Knowledge work automation
14 min read
—
Dec 4, 2024
Get ready to apply AI workflows to accelerate complex tasks

Content Writer
Artificial Intelligence (AI) is a transformative force reshaping how we work. Similar to the way the printing press democratized access to information and knowledge, AI is democratizing data analysis and decision-making. But it’s not about machines taking over; it's about leveraging their power to automate and streamline workflows.
In fields like law and insurance, research that once took hours can be completed in seconds; in healthcare, AI is helping doctors diagnose diseases and streamline patient care. Every industry can benefit from AI workflow automation.
In this article you'll find clear and practical information on AI workflow automation and how and why you'll want to get started right away. We’ll cover:
What are AI workflows? And how AI can be used safely and effectively for automation.
AI and supporting technologies you can expect to use in automating business processes.
Use cases and guidance on how firms can effectively integrate AI into workflows.
By the end of this guide, you’ll have a solid background on AI workflow automation and how you can use it to transform tasks and processes no matter your industry or technical experience.
Can’t wait? You don’t need to. Jump into AI workflow automation with easy, intuitive V7 Go. It’s the knowledge work automation platform to solve complex tasks at scale.
What is AI workflow automation?
AI workflow automation combines AI and automation technologies to complete complex tasks for you and get work done faster.
For example, AI workflow automation can quickly sort through large volumes of intricate documents, such as contracts or forms, pull out relevant information, and present you with the key insights you need.

Additionally, you can set up a complex sequence of steps and operations based on results.
Technologies used in business workflow automation
The magic of AI workflow automation comes together through many complementary technologies that have evolved over the past few decades.
Starting with basic OCR that grew into RPA to automate clear cut business processes, workflow automation capabilities expanded with newfound applications of AI, like IDP, to transform unstructured data. Today, the current evolution of business workflow automation carries earlier tools forward while expanding automation possibilities by an order of magnitude by employing generative AI.
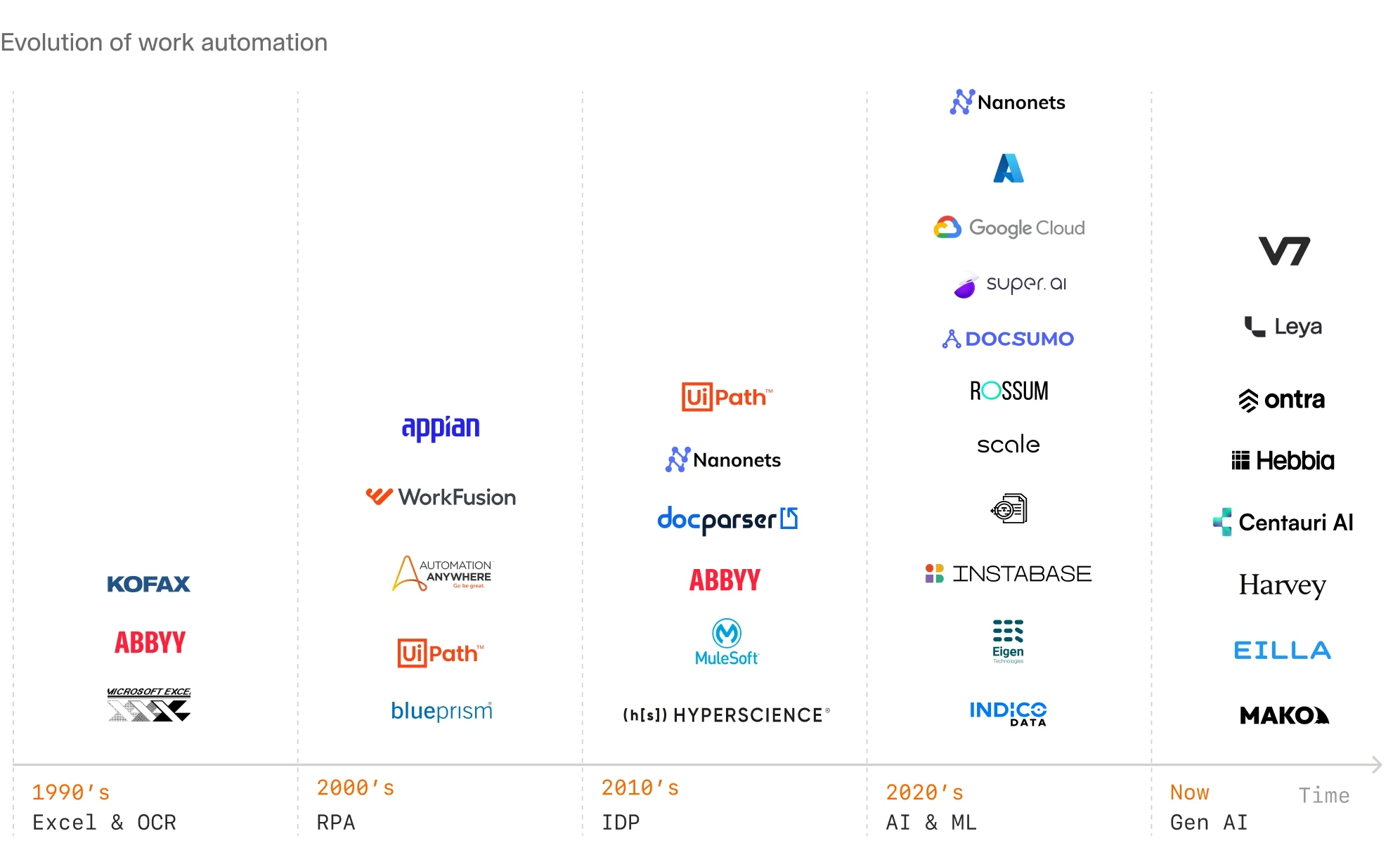
Depending on the type of process to be automated, you'll need a number of these separate yet connected tools and capabilities to accomplish the various tasks involved. So understanding the technologies and capabilities that make up workflow automation systems can be helpful.
Several key technologies stand out as the core set of power tools enabling AI-driven workflow automation solutions:
Optical Character Recognition (OCR) to digitize data
OCR technology converts different types of documents, such as scanned paper documents, PDF files, or images captured by a digital camera, into editable and searchable data. Tools like Adobe Acrobat and even Microsoft Word are common choices for businesses seeking to digitize documents efficiently. Selecting the right OCR tool largely depends on your particular use case. In many, if not most, business applications of OCR today, software that extends beyond OCR to automate document processing and data extraction is a must.
