is far more nuanced than the hype suggests:
Adoption ≠ Effectiveness: While 22% of corporate functions have rolled out generative AI for at least one use case, this indicates that a significant majority are still in the experimental phase, with full-scale deployment remaining elusive.
The Human Factor: Inaccuracy (55%) and security risks (39%) are the top concerns cited by CXOs, followed by challenges in selecting appropriate use cases (36%). These issues undermine confidence in generative AI’s ability to deliver consistent results.
Actual ROI: Even among organizations pursuing enterprise-wide strategies, only 35% have successfully deployed at least one generative AI use case, underscoring the difficulty of translating investment into impact.
It’s one thing to invest in AI tools, it’s another to translate those investments into substantial improvements in underwriting or claims processing.
Success in implementing AI for insurance underwriting requires careful attention to both technical and organizational factors. While technology forms the foundation, the human element ultimately determines whether an AI implementation thrives or falters.
Here's a practical guide to get started and scale effectively.
Step 1. Planning and assessment
Begin by evaluating your current underwriting process. Map out how long it takes to process different types of submissions, where bottlenecks occur, and which tasks consume most of your underwriters' time. This baseline measurement will help you track improvements.
Start small by selecting a single, straightforward use case. Submission ingestion often proves ideal because it's contained and delivers measurable results quickly. Gather a core team including an experienced underwriter, a technology specialist, and a project manager who understands both domains.
Step 2. Pilot implementation
Choose an AI platform that matches your specific needs. Consider starting with tools that offer pre-built templates for common insurance workflows. V7 Go, for instance, provides a free tier that lets you test document processing capabilities without significant investment.
Select a single line of business for your pilot. Commercial property insurance often works well because it involves standardized documents like Market Reform Contracts and Schedules of Values. Run your AI system alongside existing manual processes, carefully documenting any discrepancies or issues that arise.
Step 3. Refinement and optimization
Use this period to fine-tune your implementation based on real-world cases. Pay special attention to accuracy rates and processing times. Work closely with your underwriters to adjust document classification rules and optimize integration points with existing systems. Prompt engineering is another area which requires special attention and can significantly impact the quality of your results.
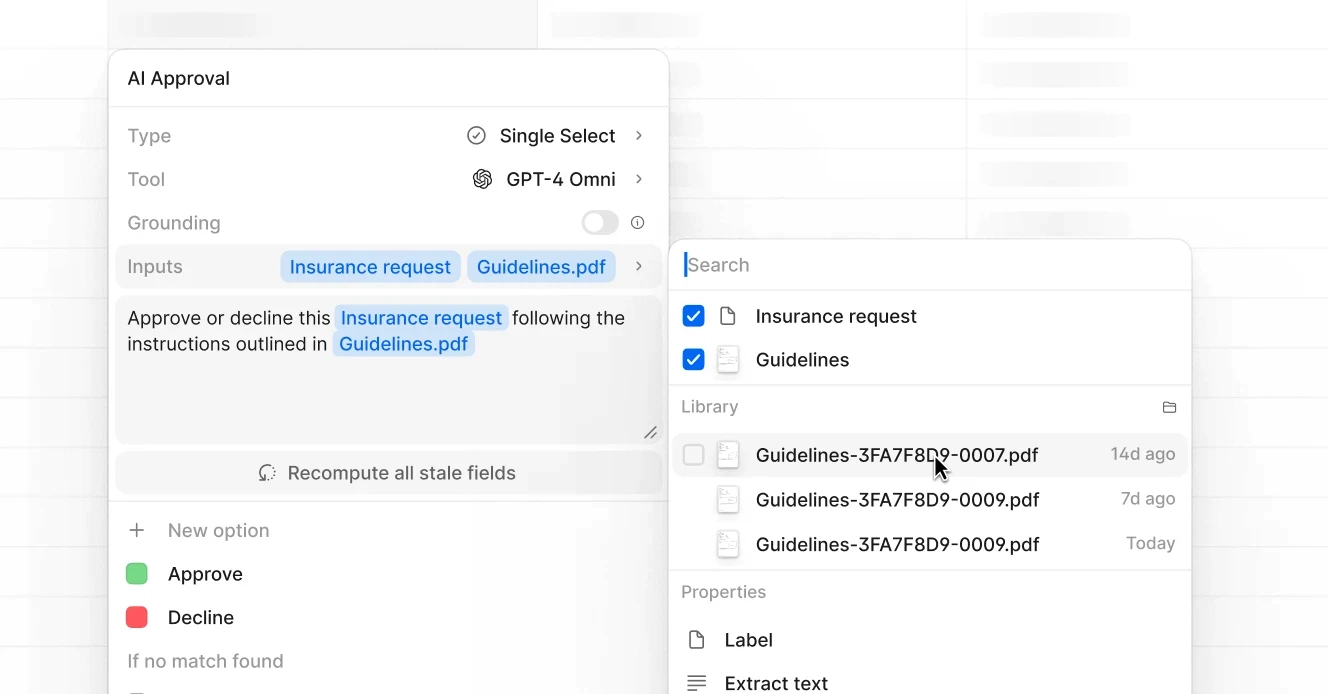
Create clear protocols for handling edge cases where AI requires human intervention. Document these procedures thoroughly – they'll become your playbook as you scale. Remember that the goal isn't to eliminate human oversight but to enhance it.
Step 4. Training and integration
Now's the time to focus on training your team. Start with hands-on sessions where underwriters can see how the AI system processes real submissions. Show them how to interpret AI-generated insights and when to override automated decisions.
Integrate your AI system with existing underwriting platforms gradually. Begin with simple data transfers before moving to more complex integrations. This approach helps identify and resolve technical issues before they affect your entire operation.
