AI implementation
How to Secure the Best ROI from Your AI Investment in 2024
17 min read
—
Mar 1, 2024
How can you ensure your AI has an ROI? We break down the best practice mechanisms for securing value from your AI products.
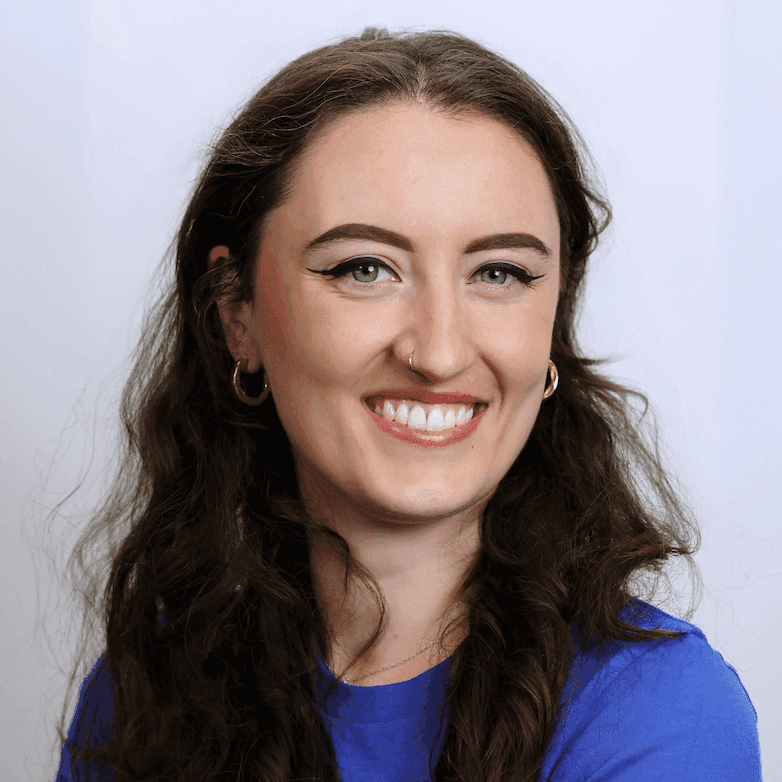
Senior Content Marketing Manager
Securing a tangible return on investment from artificial intelligence is a challenge, with the average ROI on enterprise-wide AI initiatives sitting at just 5.9%. Despite this, spending on AI (spanning software, hardware, systems, and services) is set to hit $200 billion in 2024.
Businesses worldwide are using AI to secure faster diagnoses for patients, create AI-driven co-pilots for internal teams, and even build intelligent chatbots designed to increase customer satisfaction.
However, skeptics and CFOs, quite rightly, are beginning to ask - is investment in AI a sunk cost? A premature embrace of the technology? Or simply a mismanaged opportunity that needs some tweaking?
While the average ROI of an enterprise project sits at 5.9%, research from IBM reveals that certain companies earn a more notable 13% ROI on their AI projects.
So, what’s differentiating these companies?
In this article, we tackle exactly that, from the habits of high ROI AI projects to understanding how you can squeeze value from your AI spend.
We'll cover:
Investing in AI: The Strategic Imperative
How to strategize AI ROI
Define your objectives
Address your resources
Cost estimations
Cost-saving opportunities in AI
Predicting ROI
ROI example: Buying training data operations software versus building it
How to calculate AI ROI
Long term versus short-term ROI
Maximizing AI ROI
Extra resources for managing AI spend
Investing in AI: Why it’s strategically imperative
AI experienced phenomenal growth in 2023, exemplified by a litany of valuations that have reawakened the venture capital market. This includes a $4bn valuation for Hugging Face, $29 billion for OpenAI, $750 million for Pinecone, and a monumental $820 billion for hardware giant, Nvidia.
While the ROI of AI proves difficult - it hasn’t shaken the faith of technologists, entrepreneurs, and investors in AI’s potential.
So - why is investing in AI a strategic imperative? In many respects, that question warrants an article of its own, but at a top level:
First movers’ advantage
The companies that will most benefit from AI are already figuring out how best to implement and leverage the technology. This goes beyond investment into software, hardware, and services, but also into the holistic approach a business needs to adopt AI successfully. This includes grappling with ethical concerns, embedded security safeguards, and educating teams on how and when to use AI. These companies have a distinct advantage over the companies “waiting for the dust to settle”, thanks to foundations paved today, that will accelerate their growth tomorrow.
