Document processing
22 min read
—
Apr 22, 2025
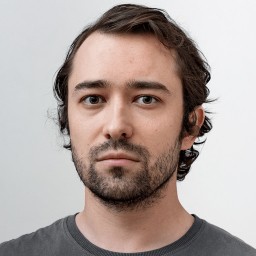
Content Creator
The due diligence phase of mergers and acquisitions (M&A) is notorious for its intensive document review, high costs, and tight deadlines. In fact, legal and advisory fees for due diligence can reach up to 10% of the total deal value, reflecting the enormous effort required to comb through financial statements, contracts, and disclosures.
Virtual data rooms (VDRs) have become the standard for managing this avalanche of information, replacing physical data rooms with secure online repositories. Now, a new shift is underway: artificial intelligence is being applied to VDR analysis, promising faster insights and fewer missed red flags. Early adopters of AI for due diligence are already reporting significant efficiency gains.
In this article:
What virtual data rooms are in an M&A context
The benefits of AI-enhanced VDRs (and their limitations)
How AI data room platforms actually work
Leading VDR software options
By the end, you’ll understand both the potential and the practical considerations of analyzing VDRs with AI.
Let’s dive in.
What are Virtual Data Rooms?
A virtual data room is a secure digital repository where deal-related documents are stored, organized, and shared for due diligence. Think of it as a highly secure cloud vault that replaces the old locked filing room. VDRs allow multiple authorized parties—buyers, sellers, attorneys, bankers—to review confidential documents simultaneously from anywhere, with fine-grained access controls to protect sensitive information.
During an M&A process, after initial interest is confirmed and NDAs are signed, the seller populates a VDR with all the company’s critical documents. These often include:
Confidential Information Memorandum (CIM)—a detailed overview of the business
Financial statements, audit reports, and cap tables
Major contracts (customer contracts, supplier agreements, leases)
Legal documents (corporate charters, licenses, litigation history)
HR records (organizational charts, key employee agreements)
Technical documents (IP filings, product roadmaps).
In later stages, additional documents like letters of intent (LOIs) from bidders, and eventually the draft purchase agreement, may also reside in the data room for all sides to evaluate.

A well-structured VDR for due diligence can contain thousands of pages spanning everything from tax returns to board meeting minutes.
How does a VDR function in practice?
At its core, the VDR is a web-based application provided by a third-party vendor that specializes in secure document hosting. The seller’s team (or their investment bank advisors) uploads files into a predefined folder structure—often organized by categories like Financial, Legal, Operations, Tax, IT, HR, etc.
An AI data room solution like V7 Go enables users to create custom views that bring together multiple interfaces into a unified workspace:
The file management system lets you filter and organize documents
The spreadsheet-style view presents document status and extracted insights at scale
The chat interface allows users to ask open-ended questions about various aspects of the deal. The AI assistant uses retrieval-augmented generation (RAG) to locate relevant information, explain its reasoning, and link each answer directly to the source documents.

Permissions are then set so that each user or group sees only the folders and projects relevant to them (for example, bidders might not see each other’s information, and certain sensitive files might only be visible to lead bidders or specific advisors). Users log in via a secure portal, often with optional multi-factor authentication, and can view or download documents according to their permissions. Crucially, every action in a VDR is logged—the system tracks who accessed which document and when, providing an audit trail for compliance and insight.
Traditional VDRs vs AI VDRs
Originally, VDRs were essentially static repositories—highly secure, but just a better-organized file server. Users still had to manually read through documents and perform analysis outside the system. The documents were typically in PDF, Word, or Excel format. If data was not standardized (and it often isn’t—one file might be an audited financial PDF, another might be a scanned contract image, another an Excel model), the onus was on the human analysts to piece together insights.
Today’s AI data room platforms can ingest unstructured documents and make sense of them, without requiring strict templates or manual data entry. For instance, large language models (LLMs)—a type of AI trained on vast amounts of text—can read through a 200-page legal contract and answer questions about it, or summarize its key provisions, something that used to require a junior lawyer’s full attention. They can also cross-reference information across documents. Imagine an AI noticing that the sales figures mentioned in the CEO’s presentation don’t match the numbers in the financial statements, and flagging this discrepancy automatically.
Such cross-document analysis was practically impossible to do at scale manually, but AI agents can now sift through the entire corpus of data room files to find patterns, inconsistencies, and answers to specific queries.
Instead of flipping through hundreds of files, an analyst might ask an AI tool, “Show me all liabilities over $100k mentioned anywhere,” or “Summarize the target company’s EBITDA and revenue by year from 2018–2022,” and get immediate answers sourced from the relevant documents.
To illustrate, consider these examples:
CIM triage
A Confidential Information Memorandum can be 50-100 pages of dense detail about a company. Traditionally, an associate might spend half a day skimming it to pinpoint the key financial metrics, growth drivers, and risk factors. An AI system can analyze a CIM in seconds, pulling out structured data (like revenue, EBITDA, customer concentration) and generating a bulleted summary of the company’s market position and challenges.