Robotic Process Automation (RPA) to automate structured tasks
RPA is software to automate highly repetitive and routine tasks usually performed by a human interacting with digital systems. One of its defining features is its simplicity to set up and use. Because RPA executes tasks following the same user interface paths made for humans, including copy-paste and logging into applications, configuring RPA workflows does not typically require special coding skills. UiPath and Automation Anywhere are major players in this field, allowing businesses to automate processes like data entry and transaction processing. By reliably executing tasks on repeat and at scale, RPA remains a core technology for automating workflows.
Intelligent Document Processing (IDP) to extract and transform complex information
IDP combines OCR, machine learning, and natural language processing to automatically extract and structure information from documents in diverse formats. Intelligent document processing represents a practical application of AI and has proven to be essential for creating end-to-end process automations when facing document-heavy workflows.
Generative AI (GenAI) to create content and enable automation flexibility

GenAI models, such as those developed by OpenAI and Google, are AI foundation models that can generate human-like text, as well as images and other media types. GenAI also brings flexibility and adaptability to processes where rules-based automation tools like RPA would otherwise fail.
Large Language Models (LLMs) to analyze data at scale and power autonomous workflows
LLMs like GPT-4 and Claude are designed to understand and generate human language, making them ideal for tasks such as summarizing legal documents or analyzing large volumes of text data. LLMs also excel in dynamic environments, able to plan and respond to inputs in context. They are the engine of next-generation AI workflows called agentic workflows, enabling a new level of autonomy and intelligence in automated processes. These workflows are able to learn from interactions and make decisions without constant human oversight.
Other technologies
Other AI technologies include machine learning algorithms for predictive analytics, natural language processing for sentiment analysis, and computer vision for image recognition, all contributing to a comprehensive AI toolkit for workflow automation.
Business automation using AI: Key benefits
Organizations big and small are eyeing the immense potential value of AI and seeking to bring home the benefits. Estimates of AI’s projected value are so large they can be hard to comprehend; PwC for example, forecasts no less than a $15.7 trillion contribution to the global economy by 2030.
So it’s no surprise that automating workflows with AI is fast becoming the standard approach to business process automation. In the next 5 years, up to 30% of hours worked are projected to be automated. And it’s easy to see why – the business benefits speak for themselves.
Improved accuracy, consistency, and scalability of operations
AI workflow automation can significantly decrease process error rates. Manual data entry and repetitive tasks are prone to human error, variability, and uneven execution. With AI-driven automation, tasks are performed with precision and consistency, ensuring more accurate, predictable results and improved data quality. Having more reliable processes and data goes a long way in supporting business decisions and client trust.