Automation
AI automation can take over monotonous tasks, reduce the financial losses that come with “busy work”, and streamline processes to ensure staff only work on valuable projects. Strategically, this makes AI a powerful tool that can reshape the working environment of modern companies; impacting hiring, task delegation, and the pace of cost-effective growth a business can achieve. For more on this, dive into our article on the impact of LLMs, spanning customer service automation, AI competitor analysis, and autonomous agents.
Customer satisfaction
AI is a particularly powerful tool in the pursuit of customer satisfaction. For example, AI can be used to create personalized responses or solutions for a customer, it can provide responsive chatbots, and it can be used to continuously nurture customers as they move through their buying cycle. These benefits are evidenced in the 2023 AI in Customer Experience report, which reveals customers are now actively seeking out AI that can guide them through a product, offer personalized deals, and make product recommendations.
Competition
AI is allowing companies to offer products that are not only innovative by design but also competitive in their time-to-market and ability to scale. Similarly - when systems are set up to ensure an AI is continuously updated - pivoting products to consumer demands becomes an intuitive part of the process. With the early adoption of AI, businesses are staying ahead of the curve - both in how they use cutting-edge technology, and how quickly they can release it to the market. Many of these businesses can expect a hefty financial reward - with McKinsey research revealing that AI could increase corporate profits by $4.4 trillion a year.
The best-performing AI products are continuously updated, with systems in place that can quickly correct, tweak, and pivot the model’s output. To discover how to do the same, dive into our article on the secret of successful AI products.
How to strategize AI ROI
Profitable enterprise initiatives follow a series of best practices that park hype in favor of strategy. So, without further ado, let’s start with the basics.
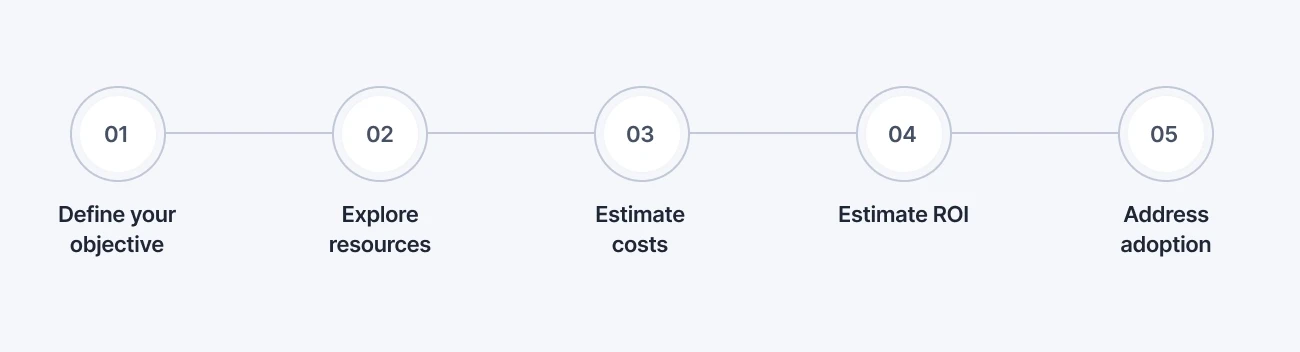
Define your objectives
While experimentation sits at the heart of innovation, it's important that your AI solves an actual problem. One of the most common reasons for product failure - and therefore, collapsing ROI - is poor product market fit. While now is certainly the time to embrace AI, it’s a technology, not a magic wand.
AI requires investment, spanning AI infrastructure costs right through to the morale of the people working on the project. By defining your objective early on - and ensuring it maps closely with your business strategy, you have a far higher chance of securing early-stage ROI, which can then be used to get the green light on more experimental projects.
AI Solutions with a Good Market Fit:
Healthcare Diagnostics: Enhances diagnostic accuracy and speed by analyzing medical imagery.
Security: Provides real-time detection of security threats and malicious activities.
Document Processing: Automates the extraction and classification of information from documents.
Manufacturing: Optimizes manufacturing processes and predicts maintenance needs.
Inventory Management in Retail: Improves real-time tracking and predictive analytics for inventory management.
Agricultural Monitoring: Aids in the timely detection of crop health issues and pest activities.
Autonomous Vehicles: Interprets sensor data for real-time driving decisions in autonomous vehicles.
AI Solutions with a Challenging Market Fit:
Emotion Recognition: Faces accuracy challenges and ethical concerns across different demographics.