The human deal team gets a quick brief, and can decide where to dig deeper—effectively triaging the opportunity faster. If something looks off (say the AI finds an anomaly in margins year-over-year), the team knows to investigate that specifically. This kind of triage does not replace reading the CIM entirely, but it dramatically focuses human attention where it matters.
Financial due diligence
In a data room, you might have the last three years of audited financials, this year’s year-to-date financials, and a financial model or forecast. An AI tool can automatically extract the figures from the PDF statements (using OCR to read scanned documents if necessary) and cross-verify them with the numbers in the Excel model.
If the revenue figure in 2024’s audited P&L doesn’t match the 2024 actual in the model spreadsheet, that discrepancy can be flagged immediately as a potential issue (could be a modeling error or an update that wasn’t captured). AI can also calculate key ratios and trends—for example, generating a quick time series of EBITDA margin or inventory turnover from the data—saving the analyst the trouble of manual data entry.

Additionally, AI anomaly detection might highlight, say, an unusual spike in expenses in one quarter that merits explanation. These are the kinds of “red flags” that AI is adept at catching across large data sets, allowing the deal team to prioritize their investigative efforts.
Data extraction, computer vision, and OCR
It’s important to note that documents in VDRs often come in unstructured formats, and AI tools have advanced to handle that. Unstructured means the info isn’t in neat rows and columns; instead it’s buried in paragraphs or complex tables. Many AI platforms use a combination of Optical Character Recognition (OCR) software to digitize text from scans, and Natural Language Processing (NLP) via LLMs to interpret the meaning. On top of that, computer vision models are used behind the scenes to understand the layout and structure of complex charts and tables.

In practical terms, whether the data room contains a clean Excel sheet or a photo of a hand-signed contract, AI can pull out the text and numbers and make them searchable and analyzable.
By turning a heap of disparate files into something more like a queryable database, AI changes the role of the VDR from a passive archive to an active due diligence assistant. Instead of just hosting documents, the VDR becomes an intelligent system that helps make sense of those documents. Still, AI won’t replace financial analysts or lawyers, but it can help them focus on judgment calls by handling the tedious, time-consuming work of extracting, sorting, and summarizing information.
Benefits of using virtual data room Solutions
Virtual data rooms by themselves (even without AI) brought numerous benefits over old-school methods of sharing deal documents, such as email or physical binders. These core benefits include security, accessibility, and efficiency. By centralizing all deal information in one secure online hub, VDRs ensure that sensitive information remains confidential—robust encryption and access controls mean only authorized users see what they’re meant to.
Every user action is tracked, creating accountability and a clear audit trail in case of disputes or compliance checks. VDRs also enable faster deal progress because multiple parties can review documents in parallel 24/7, instead of scheduling sequential visits to a physical data room or waiting on couriers. This global accessibility can be critical in cross-border deals.
Moreover, VDR software often provides convenient features like full-text search (to instantly find keywords in documents), indexing and table of contents generation, and Q&A modules that let bidders ask questions about documents within the platform. Compared to emailing files back and forth—which raises version control issues and security risks—a VDR keeps everything organized and up-to-date in real time. In short, VDRs improve confidentiality, transparency, and deal velocity in M&A due diligence
Now, when you layer AI capabilities on top of those existing benefits, the value proposition becomes even more compelling. AI augmentation addresses the biggest bottleneck in due diligence: human analysis time.
Here are some major benefits of using AI in conjunction with VDRs:
Increased document understanding
One of the immediate wins is using AI to summarize lengthy documents. Many VDR providers now integrate AI summarization tools that can produce an executive summary of a document’s content at the click of a button.
This is incredibly useful for legal counsel who might otherwise spend hours reviewing a SPA (Sale and Purchase Agreement) or for a buyer scanning a thick technical specification document. Some AI tools, like V7 Go, even offer AI citations and they highlight the exact sentences and numbers in the source document that support each point in the summary. This traceability is critical for trust: an analyst can quickly verify the AI’s interpretation against the original text.
Improved risk detection
AI can serve as an extra set of eyes, pinpointing risk factors or anomalies that warrant attention. This goes beyond keyword search, as AI can now understand context too. For example, an AI contract review tool might flag clauses such as indemnities, termination rights, or non-standard representations in contracts as potentially risky.
Instead of manually comparing hundreds of contracts to find which have unusual terms, the AI can do that heavy lifting. One can think of it as a sophisticated issue checklist running in the background. Similarly, AI can flag financial red flags like unusual revenue movements, margin outliers, or inconsistencies between different reports. While even some of the latest AI applications like ChatGPT or Claude are not fully equipped with the expertise or tools to perform tasks such as full AI financial statement analysis, a combination of LLMs working together with specialized Python libraries, third-party financial tools, and dedicated AI models can automate 95% of the process.
Advanced market insights
One innovative use of AI in M&A is the ability to compare the target company’s data against external benchmarks, public information, or the investor’s own portfolio data. For instance, a private equity firm often wants to know how a potential acquisition’s metrics stack up against industry peers or companies it already owns. AI can make such comparisons nearly instant.
By feeding the AI not just the VDR content but also external data (market data, industry reports, or internal portfolio performance data), the AI can generate context. It can also find relevant documents and information online via content scraping or AI web search engines.