Consistency and accuracy are part of an AI-powered foundation for business operations that includes increased scalability. During periods of high demand, AI systems can handle higher workloads without compromising quality or accuracy. This means that as business grows or during demand/volume spikes, operations can smoothly scale in tandem, supporting business continuity, resilience, agility, and growth.
Increased productivity and cost savings
Anticipated AI-led economic impact is directly tied to increased productivity, with more than one-third of forecasted value attributed to productivity gains. As AI workflow automation takes over mundane and repetitive tasks, human resources are made available for more complex tasks, strategic projects and roles – contributing to a related benefit: higher work satisfaction.
While cost savings are another side of the coin, so to speak, of productivity improvements, it is imperative to avoid the illusion of reducing costs when they come at the expense of quality.
Yes, through automating workflows with AI, firms can realize substantial cost savings from multiple angles: automating workflows decreases costs by reducing reliance on labor, minimizing errors and rework, and increasing efficiency which all contribute to reduced operating costs.
But, importantly, AI for knowledge work is about a human-AI partnership. This co-pilot approach mitigates the risk of hollowing out processes from blanket application of AI workflows without keeping humans in the loop.
Better compliance and risk management for critical sectors
In industries like finance, healthcare, and legal where regulatory compliance is paramount, AI can continuously monitor operations, flagging irregularities that might indicate potential risks or non-compliance issues.
In loan and insurance applications, AI can automate credit risk assessments with a workflow that expedites each step of the process from data collection through risk scoring and ongoing monitoring:
Data collection and integration.
The workflow begins with aggregating data from multiple sources – including credit scores, financial statements, and transaction histories – and consolidating it within a central system for analysis.
Analysis and pattern recognition
Machine learning AI algorithms analyze the collected data to identify patterns and trends that may indicate credit risk, with improving accuracy over time as the models process more data.
Risk scoring and decision making
Based on the analysis, AI assigns a risk score to each applicant to inform the credit approval decision. At this stage, AI can also suggest personalized loan conditions to reflect an applicant's risk profile.
Continuous monitoring
Once credit is extended, the AI workflow can include ongoing monitoring of borrower financial behavior to detect and flag early warning signs of potential default, like unusual spending patterns or missed payments.
This kind of AI workflow not only accelerates the process, supporting proactive risk management to avoid potential losses, it also improves accuracy and reduces possible human bias.
Tapping into the benefits of automated AI workflows starts with identifying tasks that can be automated and adopting intuitive, AI-first solutions like V7 Go that can easily integrate into business processes.
Workflow automation use cases
AI workflow automation is about versatility. Where automation used to reach a dead end, AI enables flexibility and adaptation to move the process forward. In fact, until recently, automating – even when described as AI workflows – often meant using Python scripts or connecting different apps via Zapier.
Now, multimodal AI enables solving complex problems by connecting AI models together. This can be accomplished by applying connected AI models, or chaining general-purpose AI language models (GPTs), where each model is tasked with executing a specific role or discrete part of the overall process, ultimately working through an entire workflow, step by step.
An example of KYC verification automated with V7 Go
These advances have further expanded an already jaw-droppingly diverse range of use cases for AI in workflow automation. And as AI technology continues to evolve, its potential applications will only expand.
For example, in data-intensive industries, AI-powered tools excel at processing high volumes of unstructured documents quickly and accurately. Solutions like V7 Go automate the extraction of essential data from contracts, forms, and reports, reducing manual data entry errors and freeing up time for more value-adding and complex tasks.
In the finance industry, AI tools have been adopted at an accelerated pace across high-value use cases including personalizing customer experiences, assessing risk, and credit scoring. Banks in particular have leapt at the opportunity to apply AI to automate fraud detection, with nearly 60% of surveyed banking institutions using the technology for this purpose.
However, adoption of AI in professional service contexts has been uneven–attributed primarily to low investment in AI tools at smaller firms. But that may quickly change, as accessible, low-cost yet sophisticated AI-powered workflow automation solutions are leveling the playing field for firms of any size ready to accelerate and scale workflows by harnessing AI.
Document processing and data extraction
Unstructured data already makes up most of the data in the world, with ever-increasing volumes of documents and data. And business data is no exception.
AI technologies like OCR, NLP, and machine learning can streamline the transformation of unstructured data into valuable, accessible information, where the combination of document volume and complexity would overwhelm a manual approach.

