Knowledge work automation
9 min read
—
Apr 10, 2025
Are financial analysts about to be replaced by AI? What does the rise of AI mean for different levels of finance roles? And most importantly—how can finance professionals stay ahead?
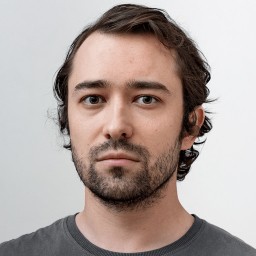
Content Creator
The finance industry stands at a crossroads. Recent research revealed that GPT4 achieved 60% accuracy in predicting earnings changes, outperforming human analysts who averaged 53%.
What makes this study particularly striking is that artificial intelligence (AI) accomplished this using only the raw numbers from balance sheets and income statements. It didn't have access to management discussions, industry context, or any of the qualitative information that analysts typically rely on. Even more remarkably, the AI's performance was on par with sophisticated machine learning models specifically trained for earnings prediction tasks.
This finding has intensified an already heated debate: will AI replace financial analysts?
The answer requires understanding not just what AI—and generative AI in particular—can do, but how it fits into the broader landscape of financial analysis.
In this article:
The role of AI in transforming financial analysis
Why entry-level analysts face the biggest disruption
Where human expertise remains irreplaceable in financial analysis
The future of analyst careers
The Reality of Automation in Finance
Traditional machine learning systems have long been used for pattern recognition and quantitative analysis, making them valuable tools for tasks like risk assessment and market analysis. These solutions can process vast amounts of historical data to identify trends and make predictions, particularly when working with structured numerical data.
But, the fundamental challenge in financial analysis isn't just about interpreting numbers. It's about getting those numbers into a usable format in the first place. A single company analysis might involve pulling data from annual reports, earnings call transcripts, industry reports, and market data. Each source typically comes in its own format, requiring manual effort to extract and standardize the information. For instance, when analyzing a Confidential Information Memorandum (CIM), analysts often spend hours copying figures from PDFs into structured Excel templates, checking for accuracy, and reformatting data to match internal standards.

For financial analysts, the reality of day-to-day work often involves hours spent on data extraction and organization—tedious but crucial tasks that form the foundation of financial analysis.
An average day of financial data analysis varies depending on the role and company, but generally involves a mix of data extraction, cleaning, modeling, and reporting. A significant portion of time—often 60-70%—is spent writing SQL queries, working with Python or other tools to clean and manipulate data, and developing models or dashboards. Tasks might include reformatting raw data, performing anomaly detection, or implementing machine learning models for classification and prediction.
Many analysts and data scientists prefer working on reusable assets like dashboards or automated reports rather than one-off analyses or presentations. Some enjoy dashboarding, using tools like Oracle BI or Tableau, while others dislike tasks that require manual updates in Excel or PowerPoint. Writing clear explanations for stakeholders is an essential skill, as even automated reports often require detailed documentation.
Meetings typically take up 10-30% of the day, whether for daily stand-ups, brainstorming solutions, or presenting insights. Some roles involve frequent context-switching due to ad hoc requests, while others have dedicated deep work time. More senior professionals may spend additional time mentoring junior colleagues, reviewing code, or discussing architectural decisions.
While the field is rewarding, common frustrations include under-documented data sources, tight deadlines, and non-technical stakeholders who require frequent explanations. Many professionals find joy in coding, problem-solving, and automation but dread repetitive manual tasks.
And, this happens to be precisely where the true impact of the latest AI models, particularly large language models (LLMs), becomes apparent.
Unlike traditional automation tools that work only with structured data, LLMs can process unstructured information—like the text in a quarterly report or a CIM—and convert it into structured formats ready for analysis. AI platforms powered by LLMs can now perform complex financial analysis in ways that mirror human reasoning. They aren’t just about crunching numbers. These models generate useful narrative insights about company performance and demonstrate an ability to reason about financial data in ways previously thought to require human judgment.

