Knowledge work automation
10 min read
—
Jan 20, 2025
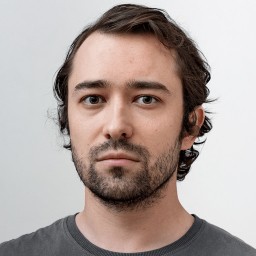
Content Creator
Private equity (PE) and venture capital (VC) firms have moved beyond experimenting with AI to fully integrating it into their operations. Generative AI tools are now central to faster deal sourcing, more accurate risk assessments, and real-time portfolio performance monitoring.
AI adoption has been rapid, with recent survey data showing that 82% of PE/VC firms were actively using AI in Q4 2024, marking a dramatic increase from 47% the previous year. AI has transformed from "nice-to-have" to a critical component of competitive strategy.
However, successful AI integration requires more than just adoption. Investment firms must navigate complex challenges in data standardization, talent acquisition, and process redesign while maintaining their fiduciary responsibilities and risk management standards.
In this article:
The role of generative AI in finance and document processing
Key technologies and use cases for automating PE/VC operations
Implementation challenges and best practices for firms
Future outlooks for the growing impact of AI on investment management
Generative AI meets investment management
The financial sector’s enthusiasm for AI reflects the demands of a data-intensive, knowledge-driven industry that depends on accuracy, speed, and strategic insights. The adoption of AI in private equity and venture capital firms presents a fascinating paradox. While junior professionals quietly embrace AI tools for daily tasks, senior partners often maintain a measured skepticism—creating what industry insiders call a "shadow AI" phenomenon.
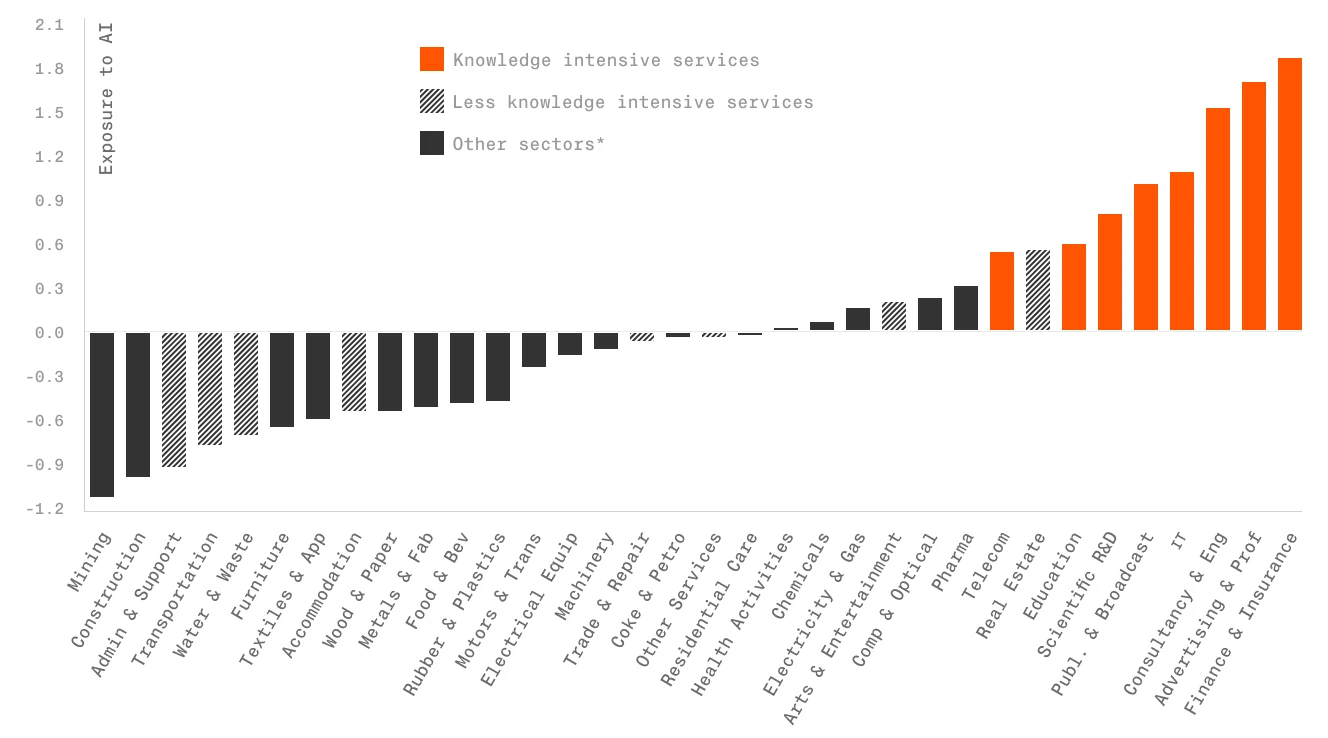
Why is AI becoming so popular, even though it still poses many regulatory challenges and remains, in many ways, uncharted territory?
A typical investment analyst at a PE firm starts their day navigating through hundreds of pages of financial statements, market reports, and due diligence documents. This manual document processing represents a core operational challenge across the investment sector.
The scale of this inefficiency is striking. Investment professionals spend the majority of their workday on manual document processing and data extraction–time that could be allocated to strategic analysis and decision-making. This creates a tangible bottleneck in deal flow and limits the depth of analysis teams can perform.