The flexibility of AI for processing data has made data pre-processing obsolete. Think of case-specific data gathering mechanisms built to meet technology solutions halfway, like the machine-readable zone on passports. AI eliminates the need for manual data categorization and set up – for example, Google photos tags your images with the right person and place, all automatically. The exciting story here is that AI can work with data in any format right from the get go.
By extracting and converting unstructured data into structured formats, AI makes information usable for any business process including analytics, reporting, and strategic planning. And AI solutions can easily scale to accommodate growing data volumes, making them ideal for firms to match data processing capabilities to their needs without a proportional increase in costs or resources.
Want to find the most effective tool for your data extraction use case? Check out our handy guide to the best data extraction tools powered by AI.
Contract review and compliance checks
AI models are a natural match for the complexity of contract and compliance reviews, speeding up the review process and ensuring documents adhere to regulatory standards, minimizing risks and boosting legal team productivity.

The combination of GenAI, natural language processing (NLP), and machine learning, along with OCR tools, can parse and analyze contracts to identify key terms, potential risks, and compliance issues. AI automated systems can cross-reference contract terms with current regulatory requirements, highlighting any non-compliance issues to minimize legal risks and help firms maintain compliance with industry standards, ultimately reducing the likelihood of legal disputes and penalties.
The bottom line is that AI systems can process contract documents much faster, more accurately, and in more detail than human reviewers, providing legal teams with much-needed speed and support.
Financial report analysis
AI technologies have proven pivotal in automating and enhancing financial report analysis. Able to process complex financial datasets from multiple sources with accuracy and speed, AI-driven workflows can produce financial insights that support strategic decision-making.
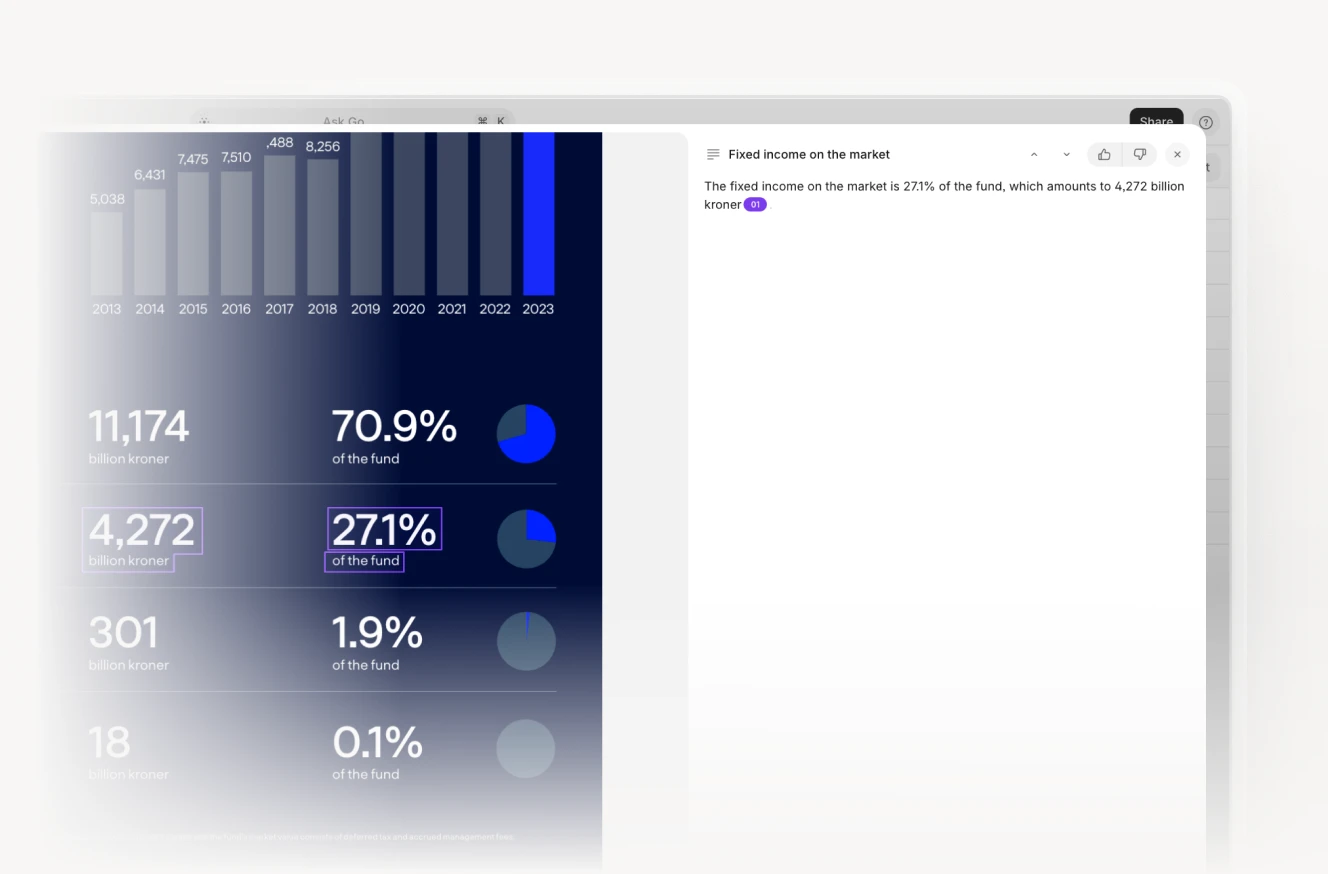
For example, to support financial portfolio analysis, AI can gather and parse data from multiple sources, including interpreting complex charts, to find and extract key data points like asset allocations, performance against benchmarks, gross margins and cash flow.