AI essentially acts as an analyst that has read not only the target’s data room but also a library of market data and can cross-reference the two. This was rarely feasible in the past due to time constraints—you’d have separate teams doing market studies—but AI can bring those threads together in real time.
The challenges and limitations of AI for VDR

While AI promises significant advantages in VDR analysis, several important challenges and limitations must be considered:
Accuracy and hallucination concerns
Large language models are known to occasionally "hallucinate" information—generating plausible-sounding but incorrect content. In high-stakes M&A transactions, even small inaccuracies can have significant consequences. This is why leading VDR platforms implement strict AI guardrails, including:
Source citations for all AI-generated insights
Confidence scoring on AI analysis
Human-in-the-loop verification for critical findings

To increase accuracy, it’s important to understand that LLMs are “language” models, and their grasp of math is, in many ways, incidental. That’s why it’s crucial to implement solutions that clearly distinguish between numeric and text formats, as well as between calculations performed by the AI and those executed via Python code or Excel formulas. In the image above, you can see a V7 Go property that is explicitly set up as a numeric output.
Implementation challenges
Implementing AI solutions in a VDR context isn’t plug-and-play. It often requires integration and possibly the help of AI solution engineers or consultants who can fine-tune the tools to your specific document types and objectives. Financial data and legal language can be very domain-specific. For instance, an off-the-shelf LLM might not understand a “convertible note cap table” or the nuances of a “force majeure clause” without some training. Many firms find they need to customize models or at least use advanced prompt engineering techniques to get reliable results.
Regulatory and compliance considerations
Different jurisdictions have varying regulations regarding data privacy, AI use, and financial disclosure. Companies must ensure their AI data room solutions comply with relevant regulations like GDPR in Europe or industry-specific requirements. Financial institutions, in particular, may face additional scrutiny when using AI for due diligence.
Despite these challenges, we are already seeing real-world adoption. Several private equity firms and investment banks have piloted AI data room due diligence. For example, Investcorp, a global PE firm, recently piloted an AI platform for VDR analysis, and found that it streamlined their review process enough that they’re expanding its use.
Learn more: Best Applications of AI in Venture Capital and Private Equity
How to use AI virtual data rooms: Practical guide
AI-enabled virtual data rooms bring together a set of advanced technologies to automate and augment the analysis of documents. Understanding the key components can help demystify what’s going on when you “ask an AI” about your data room. The major building blocks include OCR, intelligent document processing, data extraction, large language models, retrieval augmented generation, and AI search, often orchestrated in an agent-like workflow. Let’s break down each and then see how they integrate in a typical workflow.
Optical Character Recognition. Much of due diligence content is trapped in PDFs or even scanned images (think of a signed contract PDF—it’s essentially a picture of text). OCR is the technology that converts images of text into actual text data. It’s a foundational step: AI can’t analyze what it can’t read.
Intelligent Document Processing. This technology goes a step further to understand the structure and elements of a document. IDP might involve classifying a document type (is this a financial statement or a contract?), identifying sections, headings, tables, and figures, and extracting key fields. Essentially, IDP bridges unstructured and structured data—turning unstructured documents into structured outputs.
Large Language Models. At the heart of many of these AI features, especially anything involving understanding language or generating summaries, are large language models. These are AI models (like GPT-4o, etc.) trained on massive text corpora that can understand and generate human-like text. In a VDR context, an LLM serves as the “brain” that reads through documents and can answer questions or produce narratives.
Retrieval Augmented Generation. RAG is a framework that combines LLMs with a focused search of a specific document set. This is often implemented by using vector embeddings—each document (or document chunk) is encoded into a numerical vector in a database, and the question is encoded to find similar vectors (i.e., find which document sections are most related to the query).
AI Agents. Agentic AI tools can autonomously perform tasks, often by breaking down a complex job into subtasks and calling appropriate tools, which might be other models or programs. In the context of an AI VDR, you can imagine your main AI assistant as a multi-agent AI system that orchestrates multiple specialized AI agents to thoroughly analyze the data room.
In practical terms, many generative AI tools for finance appear on the surface to be simple chatbot assistants, but they perform complex operations behind the scenes—processing uploaded documents and responding to requests with synthesized results. The AI agent functions more like a project manager overseeing a due diligence review: it breaks down the task (“Analyze this company’s financials”) into smaller steps—extracting tables, classifying documents, computing ratios, checking compliance—and delegates each one to the appropriate tool.