Virtual Beings: Limited practical utility and monetization challenges outside entertainment.
AI Image Creation: Struggles to gain market value and appreciation compared to human-created artworks or photographs.
Real-time Crowd Analysis: Encounters privacy concerns and regulatory hurdles.
Virtual Try-On: Faces accuracy challenges in product representation and integration.
Fully Autonomous Customer Service: Lacks human understanding in handling complex or sensitive situations.
Take the time to interrogate your use case: What would be the impact of solving this problem? Why is AI the solution to this problem? How does this align with your existing business strategy?
On this, training data operations consultant Kevin Chang writes,
“Always start with the need, before the AI. AI is a cool piece of technology and a lot can be learned by simply trying it out, but it’s always better to apply AI to a problem you have right now. How many resources you put towards an AI project ultimately depends on the return on investment from those resources. Sometimes, that return on investment will just be knowledge and research and understanding - but it should always be for an explicit return.”
Address your resources
Time and time again, the businesses that secure reliable ROI from AI investments are those that work hard to invest in, and mature, their AI capabilities. This ranges from a well-considered tech stack that seamlessly integrates with existing systems, right through to hiring top machine learning talent - and providing training to standardize the development process. To get started, it helps to ask - and answer - a couple of questions.
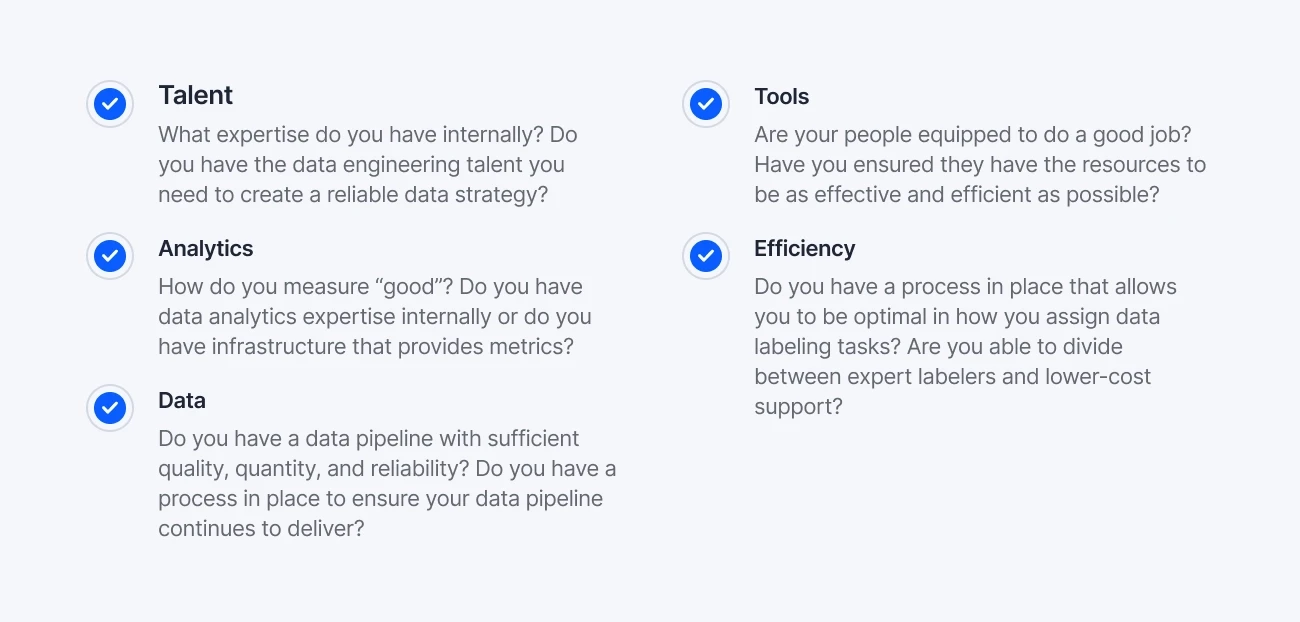
Answering the above will help you address:
The scope of talent you’ll need (and how to maximize the talent you already have).
How you’ll go about measuring the metrics of your development, (and therefore its likelihood of success).
How you’ll source, manage, and structure your data pipeline (a key driver of your AI’s success).
How to prioritize the tooling you need to invest in, versus the tools you can repurpose internally.
How to streamline your development.
