LLMs
14 min read
—
Apr 14, 2025
Multi-agent AI turns complex workflows into manageable tasks handled by specialized agents. Learn how this approach improves automation in real-world processes.
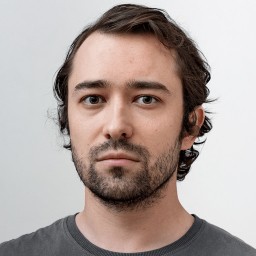
Content Creator
When ChatGPT first appeared, businesses saw great promise. At last—an AI capable of using human language and following your requests.
But anyone who has tried using ChatGPT for actual work quickly runs into frustration and disappointment. Ask it about a random encyclopedic fact or tell it to write a generic message, and it works like magic. Try to rely on it for serious work that requires reasoning or multi-step thinking, and it falls apart.
Why is that so?
Current AI tools demonstrate impressive performance on isolated tasks but struggle with the demands of real-world business processes. A financial analyst using ChatGPT to review quarterly reports soon finds the model loses track of earlier details, fails to maintain consistency across documents, and can’t connect to your existing business tools.
These shortcomings stem from foundational design constraints: fixed context windows, an inability to break down complex tasks into smaller components, and a lack of integrations or specialization across different types of work. As a result, when AI is applied to workflows like contract processing, financial analysis, or multi-source research, it delivers inconsistent results and demands constant human oversight.
This gap has brought a key insight into focus: tackling complexity requires collaboration, even for AI. The solution lies in a system powered by an army of AI agents and tools working toward a shared goal, rather than a single model.
In this article, we’ll explore:
What multi-agent AI is and how it works
The benefits and shortcomings of agentic AI
Real-life examples of multi-agent AI solutions
Best practices for implementation in business
Instead of relying on a single, general-purpose model, multi-agent AI systems divide complex tasks among specialized agents, each responsible for a specific function, and coordinate their efforts through a central orchestrator. It’s a model inspired by how organizations operate: not by expecting one person to do everything, but by enabling teams of specialists to work together.
What makes multi-agent AI different
Before diving into multi-agent solutions, it’s helpful to clarify what we mean by AI agents and agentic AI. An AI agent is essentially a large language model (LLM) that can break down a task into smaller subtasks, access external tools, and make decisions about what to do next.
By contrast, standard LLMs take a single input and return a single output. While that’s immensely useful, LLMs on their own cannot take multiple actions on behalf of their users. With some LLM-based assistants, you might be able to trigger a web search or an image generation task, but the interaction ends there. These systems lack the ability to coordinate multiple steps in pursuit of a broader objective.

A defining feature of AI agents is their ability to self-prompt—that is, to generate follow-up prompts for themselves based on intermediate results, enabling them to perform multi-step reasoning or workflows autonomously. This allows a single agent to recursively call itself, trigger additional LLM queries, and coordinate actions across tools like Google Drive, email inboxes, Python environments, or third-party APIs and webhooks. Unlike static, one-off interactions with a closed system like ChatGPT, AI agents operate dynamically, chaining actions and adapting their behavior to achieve a specific goal.
A multi-agent system consists of multiple AI agents designed to interact within a shared environment to achieve specified goals. Unlike a standalone LLM or a single AI agent, multi-agent systems are able to break down complex problems into subtasks and distribute them across specialized AIs, each handling specific aspects of a larger task and designed with unique challenges in mind.

This architecture includes specialized processing agents, communication protocols that define how agents exchange information, and an orchestration layer that manages workflow and task assignment. Some systems also incorporate shared memory mechanisms and integration capabilities with external business tools.
The distributed nature fundamentally changes how AI tackles complex problems. For example, when analyzing a lengthy contract, a multi-agent system might employ data extraction software to digitize the document, a parsing agent to identify clauses, a comparison agent to check against standard terms, and a summarization agent to compile findings. The orchestrator—in V7 Go we call this feature “AI Concierge”—manages these handoffs and maintains global context.