Learning from historical data, along with continuous improvement over time, enables AI models to forecast financial performance and potential risks, providing context for strategic planning and investment decisions.
Natural language processing (NLP) complements machine learning AI models by interpreting and analyzing qualitative data within financial reports to extract pertinent details from sections like management discussions and market analyses.
And with GenAI, it's possible to automate the creation of summary reports as well, completing an end-to-end AI-automated workflow for financial reporting and analysis.
Supply chain and logistics optimization
As the complexity and scale of supply chains grows, automating with AI workflows offers solutions to streamline processes, improve decision-making, and enable flexibility and resilience.
For supply chain optimization, machine learning and predictive analytics are core AI tools for improving the accuracy of demand forecasting to better predict customer needs and maintain inventory levels.
In logistics, AI can automate document-based workflows like shipping label processing by understanding context and adapting to any label format to maintain high accuracy even with damaged or low-quality source information.

Route optimization is another opportunity to apply AI in logistics, harnessing the complex combination of real-time traffic data, weather forecasts, and delivery schedules. This AI workflow would determine the most efficient transport paths to improve logistics performance including reduced fuel consumption, lower costs, and timely delivery.
The Internet of Things (IoT) complements these applications of AI. Real-time data from connected devices across the supply chain is valuable data to feed AI models, accelerating the improvement cycle and making AI workflows rapidly more effective in every application, whether monitoring inventory levels, tracking shipments, or managing assets.
Content generation and summarization
You don’t have to be in a creative industry to notice that AI technologies are redefining how content is created and summarized, changing the status quo for productivity, creativity, and accuracy in content workflows. In the legal, financial, and professional service industries, AI is revolutionizing document processing, reporting, and communication through advanced automation.
For law firms and legal teams, AI tools can draft contracts, case briefs, and compliance reports by analyzing historical documents and legal texts to generate accurate content following legal standards. AI-driven summarization helps legal professionals quickly distill large volumes of case law or legislation.
In a financial context, generative AI can create personalized client communications, from financial advisories to performance reviews, that are tailored to client needs and preferences.
And across professions, employing AI workflows to accelerate content creation and summarization enables professionals to quickly consolidate information and generate insights.
What type of businesses can benefit the most from AI workflow automation?
After record-breaking consumer adoption following its debut, generative AI adoption by business has begun to pick up speed. Early applications of GenAI within organizations – most common in marketing and sales, product and service development, and IT – are the leading edge of broader AI adoption, which has jumped year-on-year by nearly 50%.
Adopting AI to automate workflows presents significant opportunities for businesses that work with complex and unstructured data, including:
Law firms and legal teams to accelerate case law research and compliance checks and streamline drafting, analysis, and management of legal documentation.
Insurance firms for use cases across data analysis, claims processing, and risk assessment.
Healthcare administration and delivery for data-heavy tasks like creating personalized patient care summaries.
And in every industry, AI for customer service workflow automation offers significant value – AI-powered chatbots and virtual assistants can handle client inquiries efficiently, provide personalized support and free up human resources for more complex interactions.
How to use AI to automate tasks and business processes
Whether through accessible no-code platforms, comprehensive enterprise solutions, or custom AI applications, choosing how to apply AI for your use case and business context is probably the most important step to using AI effectively to automate tasks and processes.
No-code AI workflow builder for OCR, IDP, and LLM orchestration
One of the most accessible ways to integrate AI into business processes is through no-code AI workflow builders. These tools are designed for tasks like invoice processing and contract reviews that require orchestrating OCR, intelligent document processing (IDP), and large language models.