Next, you’ll need to take a closer look at your AI infrastructure, which will span data collection, data management, data processing, and data outputs. While you can opt to build your own solution, there are many out-of-the-box solutions that manage much of the development lifecycle, while integrating with third-party apps, platforms, and cloud-service vendors.
Businesses like V7 provide training data operations software that simplifies core components of the process, including data labeling, model training, dataset management, and customizable workflows.
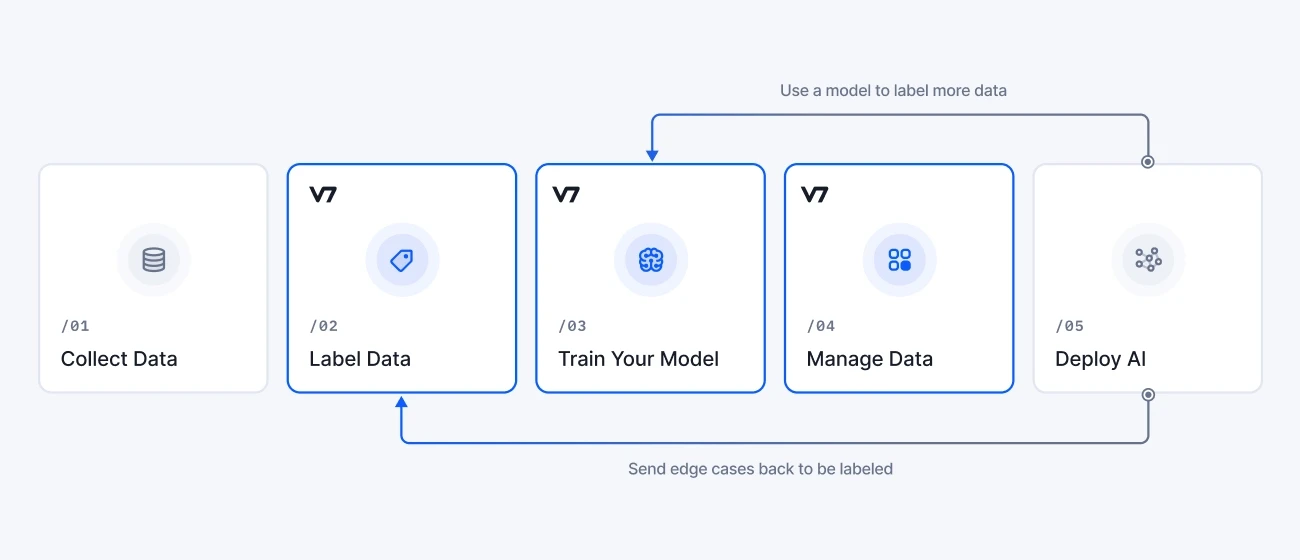
These platforms successfully reduce the total cost of ownership for AI projects, while boosting the productivity of ML teams.
When it comes to your tech stack, you’ll need to:
Address how to reduce the total cost of ownership for AI projects.
Prioritize infrastructure that promotes easy experimentation, iteration, and proofs-of-concept.
Opt for solutions that seamlessly integrate within a wider AI ecosystem.
Consider how to optimize data labeling, model training, and deployment.
Reduce the time spent manually managing infrastructure.
Leverage a solution equipped to evolve with the rate of industry change.
By tackling the above, you can ensure your team is set up to succeed while ensuring time isn’t wasted on repetitive tasks - dramatically fuelling your return on investment in the process.
Weighing up whether you should build or buy your training data operations software? Download our free guide which maps out the pros and cons of each option.
Cost estimations
Cost estimation for AI projects is complicated. Depending on how you approach your development, you may have to manage costs across software, hardware, cloud services, system software, and talent. Below, we’ve included a framework to help you ask the right questions when estimating the costs of investing in AI.
