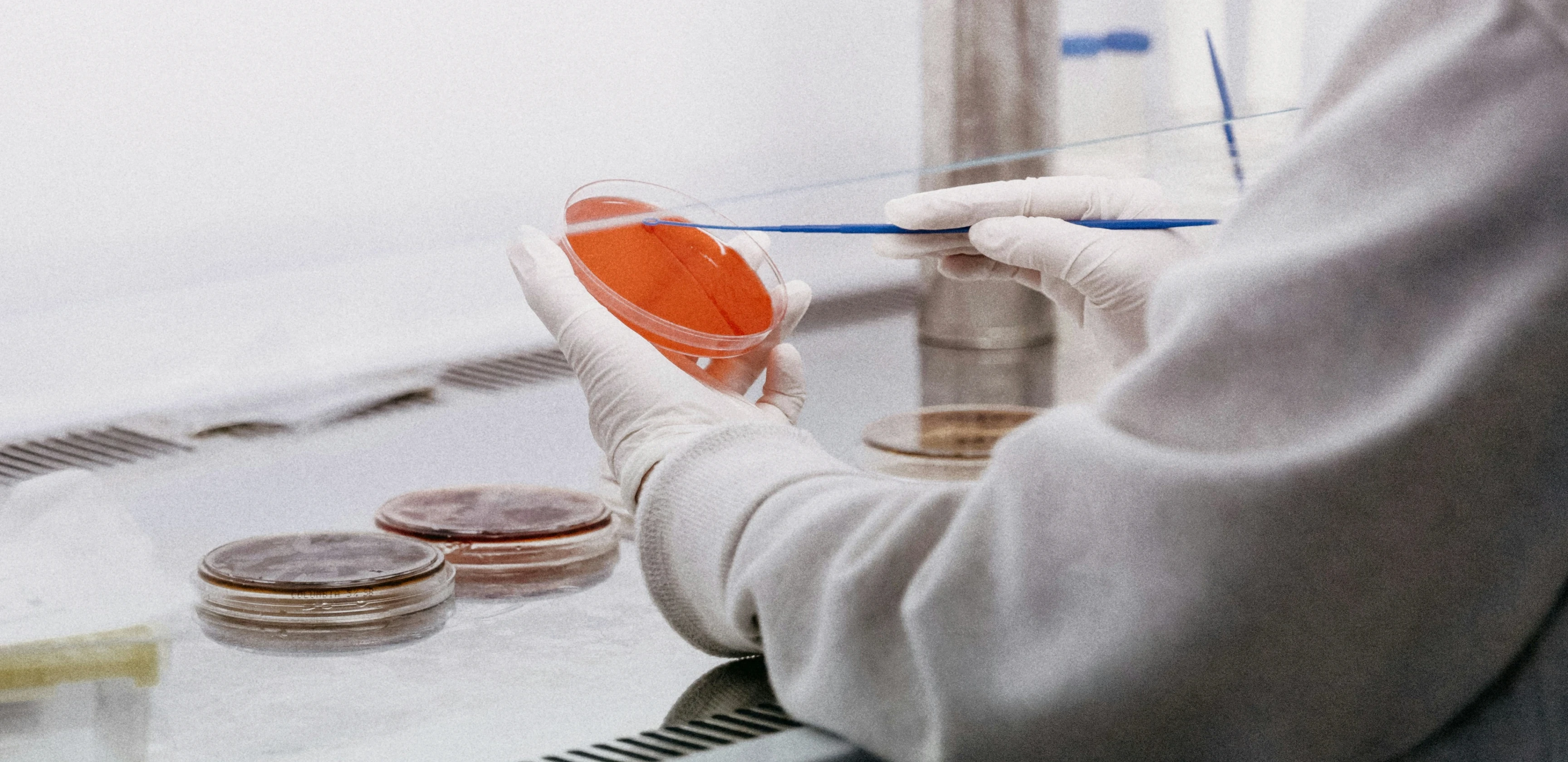
Franklin.ai helps pathologists diagnose with confidence and report faster.
Industry
Healthcare
Use Case
Digital pathology
Company size
11-50
Headquarters
Haymarket, Australia
Data labelling
Discover our solutions
About
Franklin.ai‘s goal is to address a global shortage of pathology specialists by providing pathologists with a digital assistant that uses state-of-the-art technology to enhance diagnostic accuracy and efficiency.
This Australian-based joint venture between Harrison.ai and Sonic Healthcare will provide pathologists with AI solutions that act as a second pair of eyes. These solutions have the potential to improve diagnostic accuracy and increase workflow efficiency, quality of care, and outcomes.
The challenge
Franklin.ai’s mission is to meet a worldwide shortage of pathologists by building AI digital pathology models capable of performing diagnostic tasks with increased accuracy and efficiency.
To build highly accurate AI models, Franklin.ai needs to create training data from thousands of specialist Whole Slide Image (WSI) files, annotating anomalies within tissue cells on a pixel-perfect level.
However, without the right tools managing, storing, and annotating these enormous data files would divert significant engineering time and resources.
The solution
Franklin.ai uses V7 Darwin to train segmentation models that identify anomalies in tissue cell WSIs to a near-perfect degree of accuracy, helping pathologists make confident diagnoses worldwide.
Collaboration between V7 and Franklin.ai to develop custom tiling code significantly decreased the storage costs involved in the data annotation process and facilitated more efficient collaborations between teams.
Furthermore, V7’s direct integration with Franklin.ai’s private AWS S3 storage bucket has enabled them to annotate data with a global team of users without patient data ever leaving their private storage bucket.
The results
Using V7, Franklin.ai has deployed its first product to market, helping pathologists save time by using AI to analyze medical data.
By streamlining data management and annotation with V7, this team decreased the time needed to create batches of 10,000 images to their training data pipeline from 10 hours to 30 mins.
Training data needs
Creating pixel-perfect annotations for WSI files involves sharing enormous files between expert medical labelers.
Manually managing this sharing would divert significant engineering resources to downloading, uploading, and storing files resulting in poor-quality and costly training data.
To solve this, this team needed a platform that would integrate with their existing AWS storage, and enable them to keep all data within their own S3 bucket.
So, this team needed a training data platform capable of supporting high-quality annotations, hosting large amounts of data, and facilitating easy collaboration between annotation experts.
Building intelligent AI to assist pathologists requires training machine learning models to identify abnormalities in cell tissues from WSIs.
Franklin.ai chose V7 Darwin to create this training data after careful consideration.
Key factors in their decision were our stringent data security policies designed to help build compliant medical AI products and V7’s support in building customer tiling code to overcome the challenges of large file sizes common with medical specialist WSI data.
Franklin.ai was attracted by the ability to integrate V7 Darwin with their existing AWS S3 storage, saving time-sharing files. Our accessible UI also spared the diversion of engineering resources needed to develop a custom front end.