This approach differs significantly from asking a single model to analyze the entire contract at once. Instead of processing everything sequentially within one context window, multi-agent systems break tasks into discrete steps handled by specialized components, reducing complexity at each stage while maintaining coherence across the entire process.
Benefits of multi-agent systems in business
Popular AI tools face several practical constraints in business settings. Their fixed context windows limit the amount of information they can process simultaneously. Even advanced models with 100,000 token limits cannot fully analyze lengthy financial reports or legal documents in a single pass. When context exceeds these boundaries, earlier information gets discarded, leading to inconsistent analysis and forgotten details.
Additionally, the internal "black box" nature of AI reasoning processes makes error identification difficult. When a single model produces an incorrect conclusion, pinpointing exactly where the reasoning went wrong is nearly impossible, complicating debugging and quality control.
Multi-agent systems offer a meaningful improvement here. Because each agent is assigned a discrete, well-defined task—extracting data, validating figures, applying business rules, generating summaries—it becomes much easier to trace the flow of logic and isolate where failures occur. If the final output contains an error, you can inspect each agent’s step in the chain to determine whether the problem originated in data extraction, validation, interpretation, or synthesis. This modularity turns opaque reasoning into a series of transparent, auditable checkpoints.
In practical terms, this means issues can be diagnosed and fixed faster. Instead of fine-tuning an entire monolithic model or guessing which part of a giant prompt failed, you can focus on the specific agent or logic block responsible for the error. This also enables teams to apply targeted improvements—retraining one agent, inserting a human-in-the-loop check at a known failure point, or adjusting a decision policy—without disturbing the entire system.

A system built around a single LLM model cannot easily adapt or scale specific components based on changing requirements, creating an all-or-nothing approach to capabilities.
Multi-agent systems address these limitations through specialized processing and coordinated workflows. By assigning specific tasks to dedicated agents, the system ensures each component handles only what it's designed for. Document classification, data extraction, analysis, and summarization become distinct functions performed by specialized agents, potentially using different underlying models optimized for each task.

The modular design creates natural fault tolerance. If one agent encounters difficulties with a specific task, the system can flag the issue, attempt alternative approaches, or escalate to human review while allowing other components to continue their work.
In many multi-agent AI solutions, like in V7 Go, each agent produces discrete, traceable outputs that can be logged and reviewed. This creates clear visibility into how conclusions were reached. The transparency addresses critical requirements in regulated industries where decision provenance matters for compliance and audit purposes.

The distributed approach effectively handles information volumes exceeding any single model's capacity. Agents maintain specialized memory or distill information into summaries passed to subsequent agents, managing context across extended processes. This particularly benefits legal discovery and regulatory compliance workflows where document volumes regularly exceed single-model capabilities.
The orchestration layer enables adaptive coordination based on intermediate results. If document analysis reveals unexpected content, the system can modify the process flow or activate specialized agents for deeper investigation rather than following a rigid sequence.
Multi-agent AI in practice across key industries
Multi-agent AI systems have been deployed across knowledge-intensive industries with notable success in handling complex document workflows.