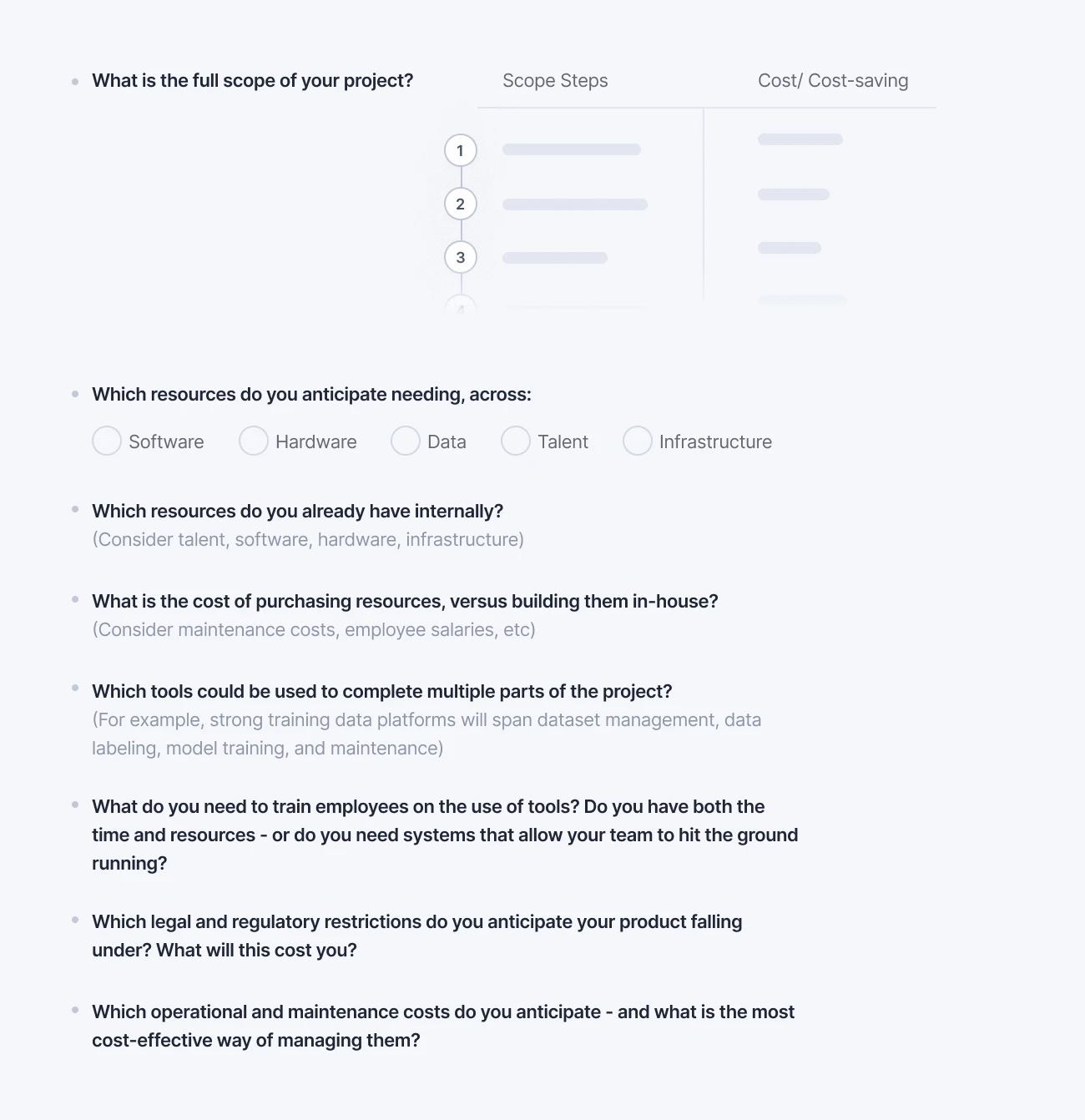
Cost-saving opportunities in AI
With the right infrastructure in place, the actual process of creating AI can result in greater cost-savings than underdeveloped frameworks, from faster POCs (which allow you to create better products overall) to more reliable products that secure higher returns on the market.
Below we’ve collated just some of the cost savings organizations can expect when they’re strategic about how they invest in their AI operations.
Faster POCs, better outcomes
With the right tools on hand - you can rapidly create POCs to test your product hypothesis. Better yet, mature infrastructure will allow you to create POCs that are more accurate by nature, in less time than underdeveloped tools. For example, V7 customers report a 33% faster release of models and a 25% reduction in errors on average, within just 6 months of implementing the platform.
These same customers boast model accuracy rates in the 90th percentile and month-long pipelines that turn into weeks. These are costs saved not just in establishing product viability, but also in staffing, development time, and infrastructural overheads.
Investing in fit-for-purpose tooling will allow you to quickly experiment, pivot, and ultimately create better products that spend less time in production - securing a better ROI in the process.
Standardization
Mature AI infrastructure will embed efficient and repeatable development processes, which maximize productivity and minimize the costs of production overall. These cost-effective production lines allow businesses to reach a profitable market position faster, beating the competition in the process, and securing more investment for future product development.