Manual processing introduces more than just time constraints. It creates significant risks of human error, especially when dealing with complex financial data. As deal volumes increase and data sources multiply, the traditional approach to document handling becomes increasingly difficult to sustain.
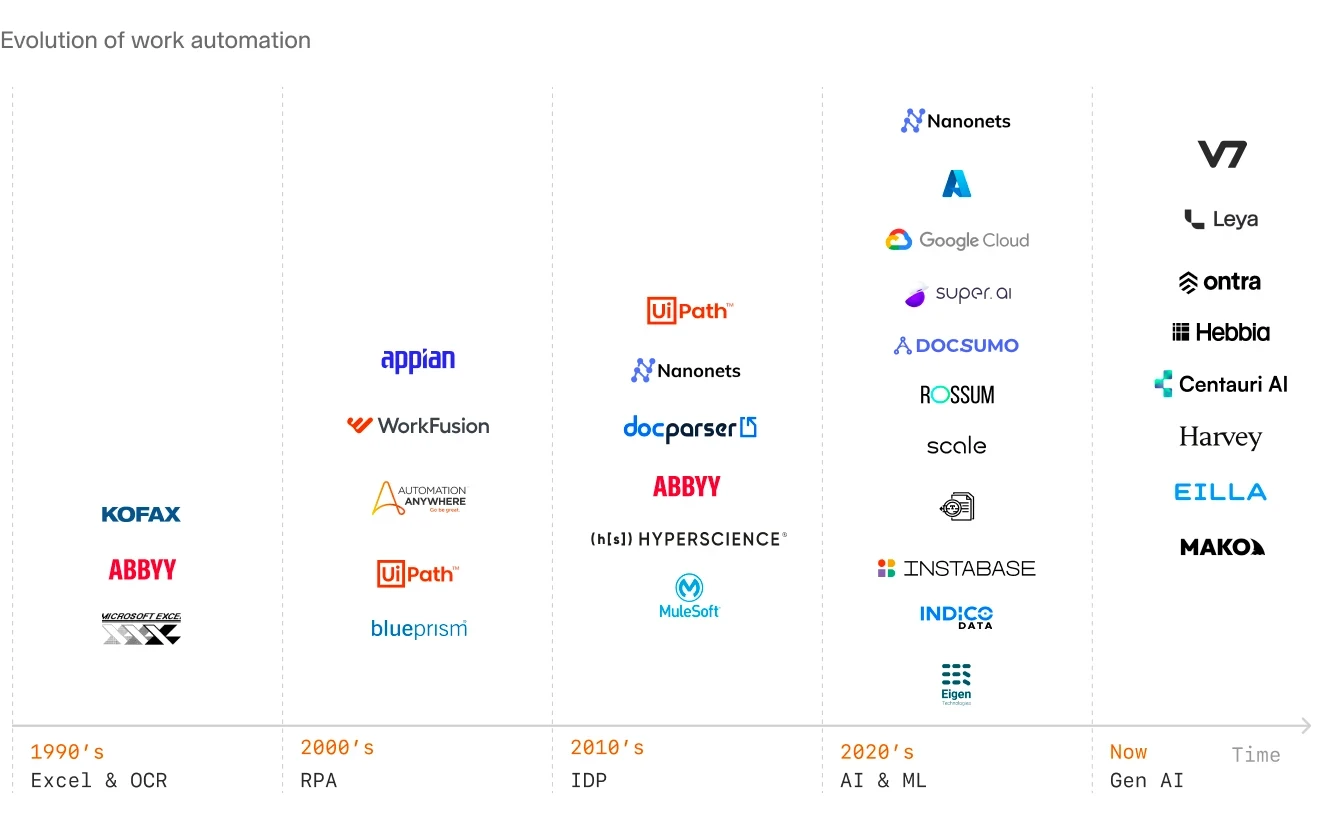
AI document processing offers a practical solution. Modern AI platforms for finance can analyze thousands of pages in minutes, extracting key data points and identifying patterns that would take analysts days to uncover manually. By automating these tasks, investment professionals can focus on higher-value strategic analysis and decision-making.
Key technologies you should understand
The adoption of AI in investment management is driven by several cutting-edge technologies, each addressing specific pain points and enhancing operational efficiency:
Optical Character Recognition (OCR):
OCR technology converts documents, scanned pages, or photos of notes into searchable and editable formats. This is essential for extracting data from legacy records, PDFs, or handwritten notes.Intelligent Document Processing (IDP):
IDP goes beyond basic OCR by using AI to extract, categorize, and process data from various document types. This technology automates workflows that involve complex data structures, reduces manual effort and improves the accuracy of extracted information.Large Language Models (LLMs):
These generative AI models are capable of processing and generating text, making them invaluable for tasks such as drafting reports, analyzing documents, and summarizing key information. LLMs can distill insights from vast amounts of unstructured data, supporting faster and more informed decision-making.Retrieval-Augmented Generation (RAG):
RAG combines the power of large language models (LLMs) with custom data, like internal documents of a business. This enables AI to deliver accurate and contextually relevant responses. More importantly, it can also ground outputs in high-quality sources or company data for easy verification. By integrating real-time data retrieval, this approach supports dynamic analysis, making AI tools more reliable for tasks like due diligence and market research.
By adopting these technologies, especially the latest LLM and RAG solutions, private equity and venture capital firms are addressing long-standing inefficiencies in their workflows. Manual processes like document analysis and data extraction can now be orchestrated with multiple LLM models chained together and automated through AI workflows.