While end-to-end automation of financial analysis is technically feasible, most LLM applications in finance remain in the piloting phase. The most promising applications focus on the crucial steps before contextual analysis and final evaluation. The workflow is remarkably straightforward: using an AI platform like V7 Go, analysts can first upload various financial reports without any preformatting. The AI handles documents regardless of their structure or format.
The next step involves uploading internal guidelines and methodologies, essentially teaching the AI your company's specific analytical approach. This can be done securely, without sharing proprietary information with third-party providers, as these systems can be implemented within closed environments. The AI then becomes an extension of your company's analytical framework.
Once configured, the AI agent can extract not just key financial metrics but also relevant contextual information. Through data enrichment powered by AI search engines, it can gather information beyond basic financials—details about a company's customers, partners, and market position. The system can also cross-reference current data against your historical database, providing deeper contextual analysis.
The final step transforms all this information into structured data formats like JSON, which can be seamlessly integrated with your existing tools. Your master Excel spreadsheet can automatically populate with the extracted and generated data, or the information can flow directly into visualization tools like Tableau. This creates a smooth pipeline from raw documents to analysis-ready data, eliminating hours of manual processing while maintaining analytical rigor.
So what does this mean for different types of financial analysts? The impact varies dramatically across different roles and levels of seniority, fundamentally reshaping how financial analysis work gets done.
Entry-Level Analysis: Most Vulnerable to Change
The reality of entry-level financial analysis involves spending 70-80% of time on data processing tasks. At major investment banks, first-year analysts dedicate most of their time to gathering and processing data. A typical mergers and acquisitions (M&A) analysis requires downloading financial statements from multiple years, extracting hundreds of data points, standardizing them across different accounting standards and currencies, and organizing everything into proprietary templates. This process alone can consume 2-3 days of work before any actual analysis begins.
These data-heavy tasks are precisely where AI can bring significant value. AI tools for private equity and venture capital firms for processing CIM documents can reduce data extraction time from days to under an hour while achieving higher accuracy than human analysts. Similarly, investment banks are adopting AI to manage the initial processing of quarterly earnings reports, thereby freeing junior analysts to focus on other analytical tasks that have yet to be automated.
While these developments enhance efficiency from a business perspective, they also raise concerns about the future of entry-level roles. The automation of foundational tasks traditionally performed by junior analysts could lead to a reduction in the number of these positions.
Moreover, as AI takes over routine work, entry-level professionals may find fewer opportunities to develop essential skills through hands-on experience. This shift could disrupt traditional career progression paths, making it challenging for aspiring financial analysts to gain the experience necessary for more senior roles.
So, where does this leave the next generation of financial professionals?
In response to these changes, it's crucial for entry-level professionals to adapt by developing skills that complement AI capabilities. This includes focusing on analytical thinking, strategic decision-making, and proficiency in AI and data analysis tools. Financial institutions can support this transition by providing training programs that emphasize these competencies.