Time and resource allocation
AI can be used in the development process of your AI-powered products, to automate repetitive tasks to ensure humans in the loop are only working on the most crucial tasks. For example, you can train an AI model to label new data, which can then be fed back into the model for improvement. This allows you to rely on a trustworthy AI to complete parts of the training process (fueled by human expertise) allowing you to more intelligently allocate available time and skills.
Model performance
The performance of your model will be a pivotal factor in the success or struggle of your product. With mature AI operations, companies can expect industry-average-exceeding model accuracy rates in less time. These highly performant models result in happier customers, more robust products, and larger financial gains.
Reduced cost of ownership
Mature AI capabilities help to reduce the overall cost of ownership of AI. For example, solutions built in-house often need continuous maintenance, requiring highly skilled engineers to monitor upkeep. Similarly, as the needs of AI evolve, you need to invest time, money, and talent into evolving your platform to remain competitive in its output; resources that could be otherwise spent on product development. In the words of V7 Co-Founder and CTO, Alberto Rizzoli,
“AI re-invents itself every 18 months with major breakthroughs. As such, time is your biggest expense. Building on a "previous generation" stack leads to worse products, and a loss of talent that wishes to push the bleeding edge. The two biggest time sinks in machine learning are data labeling and internal tool development, both of which can keep progress hostage for months. Outsource these whenever you can.”
Overwhelmingly, mature AI businesses in 2024 are reducing the cost of ownership by investing in ready-built platforms that shoulder the financial and resource costs associated with platform upkeep.
Analytics and reporting
Without analytics and a clear reporting system, it will be difficult to see firstly, where issues are in your development pipeline, and secondly, to understand how to improve them. For example, how long is the labeling process taking? Does it align with the industry average? How does it compare in terms of annotation accuracy? A mature, ROI-securing organization will invest in clear analytics and reporting that allows it to measure a range of metrics, including the efficiency of its labeling teams, the accuracy of annotations, and the performance - or drift - of its AI model. Unfortunately, many businesses are, for all intents and purposes, moving in the dark - creating AI will little understanding as to where financial losses are most prevalent.
Platforms like V7 include reporting dashboards that allow businesses to do all the above - and more - allowing you to continuously create cost-effective development pipelines that deliver better products overall.
V7’s reporting system has gone through a series of changes, with improvements to the tracking, analysis, and optimization of data annotation workflows. Check out our most recent product update to see the full suite of reporting features in the platform.
Predicting ROI
By now, you will have addressed the estimated cost of your AI investment, alongside some of the cost-saving strategies you can implement. Next, let’s explore predicting the ROI of your investment.
This is a complicated task that involves addressing upfront costs, maintenance, short and long-term savings, and finally, estimated returns. These returns could be market returns, or internal gains, such as productivity gains that in turn strengthen the profitability of your business.
The ROI of your AI will be impacted by a broad range of factors - and as a result, there’s no clear-cut formula you can use. However, for an example, let’s look at the potential returns that come with investing in mature training data operations software. This will help showcase how you can secure ROI based on a strategic approach that prioritizes best practice frameworks.
ROI example: Buying training data operations software versus building it
The build versus buy argument is ongoing in the world of AI, with pros and cons for each method. While we’ve gone into detail on this topic (explore our build versus buy guide to get the facts) it helps to have a quick recap.
In the early days of enterprise AI, companies were often forced to build their own AI/ML infrastructure. Unfortunately, this approach was prone to failure and complexity - with an investment of time and resources that often limited the overall profitability of AI.
Fortunately, the industry has dramatically improved since, with the MLOps ecosystem now brimming with options. Today, the AI Infrastructure Alliance (AIIA), recommends against building your own MLOps tools, citing the complexity and overall costs of building your own solution.
Below you can see a comparison of in-house development expectations, versus the benefits a company can expect from a training data operations platform.