Use cases of AI in PE/VC operations
Every year, venture capital and private equity firms collectively analyze millions of potential deals, yet only a fraction make it past initial screening. A typical venture capital firm reviews over 1,000 proposals annually and the majority of startup pitches get rejected—most within minutes of review.
But—
What if the real opportunity isn't in the deals you choose, but in rethinking how you evaluate them and streamline every stage of the investment lifecycle—from opportunity screening to back-office operational wins and portfolio monitoring?
Here are the key stages and processes that can be automated and streamlined with generative AI:
1. Deal sourcing and screening
Many leading VC firms try to optimize their deal flow by implementing AI screening. LLM-powered tools process thousands of startup pitches monthly, analyzing factors like market potential, team composition, and technological innovation. This allows analysts to focus on high-potential opportunities rather than drowning in initial screenings.
AI's pattern recognition capabilities extend far beyond screening. The technology analyzes diverse data streams—from patent filings to social media trends—to identify emerging companies before they hit mainstream radar. AI tools can analyze market dynamics, such as competitor activity or shifts in regulatory landscapes, to identify industries poised for disruption. By combining these insights, VC and PE firms can pinpoint under-the-radar startups with high potential and make more informed investment decisions.
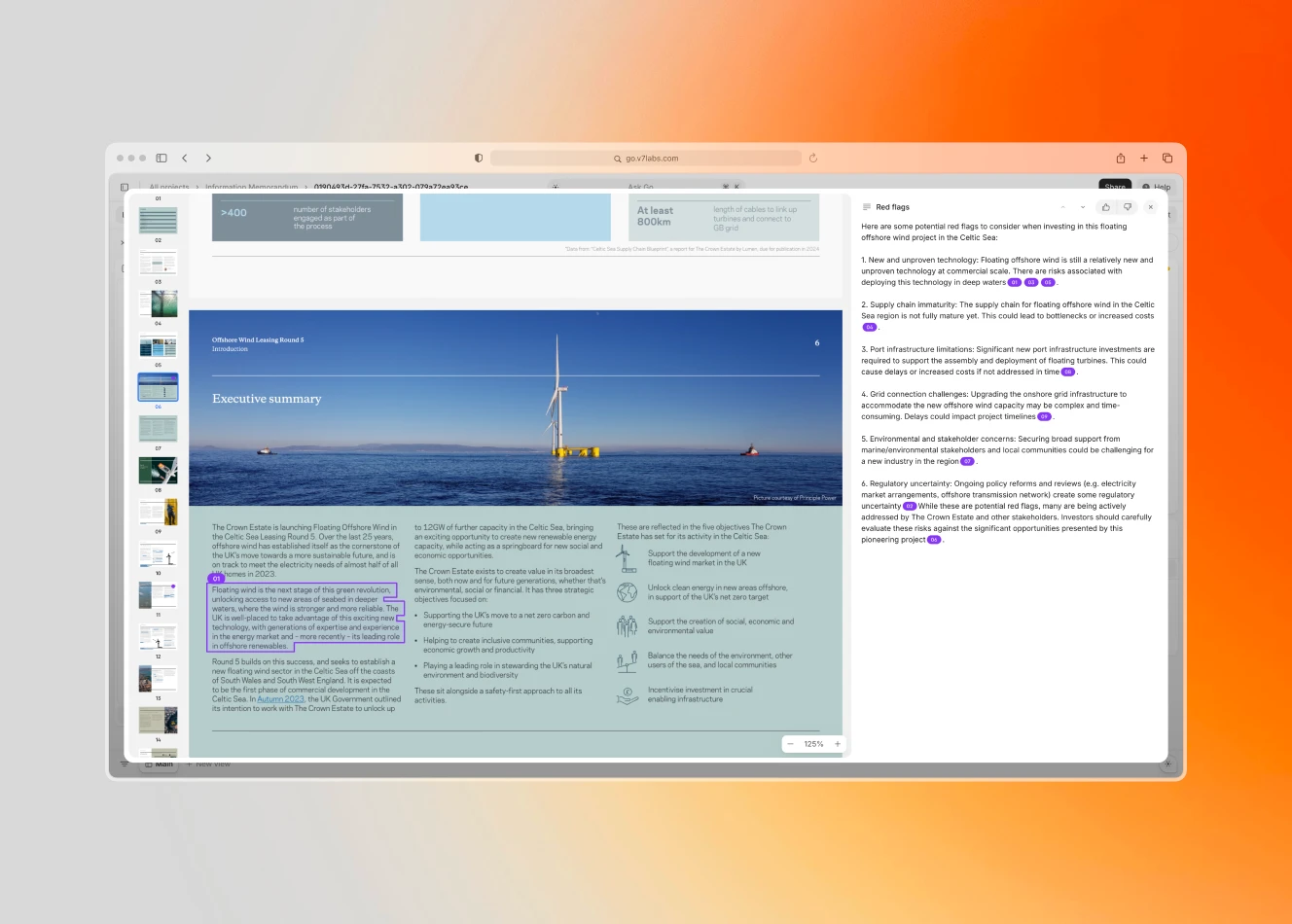
For investment firms, the stakes couldn't be higher. In a market where missing a single high-potential deal can significantly impact returns, AI provides a crucial competitive edge. The technology not only accelerates the screening process but also reduces the risk of overlooking promising investments.