Legal departments use these systems to automate contract review processes. Document processing agents handle intake of agreements, classification agents identify clause categories, comparison agents check against standard templates, risk assessment agents evaluate liability exposure, and summary agents produce analysis reports. Most law firms can reduce contract review time by 60% while increasing risk identification accuracy.
Financial services firms implement multi-agent systems to analyze investment documents and reports, for example with agents specialized in AI financial statement analysis.
Different agents extract numerical data, process textual information, identify trends, and generate visualizations, with all insights compiled into comprehensive reports. The parallel processing allows analysts to cover more companies and respond faster to market events.
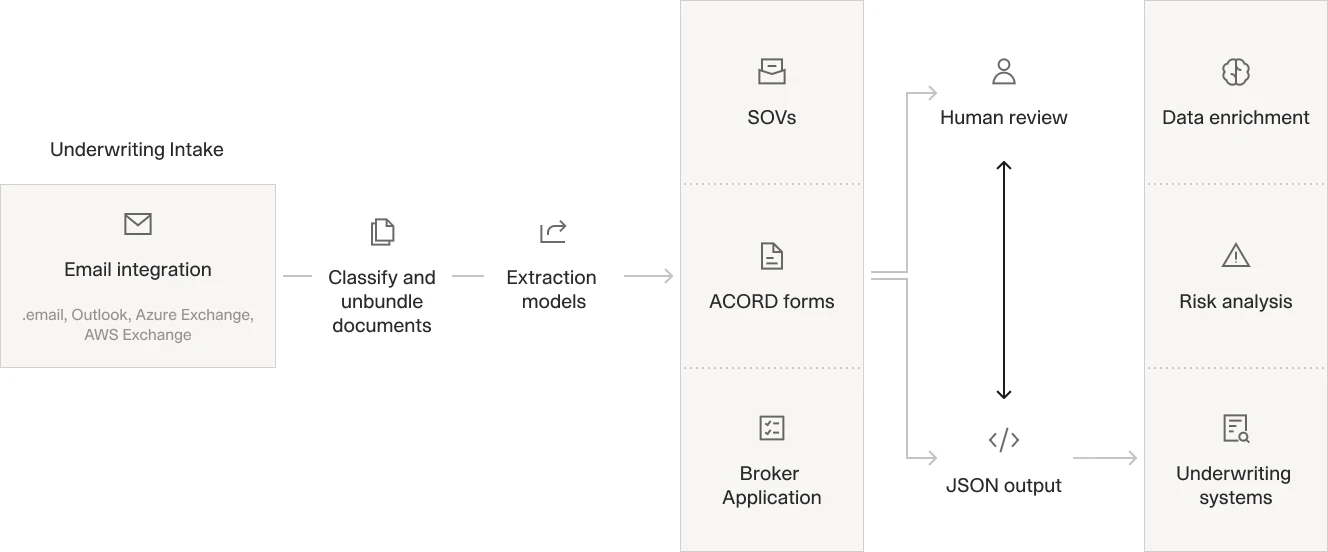
Insurance companies employ multi-agent frameworks for automated claims processing workflows. Document digitization and OCR agents handle intake forms, data extraction agents collect relevant claim information, policy verification agents check coverage details, fraud detection agents identify suspicious patterns, and adjudication agents calculate appropriate payments.
Commercial real estate operations benefit from multi-agent systems for AI lease management. Document processing agents extract terms from lease agreements, calculation agents determine payment schedules, compliance agents verify regulatory requirements, and notification agents generate alerts for upcoming deadlines or renewal options. Property management firms managing hundreds of properties report significant reductions in administrative overhead through these automated workflows.
V7 Go’s AI Concierge is a good example of the multi-agent AI approach. It enables businesses to design multi-agent workflows through a user-friendly interface. The platform allows users to create custom AI workflows powered by LLMs and multimodal AI, where each step represents a specialized agent function.
For example, a financial document analysis workflow might include:
An OCR agent converting scanned documents to machine-readable text
A data extraction agent identifying key metrics and performance indicators
A web research agent retrieving online performance data and industry benchmarks
A comparison agent using Python libraries and analyzing current performance against forecasts
A summary agent generating executive reports with actionable findings
The AI Concierge can orchestrate these workflows and determine which agents to activate based on user requests. It can manage information flow between agents and present unified results. The orchestration happens behind the scenes—users simply upload documents and submit questions, and the multi-agent system handles the complexity of coordinating the appropriate tools.
V7 Go includes pre-built agents for common industry tasks that let businesses leverage established workflows for lease abstraction, claims processing, financial analysis, and legal document review without building them from scratch. These pre-built agents incorporate domain expertise and best practices, accelerating implementation for specific industries.
Learn more: What Are the Latest Applications of Artificial Intelligence?
Implementing multi-agent AI systems
Organizations implementing multi-agent AI systems must address several key design and development considerations. Successful implementation starts with thorough process analysis and task decomposition.
Rather than attempting to automate entire workflows at once, teams should identify specific components suitable for agent handling.
For example, a loan approval process might include document verification, credit analysis, risk assessment, regulatory compliance checks, and decision compilation. Financial institutions report that starting with detailed process mapping helps ensure the system design reflects actual business requirements rather than theoretical frameworks. This approach identifies natural boundaries between tasks and highlights dependencies that must be maintained during automation.

Each agent requires clear specifications for inputs, outputs, responsibilities, and performance metrics. You can also give them access to specific tools or systems. The most effective designs create narrowly focused agents handling specific functions rather than attempting to build versatile agents operating across the entire app ecosystem. This specialization simplifies development, testing, and improvement of individual system parts.
Conditional branching accommodates decision points where the next step depends on intermediate results. Event-driven architectures enable more dynamic workflows where agents activate based on specific triggers. Hierarchical systems use meta-agents to coordinate specialized sub-agents for particularly complex processes. The orchestration layer maintains global context and manages overall process flow, making it a critical component for reliable operation.