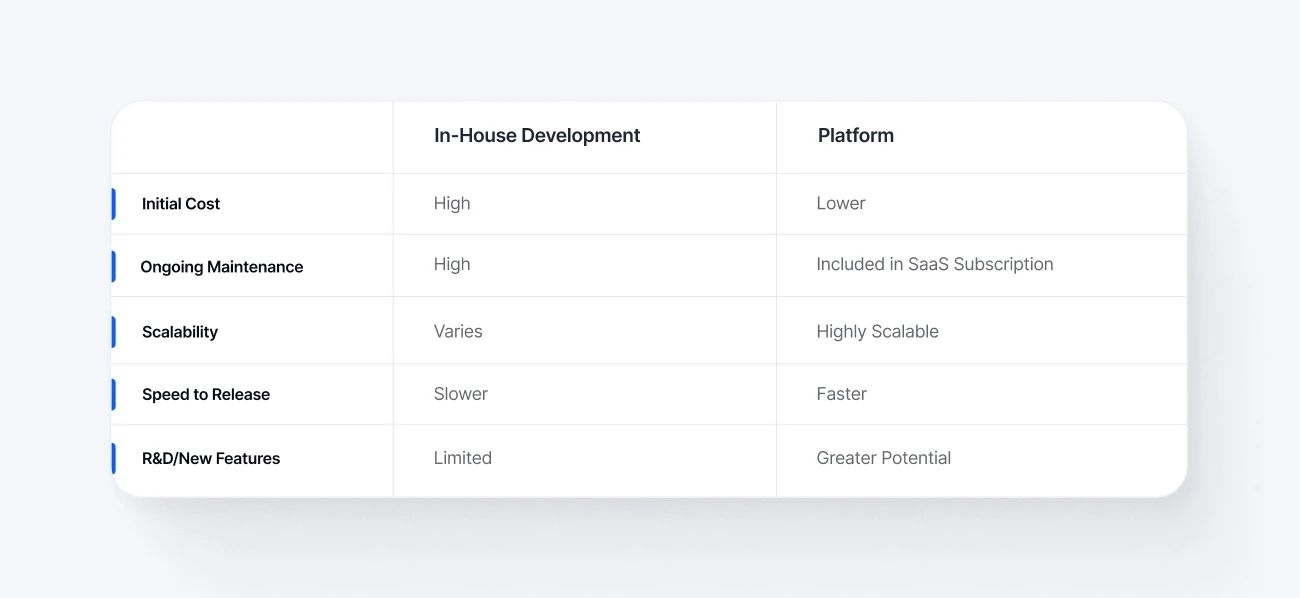
Today, companies seeking to make tangible profits scour the MLOPs ecosystem and collate the infrastructure that best suits their needs.
Let’s get into a bit more detail. What are the cost-savings and potential revenue gains of opting for training data operations software? Below we’ve compiled one example that highlights the efficiency gains of training data software. Without effective AI/CV/ML infrastructure in place, companies stand to sink costs on annotator salaries, hours spent on labeling, time spent fixing errors, quality assurance, and the overall efficiency of their machine learning and data science teams.
The below highlights how just under $200K could be gained with an effective training data process.
Day-to-day efficiency gains of training data software operations
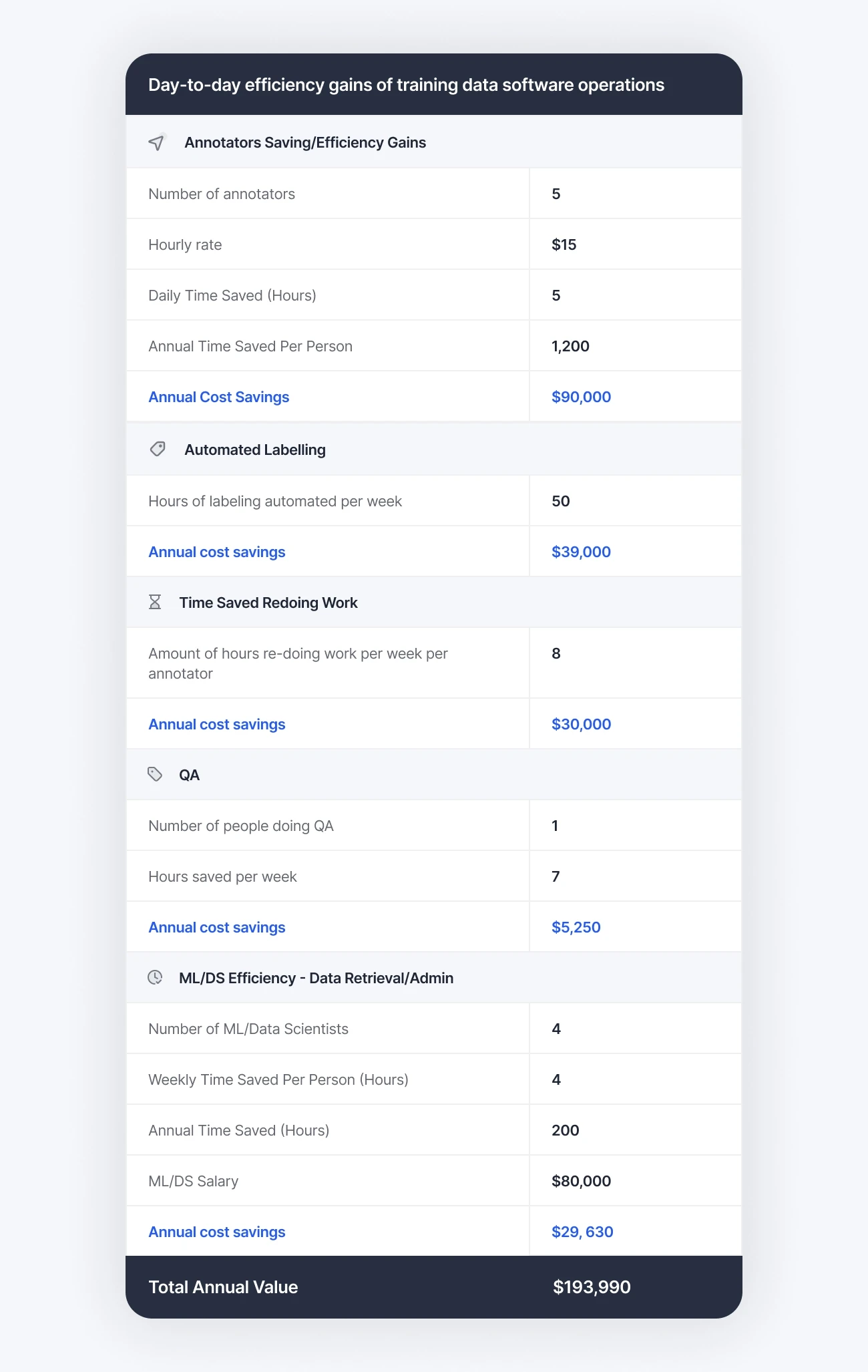
This small example quickly highlights where projects lose funds, time and time again - and how mature tooling can plug the gaps and create more profitable development pipelines.
Take the time to explore best-in-class tooling for each component of your development pipeline and be sure to measure the potential savings that can come with each solution.
Address adoption
Investment returns from AI are slowly on the rise, in no small part thanks to the increasing AI maturity of businesses. These organizations haven’t just added AI to their technological bow, they have worked hard to promote the seamless adoption of AI and its tooling within their business. This ranges from standardization of development, right through to educating teams on the benefits, risks, and ethical hurdles of AI. Put simply - investing in AI tooling is half the battle, the other half, is tackling the AI culture your business promotes.
Perhaps one of the greatest challenges facing AI - in the context of the wider world - is the uphill battle to earn the trust of its users. In terms of ROI, lacking trust in an AI will result in poor product adoption, a limited user base, and diminishing returns. Businesses that invest heavily into the trustworthiness of their AI, alongside data explainability and standardizing their process, see greater returns than those that deprioritize the impact of a trust-first culture.