Firms looking to enhance their deal sourcing capabilities can explore specialized Confidential Information Memorandum (CIM) review templates powered by AI, which provide structured frameworks for implementing these advanced screening methodologies.
2. Due diligence automation
A typical scenario involves a PE due diligence expert creating an internal database from investment memorandums using an LLM. The model extracts and structures data, converts charts and tables into CSV files, and organizes qualitative information into a vector database for retrieval-augmented generation. Within minutes, the LLM processes the data and extracts key financial metrics into structured CSV tables.
While this may sound complicated, many platforms already provide similar backend capabilities paired with an easy-to-use frontend interface. All you need to do is drag and drop your data to extract insights. Today's AI document processing solutions parse complex financial statements, identify regulatory red flags, and surface critical insights that might escape even experienced analysts.
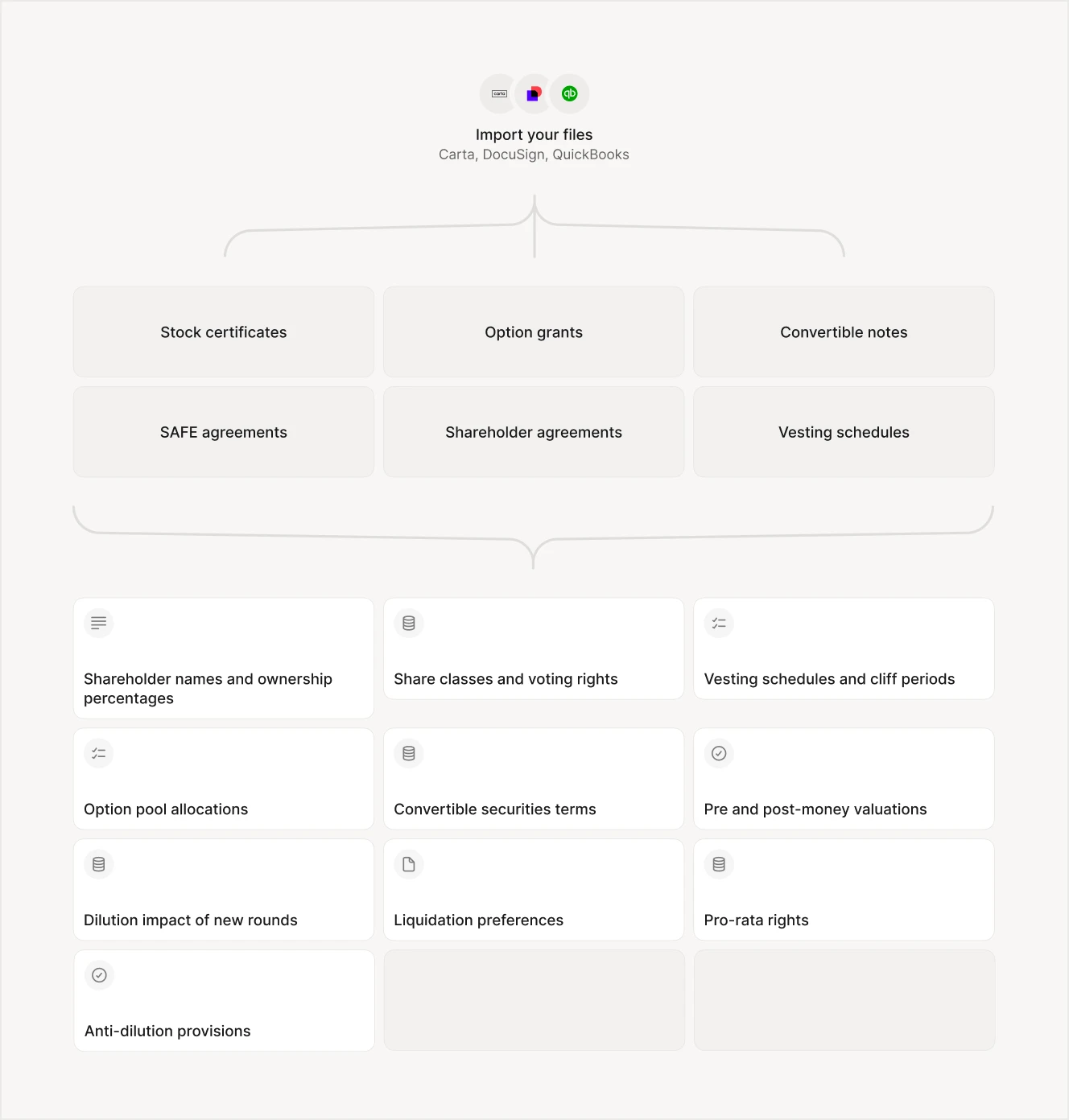
Deloitte's research shows a reduction in processing times for compliance and contract review tasks through AI automation. But the real value lies beyond mere speed. It improves depth and accuracy of the analysis too. Many analysts believe they can look at a CIM and know if they want to move forward almost instantly. But what about the nuanced details buried in footnotes or the subtle inconsistencies across hundreds of pages?
Take this quick self-assessment:
How many hours does your team spend reviewing regulatory filings?
What percentage of red flags do you catch in the first review?
How often do post-deal surprises emerge from missed details in due diligence?
The latest AI platforms for PE/VC firms don’t just scan documents. They can create structured, searchable knowledge bases. Imagine having every contract clause, every financial statement, and every compliance requirement instantly accessible and cross-referenced. This functionality is now readily available out of the box in most specialist AI software.