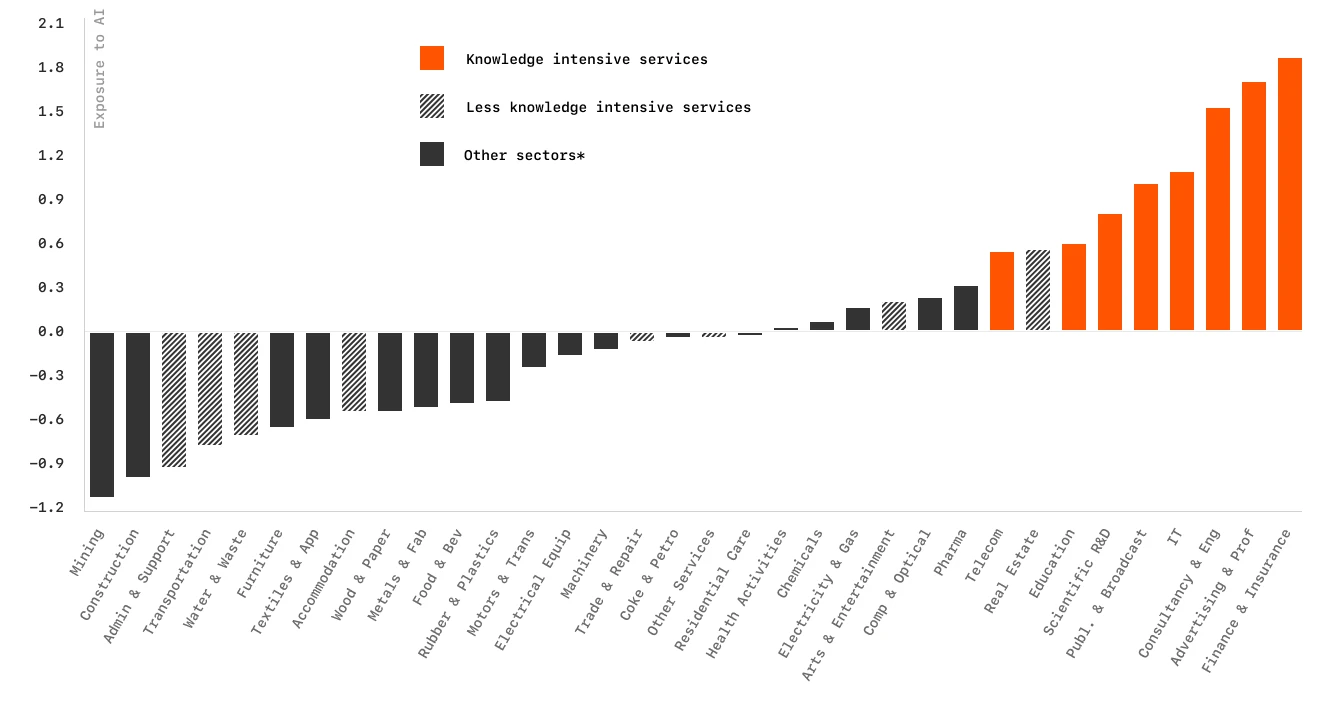
Notably, the financial and insurance sectors are already among the most exposed to AI, integrating and piloting these tools at a rapid pace. As AI adoption continues to accelerate, professionals who proactively build these complementary skills will be best positioned to thrive.
Learn more: 10 Key Use Cases of Generative AI in Finance
Middle-Office Functions: A Shift in Focus
The story becomes more nuanced when we look at mid-level analysts. Both AI and human analysts can bring unique insights to the table. Mid-level analysts aren't being replaced. Instead, they're evolving into what the industry calls "hybrid professionals." These analysts combine traditional financial acumen with technological sophistication, creating a new breed of professional that's more valuable than either pure human analysis or pure AI automation.
This transformation is particularly evident in how mid-level analysts approach their work. Rather than spending hours on data processing and initial analysis, they're now focusing on strategic interpretation and business partnering. Many analysts are learning programming languages like Python, not because they're becoming programmers, but because understanding these tools allows them to work more effectively with AI systems.
Learn more: An Introduction to Financial Statement Analysis With AI
Senior Analysts: A New Strategic Role
While AI is proving highly effective at automating repetitive tasks, like AI document processing, senior financial analysts remain indispensable. Their roles are evolving into more strategic positions, focusing on high-value tasks that require deep expertise, market intuition, and nuanced decision-making.
Rather than spending time on spreadsheet-heavy analysis, senior analysts are leveraging AI-generated insights to guide investment decisions and shape financial strategies. AI provides a powerful tool for extracting and organizing data, but senior analysts apply their judgment to interpret the numbers in a broader business and economic context. For instance, while AI can flag anomalies in financial reports, human analysts determine whether those anomalies are significant enough to impact investment decisions.