2.5 times, in fact, according to IBM.
Ensure you secure the value from your AI investments, by educating your teams on the importance of trustworthy AI. This will impact the performance of your model, the pace of your development, the viability of your product, the trust of your customers, and overall, the ROI of AI.
Maximizing AI ROI and extra resources for assessing your spend
AI continues to grow in popularity, with product launches from global incumbents like Google, Adobe, Github, and Notion - to name just a few. As products continue to hit the market, a clear route to ROI is coming to the fore; one which prioritizes a strategic approach to development, investment into mature AI tooling, and a considered approach to AI adoption. While ascertaining the ROI of AI remains complex, there are a number of resources available to get you started. Check out some of the below to help you decide the best infrastructure for your use case.
Training Data Ops Software: Build or Buy (A close look at the pros and cons of each approach)
Training Data Platform Buying Guide: A collection of free worksheets to help you compare providers
Build successful AI with V7
V7 provides training data operations software that is consistently ranked by G2 as a leader in its ability to turn customer data into trustworthy AI. Customers like Boston Scientific and Continental rely on us for their training data operations, dataset management, and human-in-the-loop element, earning, on average, a 33% faster release of models and a 25% reduction in errors on average within just 6 months of implementing V7.
Trusted by household names, and backed by industry greats, we’ve spent half a decade helping customers to build better, and faster AI products that dominate their market.
Preparing to embrace AI?
Try V7’s Darwin and see how you build an accurate and efficient product development pipeline.