No-code solutions for building AI workflows empower firms to automate transforming unstructured data into usable formats without requiring extensive technical expertise. The power of these tools is their ability to extract and transform key information from complex documents such as invoices, contracts, and forms, making the right data available for integration into business systems and workflows.

A prime example is V7 Go, an end-to-end solution for document processing and data extraction. Applying advanced OCR capabilities and IDP, it converts both complex documents as well as scanned images into editable and searchable digital text, significantly reducing manual data entry time and error rates.
Importantly, V7 Go incorporates LLM orchestration to enhance document comprehension and contextual understanding. This allows businesses to deploy AI models that can perform tasks such as summarizing long reports, extracting insights, and even automating customer support responses. Its no-code interface ensures that users can design and implement workflows quickly, making it an ideal choice for companies looking to boost productivity and accuracy without significant investment in technical resources.
Enterprise AI for process automation
For larger organizations, enterprise AI solutions offer robust frameworks for process automation, designed to handle complex and large-scale operations. Companies like IBM and AWS provide comprehensive platforms that integrate AI with existing enterprise systems. These are, however, much more complex to implement as they typically rely on multiple modular solutions.

IBM's AI solutions, for instance, incorporate machine learning, AI-driven analytics, and natural language processing to automate tasks across IT operations, customer service workflows, and supply chain management. These enterprise tools are engineered to scale with business needs and provide flexibility to customize AI models to specific enterprise requirements. This process of course requires significant technical and business resources.
These traditional frameworks are particularly beneficial for organizations with the infrastructure to support advanced AI deployments as part of enterprise process automation efforts.
Building custom AI solutions based on model training or fine-tuning
For firms that require tailored AI applications, building custom AI solutions through model training or fine-tuning offers unparalleled flexibility and precision. As custom implies, this approach involves technical knowledge and hands-on configuration work to prepare data and train and deploy the customized model.
Building a custom AI solution starts with data preparation, which involves gathering and organizing data that accurately represents the use case domain so that AI models are trained on high-quality inputs. Annotation tools like V7 provide key infrastructure for effective data labeling and annotation to streamline the process of working with extensive datasets.
Once prepared data is in hand, the next step is model training. This typically involves developing/refining machine learning algorithms to perform specific tasks. Fine-tuning pre-trained models is a great way to expedite this process. Adapting existing algorithms to new applications requires less data which saves time, cost, and effort.
Building custom AI solutions, while a non-trivial project, has the benefit of addressing unique business scenarios and opportunities – and can achieve automation that aligns most closely with strategic goals.

Best practices for implementing AI automation
Successful AI implementation for intelligent workflow automation does not happen in a vacuum; taking into consideration the bigger picture of business needs and requirements will have a major impact on implementation outcomes.
A successful AI workflow must balance the power of automation with practical considerations including governance, data security, and scale.
The good news is that there is already a lot of great advice available, and it’s just waiting to be used to help put your implementation of AI on the right track.
Understand the problem and break it into steps
Before introducing AI into a workflow, thoroughly understand the problem you aim to solve. Complex workflows can often be broken into smaller, more manageable components. Each step should align with what AI can solve effectively given current capabilities. For instance:
Identify where AI adds value. Does your workflow involve unstructured data (e.g., scanning invoices or extracting insights from customer reviews)? AI excels at structuring this data.
Divide tasks by best-fit technology solution. Take a financial reporting process as an example. AI could extract figures from raw documents, but final calculations, such as tax adjustments or projections, should use specialized Python libraries like NumPy or pandas.
By isolating tasks like these, workflows won’t over-rely on AI in areas where alternative tools are more accurate or cost-effective.
Select the right model for each task
Choosing the right AI model should not feel like a guessing game. Selecting the right-fit model for a particular project minimizes waste and drives reliable results. Balancing accuracy, performance, and resource costs is possible when selecting AI tools. Consider:
Optimize for cost vs. performance. If your use case doesn’t demand high complexity, GPT-4o mini could reduce token costs while delivering adequate results. However, GPT-4o might be necessary for deeper or contextual understanding. For example:
A chatbot for basic FAQs could suffice with a simpler model.
Researching nuanced legal cases might demand the higher sophistication of GPT-4o.
Apply multimodal models where needed. Does your workflow require text, image, or voice data processing? Multimodal models like GPT-4V (vision enabled) could be valuable, but only if features will be used in full.
