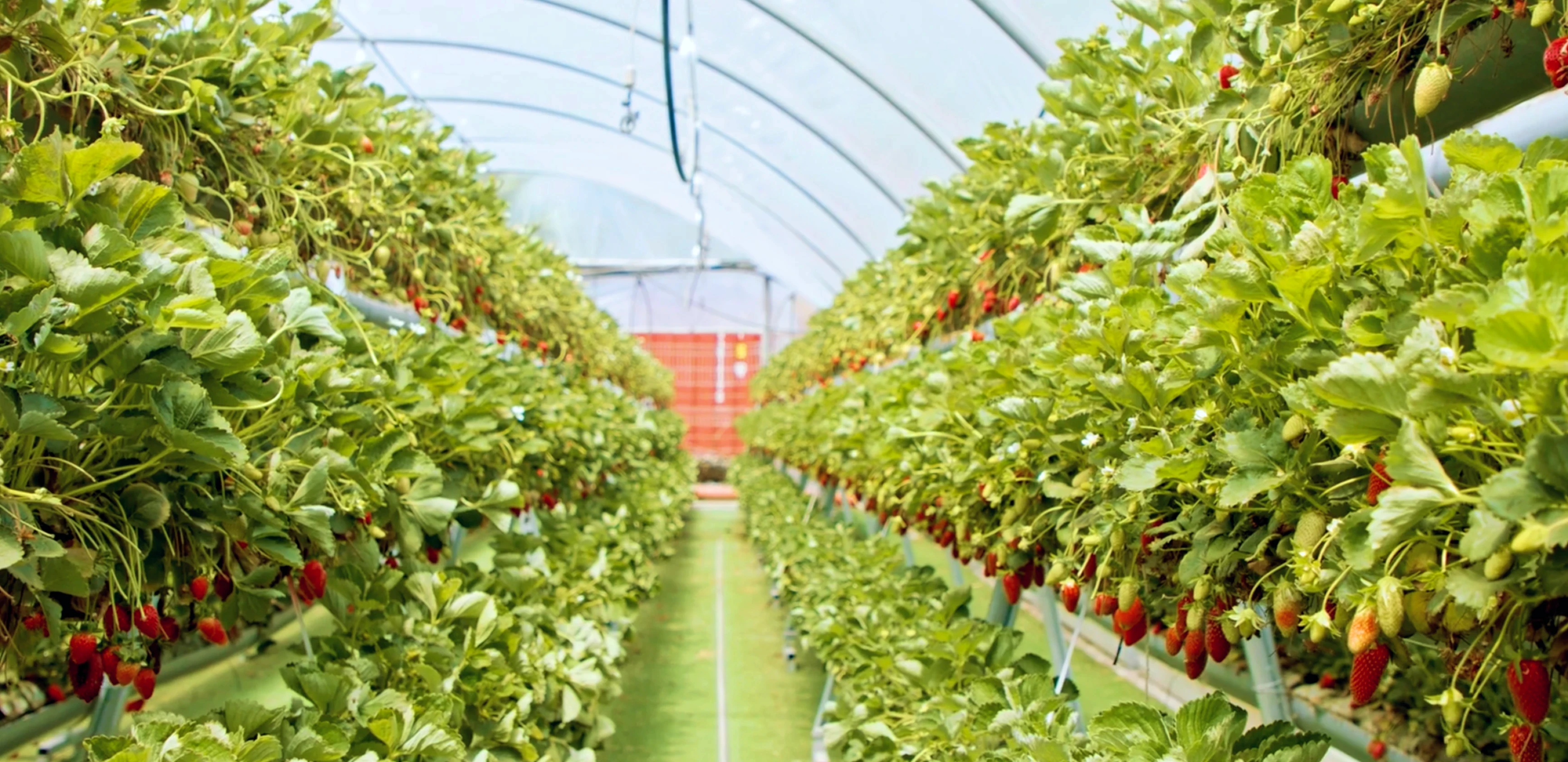
Forecasting strawberry yields using computer vision
Industry
Agriculture
Use Case
Yield Estimation
Company size
2,000+ employees
Headquarters
Riseholme Campus
Data labelling
Discover our solutions
About
LIAT’s mission is the development of technologies that add value or solve challenges across the food chain. In this project, they focused on the horticulture sector—starting with a small strawberry farm located near Riseholme Campus. The team uses vehicle-mounted cameras to identify and count individual fruits, estimate their weight and maturity state. This data is used to develop AI algorithms for strawberry yield forecasting.
The challenge
Predicting the timing and yield of strawberries is critical but extremely difficult to do accurately. More than 40% of horticulture production costs are spent on picking labor, and much of the rest belongs to incorrect yield predictions. LIAT's team is using computer vision and robotics to create a system to predict strawberries' readiness and decrease the uncertainty of those forecasts by monitoring the crop responses in real-time.
The solution
LIAT collects image and video data from hand, vehicle or robot-mounted cameras, and uses V7 to label, identify, and track every strawberry in a greenhouse through semi-automated video annotation. They team leverages annotated data to train ML models and build robust, AI-powered yield forecasting systems able to predict yield six weeks ahead of existing systems.
The results
Thanks to the successful implementation of their computer vision models, LIAT's team has improved yield prediction accuracy and prediction time from 3 to 5 weeks. This means higher profit for the farmers and reduced supply chain friction and crop waste.
Apart from that, using V7's auto-annotation tool, the team managed to improve the model's accuracy going from 85% to 95% and achieving industry-leading performance tested on on real agricultural data.
Training data needs
LIAT's team is working with large datasets of strawberries (and other soft berries) captured using a vehicle or robot-mounted cameras. Data is annotated using V7's labeling tools such as bounding boxes, polygons, and directional vectors.
Labeled images are then used to train AI models that have already proven to achieve state-of-art performance on real agricultural data.
LIAT's team has decided to outsource their data annotation to V7's labeling partners to speed up the process and focus on the most critical aspects of their project.
Testimonial
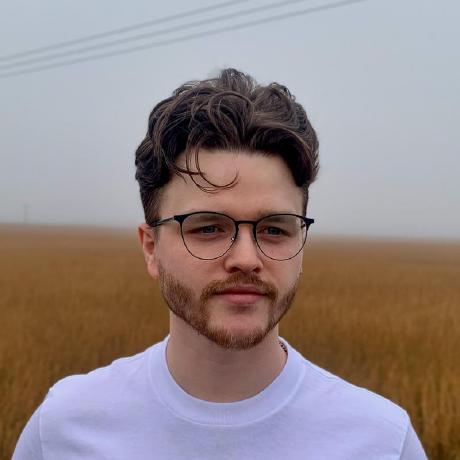
Raymond Tunstill
PhD Researcher, CTO of FruitCast
Why V7?
The LIAT's team found V7 when looking for an alternative to Labelbox.
After giving it a try, they were pleasantly surprised to see the model accuracy going from 85% to 95% when labeling data on V7.
The team has also come to appreciate V7's advanced dataset management features, data versioning capabilities, and UX-friendly design that makes the whole process easier and more efficient.