If you are interested in exploring AI due diligence further, the AI due diligence guide offers many valuable insights.
3. Operational task automation
Back-office operations are consistently identified as one of the most promising areas for business automation with artificial intelligence. A portfolio operations manager can leverage AI tools to flag discrepancies before they escalate into problems, rather than spending time playing Excel detective.
Consider a typical mid-market software company in a PE portfolio. Its finance team might spend 40 hours each month standardizing reports across subsidiaries. With AI tools for data extraction and report automation, that time could be reduced to just 4 hours.
The key lies in starting small. Begin with repetitive, well-defined tasks like:
Customer segmentation analysis
KPI tracking and reporting
Invoice processing and reconciliation
Inventory optimization
Sales forecasting
Monitoring performance and standardizing dashboards is an excellent starting point. Instead of focusing solely on specific metrics, consider generating relevant reports tailored to key areas of your business. Examples include:
Financial Performance Reports: Highlight metrics such as cash flow dynamics, profit margins, and revenue growth trends.
Customer Acquisition Reports: Include metrics like customer acquisition costs (CAC), conversion rates, and lifetime value (LTV).
Operational Efficiency Reports: Focus on supply chain efficiency, inventory turnover rates, and delivery times.
Team Productivity Reports: Track metrics such as employee performance, project completion rates, and resource utilization.
Market Analysis Reports: Include metrics related to market share, competitive positioning, and brand sentiment.
Remember: automation isn't about replacing human judgment—it's about freeing up your team to exercise it more often. When routine tasks like reporting run on autopilot, your professionals can focus on what machines can't do: building relationships, spotting strategic opportunities, and making the complex decisions that drive real value creation.
4. Competitive intelligence and market analysis
Many firms treat competitive intelligence as something to address later—important, but never quite urgent enough to make it a priority. It often gets left on the back burner, with conversations like, “We still have that spreadsheet John compiled, right? Wait… that was last year?” slipping into the background as day-to-day demands take over. The assumption is that there will always be time to revisit it, but that time rarely comes.
As a result, companies often find themselves unprepared when a competitor unexpectedly secures a key client, launches a disruptive product, or gains ground in a supposedly secure market. Treating competitive intelligence as an ongoing, strategic effort is essential to staying ahead—not just reacting to what’s already happened.
The good news is that you can automate these efforts with AI.
Instead of drowning in quarterly reports and industry newsletters, AI platforms like V7 Go can now digest this information in real-time, perform web searches to gather information from hundreds of sources, and spot patterns that human analysts might miss.
AI can sift through customer reviews, social media posts, Reddit, and news articles to gauge public perception of products, services, companies, or their leadership. It can even detect shifts in tone over time, revealing early signs of market backlash or emerging opportunities.
For those looking to enhance their competitive intelligence, exploring IDP use cases and AI sentiment analysis frameworks can help you uncover valuable insights.
5. Daily task quick wins
While senior partners debate AI's strategic value, a quiet revolution is already underway in PE/VC firms. Junior associates are finding organic ways to integrate AI into their daily workflows.
A common scenario is a junior private equity professional using AI tools like ChatGPT or a GPT wrapper to save time on everyday tasks. They might use them to quickly summarize emails or handle web searches that would normally take hours. For instance, instead of spending half a day digging through data, they can let AI handle the heavy lifting and save a couple of hours in the process.
These small efficiency gains compound quickly. More importantly, they allow employees to develop AI literacy naturally, without disrupting existing processes or requiring significant infrastructure changes. Users discover practical applications that fit their specific needs, leading to bottom-up identification of high-value automation opportunities.
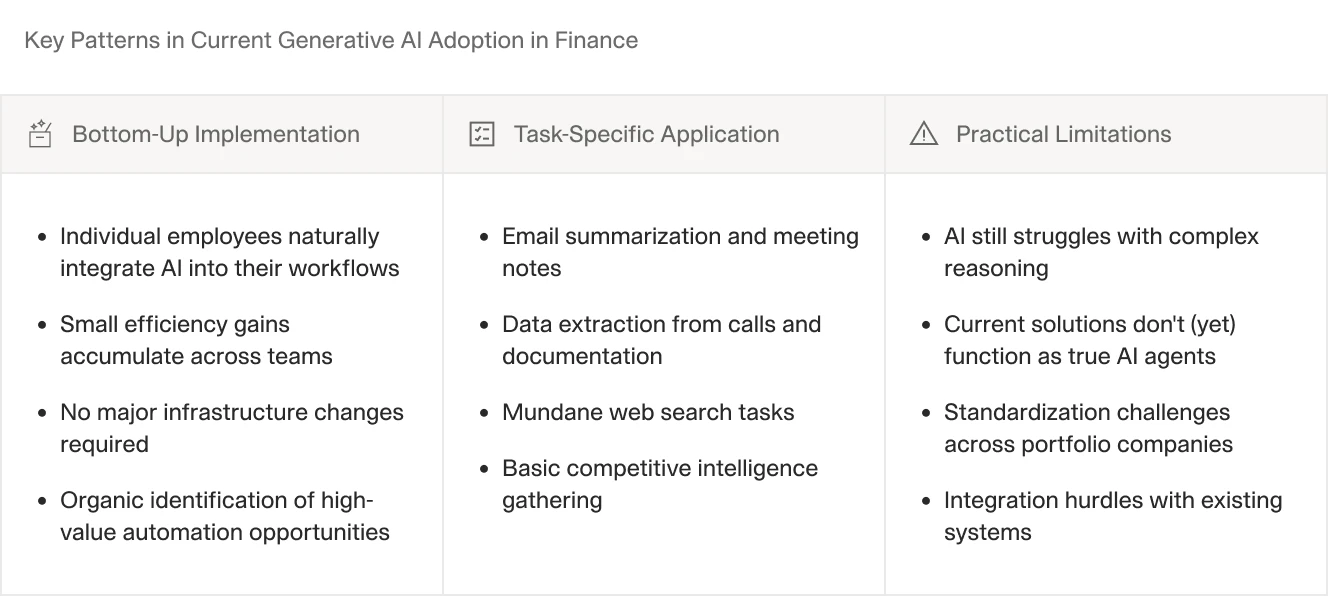
However, there’s a significant risk that comes with using third-party tools like ChatGPT for sensitive tasks. These solutions operate as external platforms, and users often have limited visibility into what happens with the data they input. This lack of transparency can pose serious security concerns for firms handling confidential information like deal terms, financial projections, or proprietary strategies.