Combine AI and traditional coding when possible
AI automation is not necessarily about replacing existing tools—it should complement them. Consider hybrid workflows where both AI and traditional automation approaches are combined for maximum efficiency. It's a mistake to force AI into every step of a process; focus on where it can save time or add insight where traditional methods fall short.

For example:
Financial workflows: AI can extract or analyze text from annual reports, while numerical calculations (e.g., interest rates or portfolio metrics) are better handled by proven Python-based libraries.
Data pipelines: AI can parse and clean raw data (e.g., social media sentiment analysis), but the integration and storage could rely on Python scripts or SQL-based databases for infrastructure.
Ensure data quality and relevance (or not)
When developing a new AI model, optimal performance requires high-quality input data. Models rely on the clarity, consistency, and relevance of data fed into them. In fact, when developing custom AI models, data labeling is a critical part of the process.
That said, when applying existing AI tools and out-of-the-box solutions, advanced AI workflow automation platforms are now able to do the data heavy-lifting for you without additional training. They can work with low-quality source data – think low-light snaps of crumpled receipts – and still extract everything you need.
In all cases,
Use tools that can integrate information from multiple platforms, such as shared databases or systems of record.
Look for models pre-trained on domain-specific data. A healthcare chatbot, for instance, will perform better if trained using medical conversations rather than generic public datasets.
Optimize AI prompts to reduce costs and improve accuracy
The majority of AI workflows can and should be optimized with the right prompting techniques. It's about understanding your model, refining your approach, and iterating prompts accordingly. By paying attention to prompts, you can dramatically improve your AI's performance. While AI model quality matters, in reality, a good model combined with a very good prompt can surpass the results of a very good model with a bad prompt.
Prompt engineering is a strategic approach to communication with AI with the goal of crafting the right type of request to elicit the best responses from AI systems. For instance, a well-designed prompt can guide an AI system in a more targeted direction, generating more accurate and useful results.
Prompt engineering is as much a science as an art. Poorly designed prompts can lead to inaccurate results, wasted compute resources, and higher costs.
A few prompting tips:
Be specific. Detailed context in prompts guides AI towards targeted outputs. For example, instead of asking, “Extract key points from this report,” specify: “List the three most important financial metrics from this annual report.”
Consider token efficiency. Long prompts can inflate token usage. Trim prompts for concise formatting and just enough detail.
Create templates. If workflows involve repetitive tasks, consider standardizing prompt templates to ensure consistency while scaling.
Start small, then scale
Ambition often outweighs feasibility in AI implementations. Begin with small projects to get started automating with AI workflows. This approach helps refine processes, address challenges, and demonstrate value before full-scale deployment. For example, a corporate insurance firm might start by automating parts of the claims process to gauge effectiveness before extending AI solutions across all operations. Gradual scaling helps establish AI automation that is both manageable and sustainable.