As a result, your AI VDR doesn’t just “read” documents—it understands, processes, and synthesizes them across multiple layers of analysis and reasoning by triggering the right automations.
On top of the technologies mentioned above, integration with existing tech stack is also of critical importance. AI VDRs don’t work in isolation. They often integrate with other software via APIs. This allows them to pull in data from or push results to other tools. For example, a due diligence AI might connect to a CRM system like Salesforce to cross-check customer names in the VDR against known customers. Or it could integrate with an accounting system to fetch the latest actuals for comparison with the numbers provided in the data room. On the sell-side, an AI might connect to a company’s SharePoint or Google Drive and automatically ingest new documents into the VDR.
Here is an example of how an AI virtual data room workflow might function in practice:
Step 1: Document upload
The seller uploads documents into the VDR. AI reads file names and samples content to group documents and create top-level tags like “Financial”, “Legal”, “Commercial”, “HR” etc., and files are sorted accordingly. It can also populate documents with metadata: e.g., mark five PDFs as “Financial Statements” based on content and file name patterns, and identify some spreadsheets as “Financial Model” and “Budget”. This automated organization means you don’t have to dig for the key documents—they’re neatly sorted.
Step 2: Data extraction
This process is triggered right after the upload. OCR kicks in so that now every document in the VDR is text-searchable. Additionally, the knowledge base is indexed for RAG and data extraction. From the financial statements, it pulls the income, balance sheet, cash flow line items into a structured table. It does the same for interim monthly financials provided. From cap table documents, it extracts the list of shareholders and ownership percentages. From each customer contract, it extracts fields like customer name, contract value, start/end dates, renewal terms, termination provisions. Essentially, it’s creating a mini database of key deal data: financial metrics, key contracts, key assets and liabilities, etc. This might take a few minutes to process all documents.
Step 3: AI agent analysis
Depending on the nature of the task, one or more specialized agents can be triggered. In some cases, you can design an intelligent workflow that routes documents to different agents or automations based on initial classification. For example, legal documents might automatically go to an agent tailored for contract analysis, while financial documents are sent to another focused on financial review. The number of reasoning steps can be configured to match the complexity of the task, but the end result is a structured summary compiled by the final AI response and presented in a custom dashboard.

Step 4: User Interaction via Q&A
Once the documents are fully processed and analyzed, users can ask additional questions and interact with the VDR through a natural language interface. This allows deal team members—whether legal, financial, or commercial—to ask questions like “Can you list all customers with annual contract values above $100K?” The AI will understand the underlying structure and reference the indexed knowledge base, extracted data, and structured outputs to return accurate, context-aware answers. This turns the VDR into an interactive, intelligent assistant—saving hours of manual review and surfacing insights that might otherwise be missed.
Best virtual data room software
The market for virtual data rooms is well-established, with many providers now incorporating AI to differing degrees. Below we evaluate some key platforms: V7 Go, FirmRoom, Datasite, Intralinks, iDeals, and SecureDocs, focusing on their strengths, especially in AI workflows.
V7 Go
A newer entrant that comes from the AI world rather than the traditional VDR space. V7 Go is essentially an AI work automation platform that has been applied to portfolio data room analysis and due diligence. V7 Go’s strength is in its AI-first approach—it is built to handle unstructured documents with large language models, computer vision, and an agent-based architecture.