For PE/VC firms, secure alternatives with custom deployment options are a better fit. On-premises, location specific, or private cloud-based AI solutions allow companies to retain better control over their data while reaping the efficiency benefits of AI. These platforms enable tasks like email summarization, data extraction, and competitive intelligence gathering, but with the added assurance that sensitive information remains private.
Curious about how to bring generative AI into your PE/VC firm without shooting yourself in the foot? Keep reading for tips and things to watch out for.
AI implementation best practices in PE/VC
Implementing AI in venture capital and private equity isn't a smooth sail—challenges lurk beneath the surface, ready to derail even the most promising initiatives. The sheer volume of documents that firms handle daily can overwhelm traditional AI systems. From confidential information memorandums and financial statements to legal contracts and compliance documents, each requires different processing approaches and specialized understanding.
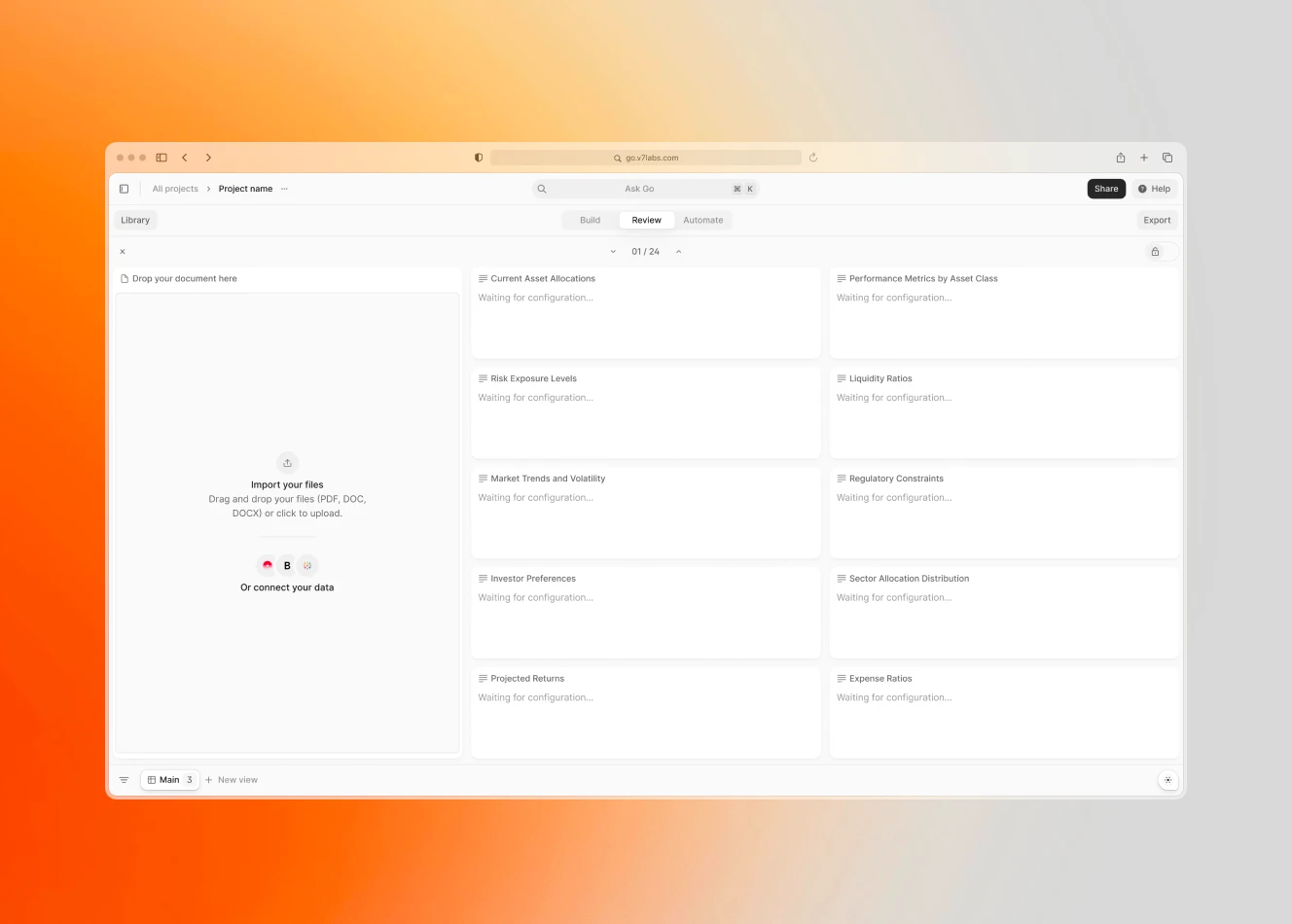
Document complexity in investment workflows
Investment firms face unique challenges when processing diverse document types. A typical deal involves analyzing hundreds of pages across various formats: pitch decks in PowerPoint, financial models in Excel, legal documents in PDF, and increasingly, alternative data sources like customer feedback or social media sentiment. Modern multimodal AI platforms like V7 Go can handle this complexity and they can process everything from earnings calls to financial statements while maintaining the context crucial for investment decisions.