Test on specific use cases. For example, a law firm integrating AI for document review could start with non-critical operational documents before tackling client-related contracts. AI can address some parts of due diligence with basic data extraction and automation.
Define performance metrics like processing time reduction or accuracy improvements. Use these metrics to validate value before scaling to larger projects.
A phased approach reduces implementation risk while delivering incremental value.
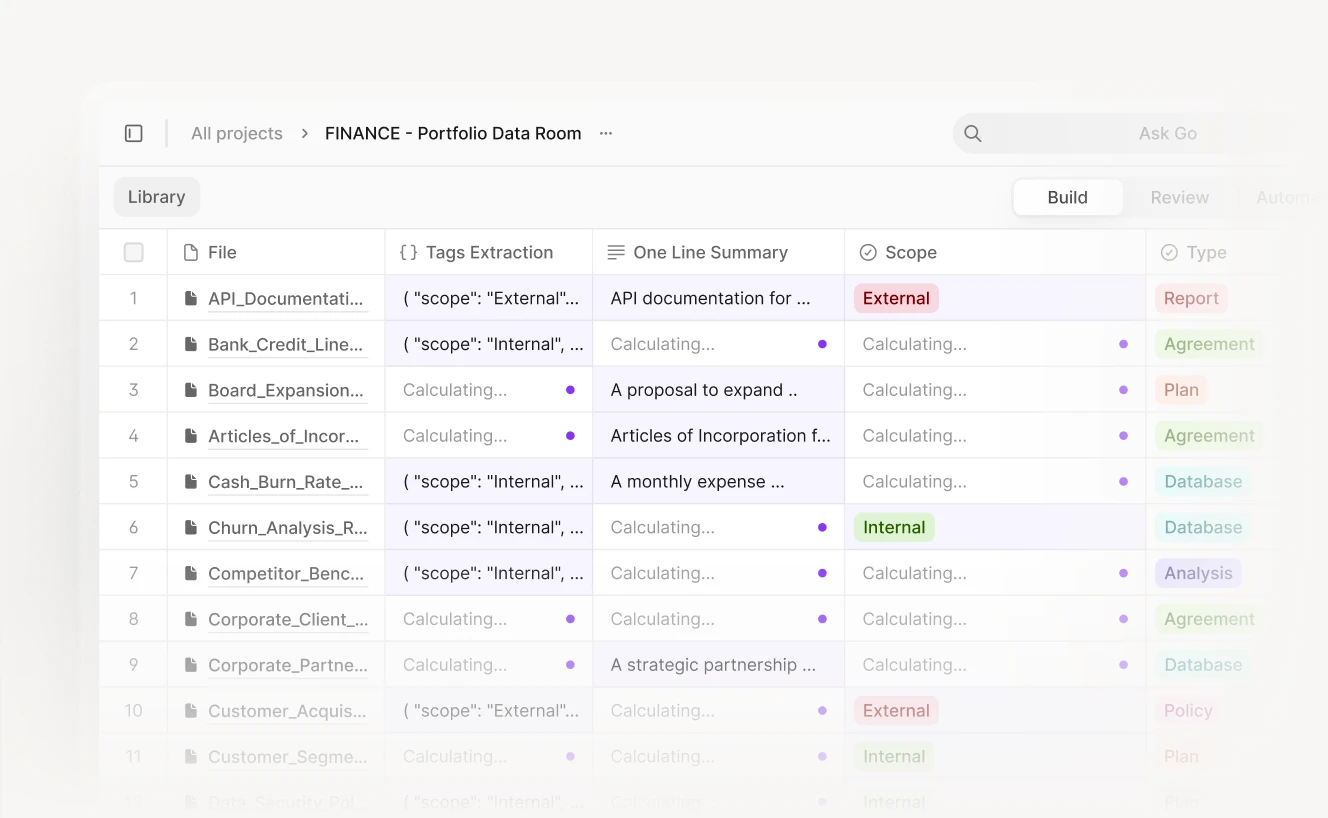
Embed governance and compliance from the start
Implementing AI automation involves navigating governance and compliance requirements. Establish clear policies and frameworks to ensure that AI applications follow industry standards and regulations, including data protection, privacy, and accountability considerations. Integrating compliance into AI workflow design phase can avoid future high-cost setbacks.
Document processes and outcomes to ensure transparency and accountability for AI-driven decisions.
Be vigilant with sensitive data. For instance, when handling medical or financial documentation, anonymize data and comply with standards like GDPR or HIPAA.
Assign oversight roles. Define roles and responsibilities to ensure effective oversight and compliance and corrective action when violations/deviations are found.
Monitor and iterate
AI workflows aren’t static—they evolve as needs shift and models improve. Continuous improvement is part of maintaining the health and effectiveness of AI workflows. Regularly evaluate automation performance and make adjustments when needed. Collect feedback from users and stakeholders to identify changes to underlying needs and business processes.
Maintain AI workflow performance by:
Scheduling periodic evaluations of AI outcomes compared to original benchmarks. This can uncover declining performance or new opportunities for optimization.
Learn from user feedback. Stakeholders that interact with outputs from the workflow are your frontline for feedback to ensure ongoing alignment with business goals.
Update models and systems. Because AI tools rapidly advance, reviewing system upgrades and new versions of AI models is a must.

Can businesses effectively integrate AI to automate workflows?
The short – and sweet – answer is: Yes.
But...
With fast-moving advancements in AI technology, it requires being open to innovation. Effectively integrating AI to automate workflows takes a firm-wide appetite for experimenting with new tools and willingness to let go of old ways of working. Successful implementation of AI automation is not just a technical challenge; it requires organizational and cultural readiness for change.
That said, getting started, even with a single workflow, can be the spark that ignites a firestorm of innovation. With the right AI solution, you can effortlessly integrate AI into workflows and empower teams to experiment with automating time-consuming tasks like document processing and data analysis – freeing up valuable time for more strategic work.
Try V7 Go. It’s an intuitive, no-code platform for AI workflow automation. No AI expertise required. Just drag and drop your data, select the desired outcome, and let AI do the rest.
With more than a decade of experience in cloud and AI, Rachel is a product marketing veteran specializing in connecting technology with real-world impact. A recognized thought leader in emerging technologies, Rachel writes extensively about work tech, applied AI, Intelligent Document Processing (IDP), and agentic automation.