Step 5. Scaling and expansion
With a proven process in place, begin expanding to additional lines of business. Start with those most similar to your pilot program – this lets you leverage existing workflows while managing risk. Monitor key metrics like processing time, error rates, and underwriter satisfaction throughout the expansion.
Use the insights gained from your initial implementation to refine your approach. What worked well in commercial property might need adjustment for liability coverage. Stay flexible and keep gathering feedback from your underwriting team.
Remember that successful AI implementation in insurance isn't about replacing underwriter judgment – it's about enhancing it. When done right, AI handles routine tasks while freeing your experts to focus on complex risk assessment and client relationships.
The key to success lies in maintaining this balance between automation and expertise. Start small, prove value quickly, and scale thoughtfully. Your underwriters are your greatest assets in this transformation – involve them early and often in the process.
Critical considerations for long-term success
AI implementation requires careful attention to both technical and organizational readiness. Organizations must assess their data quality, infrastructure capabilities, and team skills before beginning. One regional insurer spent three months organizing and preprocessing their historical data before starting their AI implementation – an investment that paid dividends in system accuracy.
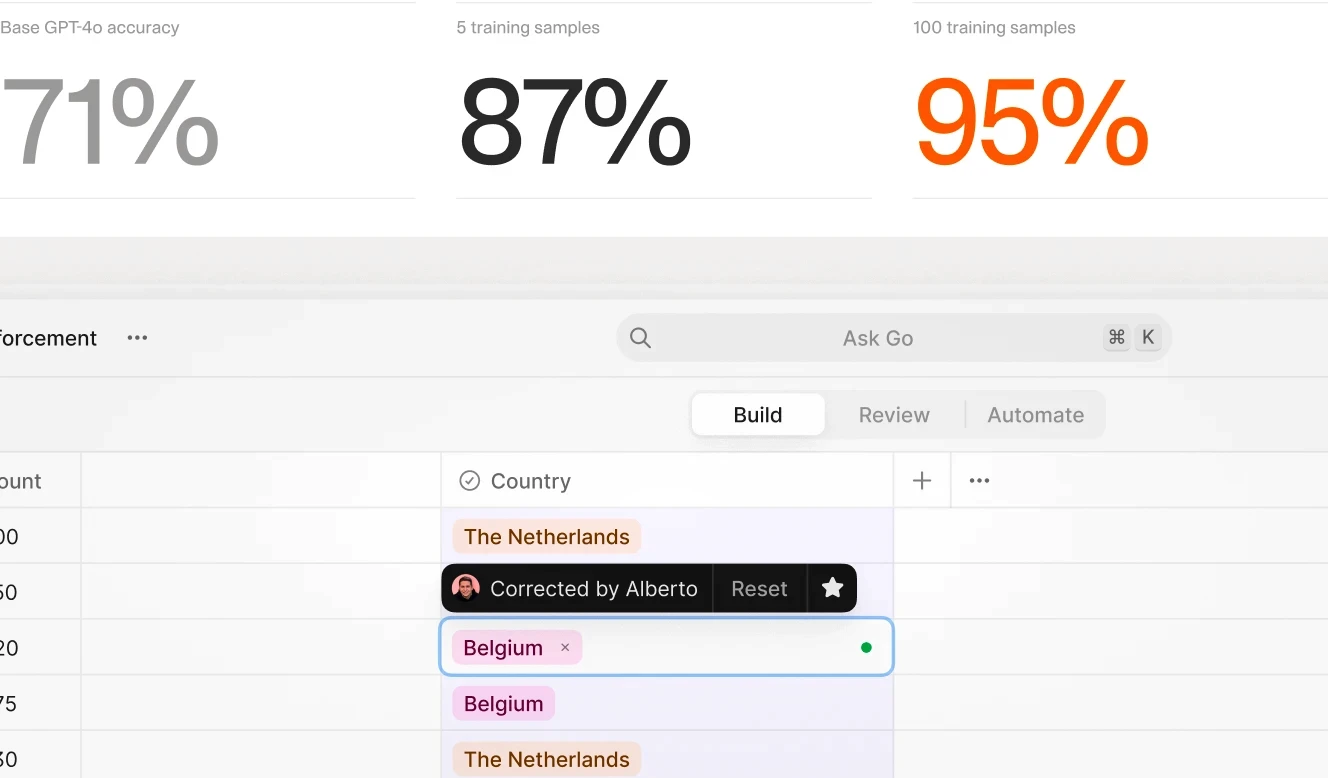
Accuracy rates demand ongoing monitoring and refinement. Leading organizations establish clear thresholds for acceptable AI performance and processes for handling cases where confidence scores fall below these thresholds. Regular audits of AI decisions help maintain quality while identifying areas for system improvement.
Privacy and security concerns require constant attention as AI systems process sensitive customer and business information. Organizations must implement robust data governance frameworks and regular security audits to protect against breaches and ensure compliance with evolving regulations.
Looking ahead: what is the future of underwriting?
The integration of AI in insurance underwriting represents more than a technological shift – it marks a fundamental evolution in how we approach risk assessment and policy pricing. As these systems become more sophisticated, the role of underwriters will continue to evolve toward higher-value activities that require human judgment and expertise.
Success in this transformation requires maintaining a delicate balance between automation and human oversight. Organizations that thoughtfully integrate AI while preserving the crucial role of underwriter expertise will find themselves best positioned to thrive in an increasingly competitive insurance landscape.
The future belongs not to those who simply deploy AI, but to those who harness it thoughtfully to enhance rather than replace human capabilities. In this light, today's implementation decisions lay the groundwork for tomorrow's competitive advantage.
Ready to give AI a shot?
Explore how platforms like V7 Go empower underwriters to focus on what matters most.