V7 Go is used by some venture capital and private equity firms specifically to automate CIM reviews and portfolio data room analyses, showing its focus on the buy-side need for speed. For a financial professional, using V7 Go might feel like having an AI co-pilot deeply integrated in the data room—it can answer questions, find metrics, and trigger custom workflows using internal or external tools.
If you’d like to learn more about the platform and see if it fits your data room needs, you can book a demo. In most cases, V7 Go’s solution engineers can deliver a working prototype of your document workflow within just a few days.
FirmRoom
FirmRoom is a more traditional virtual data room platform that spun out of the DealRoom M&A software suite. It’s known for being cost-effective, user-friendly, and focused on mid-market M&A transactions. FirmRoom’s key strengths are in its simplicity and performance: it has an intuitive interface with drag-and-drop uploads, automatic indexing, and very fast full-text search across documents. In terms of AI, FirmRoom itself (as a standalone VDR) doesn’t advertise as many AI-specific features as some competitors—it focuses on getting the basics extremely right: secure sharing, permission control, and Q&A.
Datasite
Datasite is one of the premier VDR providers globally. It is a go-to platform for large deals, used by investment banks, PE firms, and corporates alike. Datasite has invested heavily in AI and machine learning features, branding their suite as Datasite Intelligence. One of its hallmark AI features is automated redaction—Datasite can automatically find and redact sensitive information (like personally identifiable info or specific keywords) across thousands of pages, which is a huge time-saver when preparing documents for sharing.
Intralinks
Another heavyweight in the VDR arena, historically one of the first widely used VDRs for M&A. It is known for rock-solid security and a rich feature set for deal management. In recent years, Intralinks has also infused AI into its platform. Intralinks offers real-time analytics and reporting on user behavior (e.g., who is spending time on what pages), which while not AI in the analytic sense, is a useful intelligence feature for sell-siders to gauge buyer interest. On the collaboration front, it has advanced Q&A workflows, vital for managing bidder questions during diligence.
iDeals
A popular VDR provider that positions itself as a secure and user-friendly solution for a broad range of use-cases (M&A, fundraising, board communications, etc.). Over the past few years, iDeals has gained a strong reputation, often appearing in “best data room” lists, thanks to its balance of features and cost. iDeals has embraced AI in features such as smart search, content indexing, and OCR.
SecureDocs
This platform has gained popularity among startups, small to mid-size companies, and for applications like fundraising due diligence or smaller M&A deals. Its hallmark is in the name—security with extreme simplicity. SecureDocs focuses on the core features without a lot of superfluous extras, and offers one of the fastest setup times: you can set up a data room in 10 minutes. It’s basically ready out-of-the-box, which is great when time is critical. The interface is straightforward, and admins can easily drag and drop bulk files, set permissions, and invite users quickly.
Each virtual data room platform tends to cater to a specific use case—some, like V7 Go, are optimized for AI analysis, others prioritize ease of use for M&A workflows, while some focus on advanced security, enterprise features, or quick, cost-effective deployments. The good news is that the market is highly competitive, driving rapid innovation—particularly in AI capabilities—and more flexible pricing models. When evaluating options, consider the complexity of your deal, the level of AI functionality you need, and your priorities around budget, user experience, and support.
AI and the VDR: What’s Next?
Today's AI-enhanced VDRs no longer function as passive storage systems but as intelligent assistants that read, categorize, and extract meaning from unstructured data. Investment banks and private equity firms at the forefront of this revolution report dramatic efficiency gains. This acceleration doesn't come at the expense of thoroughness—quite the opposite. AI systems are good at spotting patterns, inconsistencies, and anomalies that human reviewers might miss when facing tight deadlines and information overload.
The most effective implementations pair AI's processing power with human expertise. While the technology can summarize contracts, extract financial metrics across years of statements, and answer complex queries by synthesizing information from multiple documents, it cannot replace the nuanced judgment of experienced deal professionals. The AI presents information and preliminary insights; humans interpret those findings in context and make strategic decisions.
Looking ahead to the next two to five years, we can expect several developments to further reshape due diligence practices. Routine document review will become almost entirely automated, with specialized AI agents handling specific domains like financial analysis, legal compliance, and operational assessment. These systems will continuously improve as they process more deals, leading to higher-quality insights and fewer post-closing surprises.
The role of deal teams will evolve accordingly. Analysts and associates will spend less time on mechanical document summarization and more on interpretation, strategy, and asking the right questions. New hybrid roles will emerge, combining financial acumen with technical expertise in configuring and optimizing AI systems for specific transaction types.
We'll also see greater integration between VDR platforms and the broader deal ecosystem. AI assistants will follow transactions from sourcing through execution and into post-merger integration, creating a continuous intelligence thread. This seamless flow of insights will make institutional knowledge more accessible and actionable throughout the deal lifecycle. As these capabilities become standardized, even smaller advisory firms will gain access to tools previously available only to large institutions. The competitive playing field may level somewhat, though larger organizations will likely develop proprietary enhancements to maintain their edge.
Book a demo today to see how AI can elevate your due diligence process from document review to insight generation.