This integration of AI and human expertise is further reinforced by human-in-the-loop solutions, where analysts validate and refine AI-generated outputs. Reinforcement learning from human feedback (RLHF) ensures that AI continues to improve over time. This is essential for streamlining complex financial scenarios that require contextual understanding and nuanced decision-making.
Additionally, AI is enhancing the way senior analysts work with clients. Instead of merely presenting raw financial data, they can now use AI-driven tools to provide clients with real-time, tailored insights based on large datasets. This shift enables them to take on more of a consultative role, positioning them as trusted advisors rather than data processors.
Learn more: How AI Can Finally Fix Investor Reporting and Investor Relations
AI as a Key Enabler in Finance Functions
AI is not just transforming financial analysis but also revolutionizing core finance processes. According to research from the IBM Institute for Business Value, finance executives who integrate AI into their operations report measurable efficiency gains. AI is being incorporated into various financial workflows, including:
Record-to-Report (R2R): AI-powered reconciliation modules aggregate subledger transactions and perform risk-based reconciliations, improving reporting accuracy.
Procure-to-Pay (P2P): AI-driven automation has increased productivity and enhanced fraud detection in invoice processing.
Order-to-Cash (O2C): AI facilitates credit scoring, pricing decisions, and payment fraud prevention.
Financial Planning and Analysis (FP&A): AI and advanced analytics optimize financial planning, performance management, and forecasting.
AI’s ability to handle repetitive and time-consuming tasks has led to efficiency improvements in financial analysis. For example, many financial consulting firms have reported productivity gains after automating due diligence processes.
One such firm, Centerline, implemented AI to extract and analyze financial data, reducing manual workload and increasing efficiency by 35% in early trials. However, this automation also raises concerns about potential skill erosion among entry-level professionals, as fewer opportunities for hands-on learning could impact their long-term career growth.
For now, the accuracy of AI outputs depends on multiple factors and human oversight remains essential to validate results. Analysts must be equipped to critically assess AI-generated insights rather than relying on automation as an unquestioned authority.
The Future of Financial Analysis
While some junior roles may decline in number, financial analysis is not becoming obsolete. Instead, AI is creating new opportunities by redefining how work is done.
Companies are investing in hybrid roles that require financial expertise alongside AI fluency. Analysts who can bridge the gap between traditional financial analysis and emerging AI technologies will be in high demand.
For financial professionals, this means:
Developing AI literacy: Understanding how AI models work and how they can be leveraged in financial analysis.
Strengthening analytical and strategic skills: AI can process data, but it cannot replace human intuition, experience, or the ability to synthesize complex information into actionable strategies.
Orchestrating AI workflows: Just as a conductor must thoroughly understand a symphony to lead an orchestra, financial professionals must deeply understand financial processes to effectively integrate AI. Building a good AI automation workflow requires a well-informed human to ensure alignment with business goals as well as technological and financial realities.
Enhancing communication and advisory skills: With AI handling much of the data processing, financial analysts will need to focus more on interpreting results and communicating them effectively to stakeholders.
While AI is undoubtedly changing the finance industry, it is not eliminating the need for financial analysts. Instead, it is redefining their role, shifting focus from routine data processing to complex, value-driven tasks. The ability to collaborate with AI effectively will be a crucial skill, allowing professionals to focus on interpreting and applying insights rather than performing repetitive manual tasks.

As companies continue to explore AI-driven financial workflows, maintaining transparency about its strengths and limitations will be essential. By understanding both the capabilities and constraints of AI, financial professionals can better position themselves to leverage these tools effectively. Those who embrace this evolution and develop complementary skills will be well-positioned to navigate the future of financial analysis, ensuring their expertise remains relevant and valuable in an AI-augmented industry.
For those looking to integrate AI into their financial workflows, exploring the right tools is a critical step. If you are interested in learning more about AI tools for financial analysis and how they can enhance your company's decision-making processes, book a demo to see these solutions in action.