Secure processing of sensitive financial data
PE/VC firms operate under strict confidentiality requirements, making data security paramount. Traditional cloud-based AI solutions often raise concerns about data privacy and regulatory compliance, while building internal solutions requires substantial investment in security infrastructure. Advanced platforms now offer enterprise-grade security through private deployment options, ensuring sensitive financial data never leaves firm control while maintaining SOC2 and GDPR compliance.
Integration with investment workflows
The challenge of integrating new technology into established investment processes often leads to resistance from investment professionals who prioritize deal execution over learning new systems. Gen AI solutions address this through flexible architectures that seamlessly integrate with existing tools—from CRM systems to deal management platforms—allowing teams to maintain their preferred workflows while automating document processing in the background.
Quality assurance in investment decisions
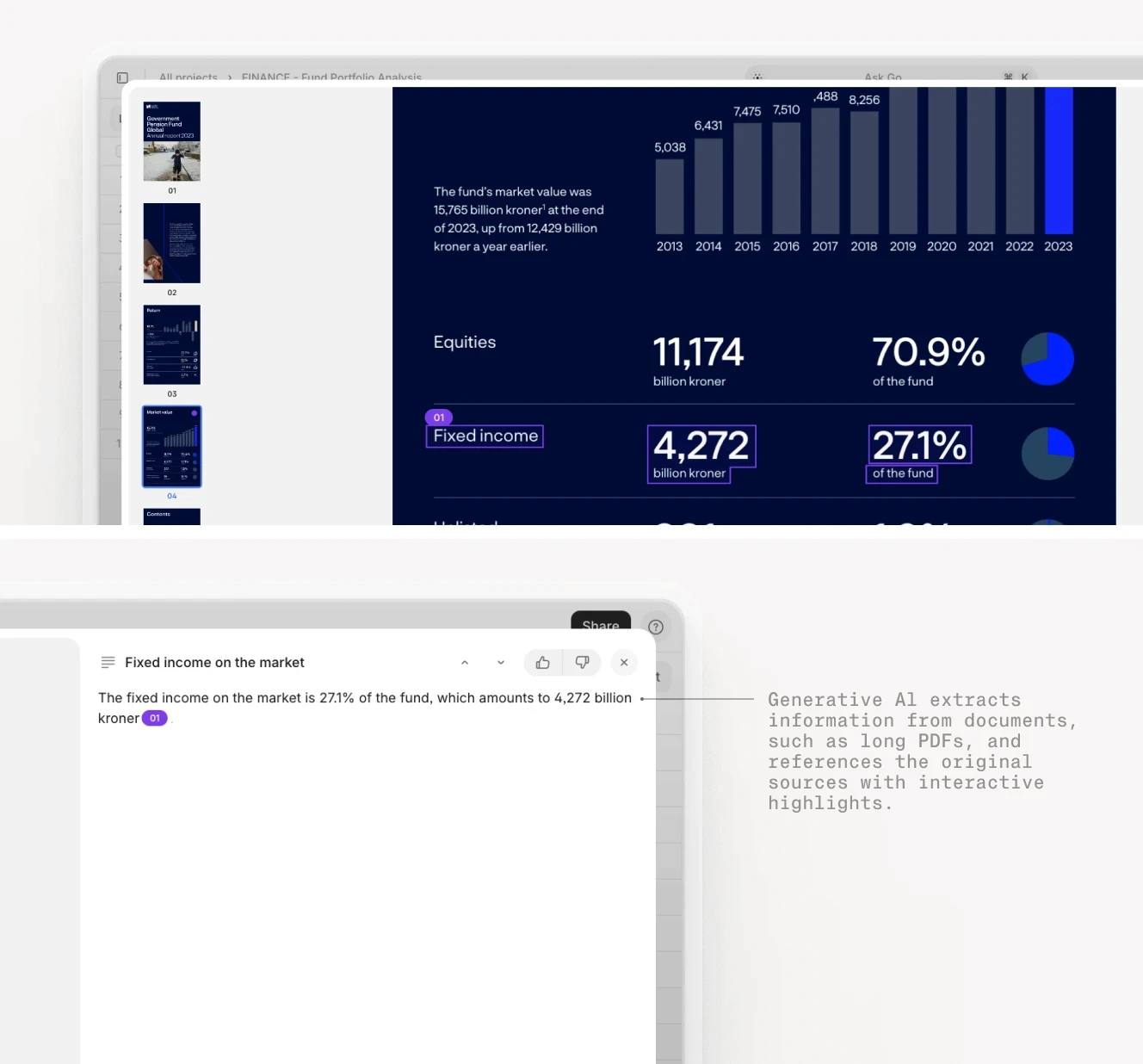
In private equity and venture capital, accuracy is non-negotiable. Investment decisions based on flawed data analysis can cost millions. The latest generation of AI tools provides comprehensive verification capabilities, allowing investment professionals to trace extracted data back to source documents and understand the reasoning behind conclusions—critical features for investment committee presentations and due diligence documentation.