Several technical frameworks support multi-agent system development. Code-first platforms like LangChain and LangGraph provide libraries for creating agent workflows and managing inter-agent communication. AutoGen offers tools specifically designed for agent coordination and information exchange. V7 Go provides a no-code platform for designing and deploying multi-agent workflows using visual tools and a chat interface. Organizations typically select platforms based on their technical capabilities, implementation timeline, and integration requirements.
Integration with existing business systems provides much of the value from multi-agent AI. Key integration points include document management systems providing input materials, enterprise databases supplying reference information, communication tools for notifications, and analytics platforms for monitoring performance. Successful implementations feature bidirectional integration allowing agents to both access and update business systems, creating closed operational loops.

Governance frameworks have also become essential as multi-agent systems handle sensitive business data. Leading implementations establish access controls determining which agents can view specific information, comprehensive logging of all agent actions, regular security audits, and clear data handling policies. Financial and healthcare organizations often implement additional monitoring agents specifically tasked with verifying compliance across the entire workflow.
Learn more:
Challenges and solutions
Organizations implementing multi-agent AI typically encounter several challenges requiring thoughtful approaches. System complexity increases with each additional agent, complicating design, testing, and maintenance. Technology leaders report difficulty diagnosing issues in systems with many interconnected agents. Successful implementations typically start with minimal viable workflows using 3-5 agents before expanding gradually as teams develop expertise.
Operating multiple AI models increases computational costs compared to single-model approaches, especially with local, on-premises deployment based on models such as Llama or Mistral. Organizations must balance performance benefits against resource requirements. Some teams implement tiered approaches where intensive models handle critical tasks while lighter-weight models manage routine functions to control overall costs.
The orchestration layer introduces potential failure points beyond the agents themselves. Rigorous testing of coordination logic before deployment has proven essential for reliable operation. Identifying the responsible component when errors occur requires comprehensive monitoring and diagnostics. That’s why organizations are developing specialized tools to visualize workflow execution and pinpoint failure points within complex agent networks.

Maintaining consistent knowledge representation across different agents remains challenging, particularly when agents employ different underlying technologies. Standardized data schemas and explicit verification steps between agent handoffs help ensure information remains accurate throughout complex workflows. In many cases, vector databases and retrieval augmented generation (RAG) are becoming important auxiliary tools that allow integrating off-the-shelf AI agents with internal knowledge and processes.
Perhaps the most significant challenge involves building organizational trust in multi-agent systems. Technical teams must explain not just what the system does but how it makes decisions through component agents. Documentation showing agent roles, responsibilities, and interaction patterns has proven effective in building confidence among business users.
Learn more:
What Are the Latest Applications of Artificial Intelligence?
The Rise of Work AI: Will Knowledge Work Be Fully Automated?
The future of multi-agent AI in business
Multi-agent AI systems will likely evolve toward greater autonomy and deeper business integration. Future systems will monitor business environments and proactively identify opportunities for action rather than waiting for explicit instructions. Financial compliance systems might continuously check new regulations against existing policies, alerting relevant departments to potential gaps without specific queries.
Agent compositions will become more dynamic, with orchestrators assembling appropriate teams based on specific task requirements rather than following fixed workflows. This mirrors how human organizations form project teams with appropriate skills for particular challenges.
These systems will also increasingly become embedded infrastructure components rather than standalone applications. They will continuously process information flows, make routine decisions, and handle exceptions according to established policies. This transition from tool to infrastructure follows the pattern of earlier technologies like databases and networks that became foundational business elements.
As standards emerge, agent interactions may extend beyond organizational boundaries. Supply chain partners might share compatible agent systems that communicate across company lines according to established protocols while maintaining appropriate security boundaries, enabling seamless coordination while preserving organizational autonomy.
The most promising direction involves evolving relationships between human workers and multi-agent systems. Rather than simple automation that replaces human tasks, emerging approaches position multi-agent systems as collaborative partners that handle routine aspects while escalating exceptions and novel situations to human colleagues. This partnership model preserves human judgment for critical decisions while eliminating repetitive elements of knowledge work.
If you're exploring how multi-agent systems could streamline your workflows, our solution engineers are here to help. Book a demo and share a sample of your data, and we’ll work with you to build a working proof-of-concept using V7 Go’s AI Concierge. Whether you're starting from scratch or looking to scale, we’ll guide you through the next steps—from mapping your processes to deploying agents with real business impact. Let’s turn your documents into decisions, faster.