Domain knowledge and customization
Generic AI solutions often stumble over specialized investment terminology and metrics. Understanding concepts like EBITDA adjustments, cap tables, or industry-specific KPIs requires specialized training. Advanced platforms now enable firms to incorporate their investment expertise through customizable knowledge bases, ensuring accurate handling of nuanced industry-specific metrics and terminology.
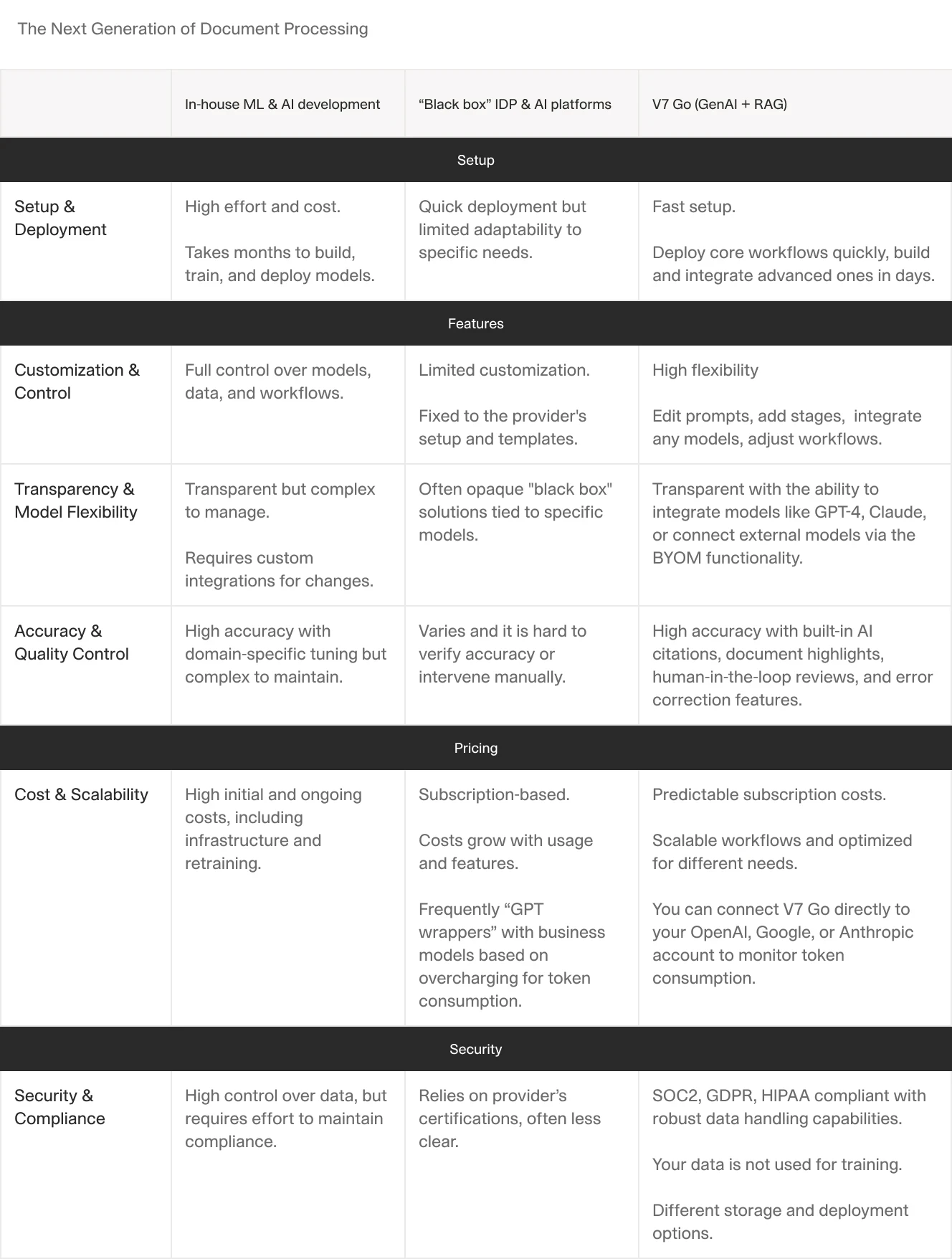
Conclusion
The evolution of AI platforms has made implementation challenges in PE/VC more manageable. With comprehensive security features, seamless workflow integration, and domain-specific customization options, firms can now enhance their investment processes while maintaining the rigor and confidentiality required in private markets. This technological advancement allows investment professionals to focus on what matters most: making better investment decisions faster.
The window for early adoption is closing. The question isn't whether to implement AI—it's how quickly you can do it without disrupting your existing operations. Thankfully, many solutions can be implemented without massive infrastructure changes or lengthy training periods.
Don't let analysis paralysis cost you your competitive edge. The future of investment success belongs to those who act now, not those who wait for perfect certainty. After all, in venture capital and private equity, timing isn't just important—it's everything.
For those looking to get started, book a demo and explore our gen AI toolkit built for investment firms.