Knowledge work automation
7 min read
—
Jan 16, 2025
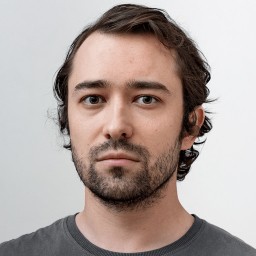
Content Creator
The Rise of Work AI: Will Knowledge Work Be Fully Automated by the 2030s?
Work AI adoption has nearly doubled in recent months, with 75% of knowledge workers now using AI tools in their daily operations. It's no longer a matter of "what if" or "when." The reality is that AI technology has become firmly embedded in everyday knowledge-intensive tasks, whether or not management approves.
So, the trillion-dollar question is: will AI fully replace white-collar jobs and knowledge workers within the next few years?
Roles most affected include paralegals sifting through case law, financial analysts processing market data, and insurance underwriters evaluating risk factors—and the impact is overwhelmingly positive. Rather than jobs being eliminated, we're seeing significant productivity enhancements, particularly in back-office operations, where AI is expected to boost employee productivity by 40%.
In this article, we'll cover:
What is work AI and what impact does it have on business operations?
Differences between generative AI and other knowledge work technologies
Implementation best practices and frameworks
Overview and comparison of the best knowledge work AI platforms
Before we dive in, let's explain what we exactly mean by knowledge work:
Knowledge work involves tasks that require analyzing information, making complex decisions, and creating new insights—activities traditionally performed by professionals like analysts, lawyers, and researchers.
What is work AI and why should I care?
Work AI represents a new category of software that combines Large Language Models (LLMs) with specialized workflows to automate complex knowledge work. Unlike traditional automation tools that handle repetitive tasks, knowledge work AI tackles nuanced intellectual work—from legal document analysis to financial modeling.
At its core, work AI consists of three essential components:
A foundation of Large Language Models (LLMs) that understand context and generate human-like responses
Domain-specific training or Retrieval-Augmented Generation (RAG) tech that adapts these models to particular industries and use cases
Workflow automation capabilities that coordinate multiple AI operations into coherent business processes
Here is what this typically looks like:
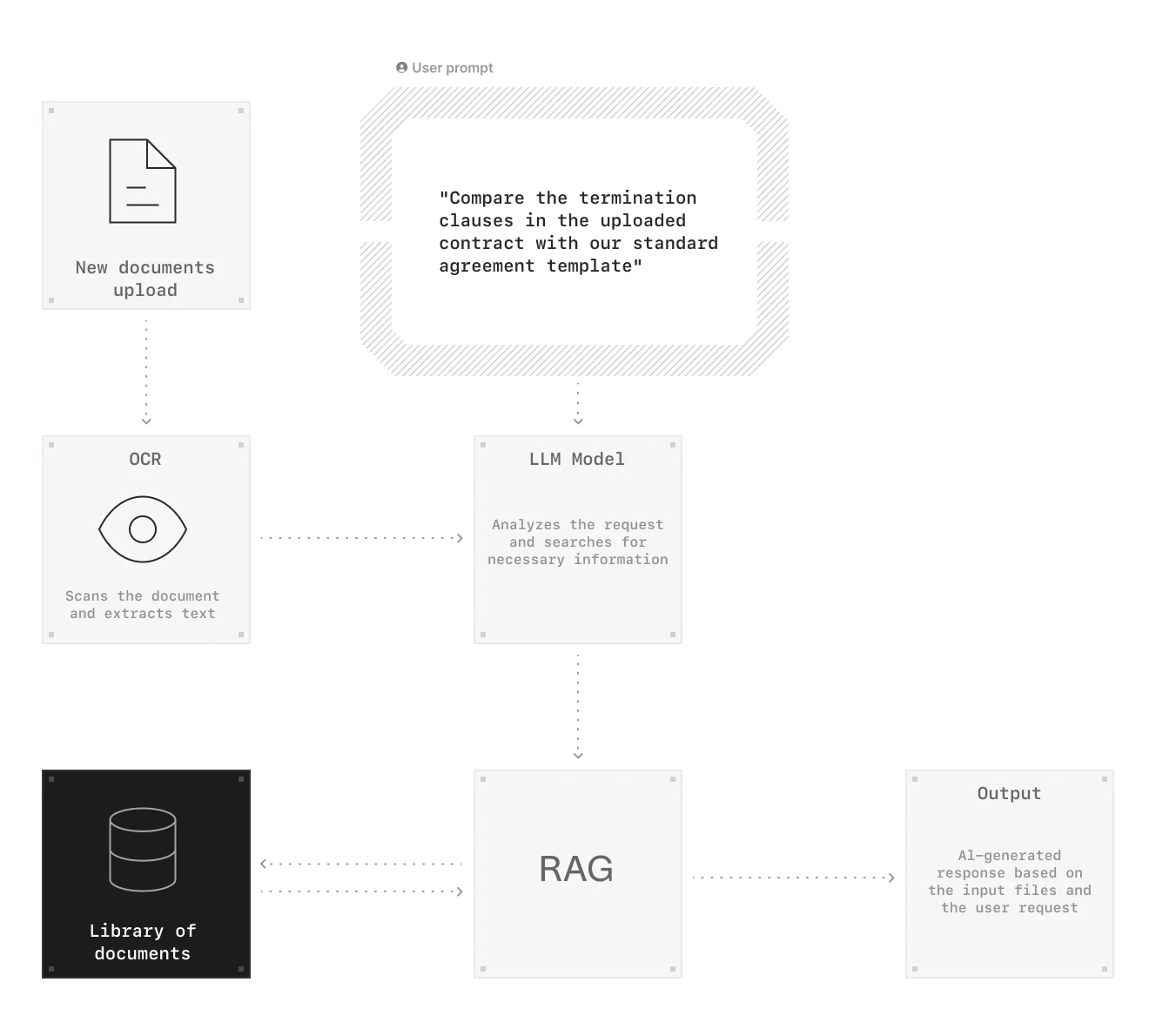
Unlike traditional Optical Character Recognition (OCR) software that simply lifts text from documents, a knowledge work AI platform can understand document structure and context. These solutions can tell the difference between a liability clause in a contract and a similar-looking paragraph in a marketing brief—and can automatically trigger appropriate workflows, such as routing contracts for legal review or forwarding marketing content to the creative team for approval.
First, LLMs go beyond basic text recognition to provide true comprehension and reasoning when extracting and understanding information.
Secondly, RAG enables the AI to tap into your organization’s internal knowledge bases, such as proprietary databases, document repositories, or CRM systems. Instead of relying solely on its pre-trained data, the AI retrieves relevant and up-to-date information from these custom sources.
Finally, the workflow automation capabilities of work AI ensure tasks follow a specific course of action, whether based on predefined logic, integration with third-party apps, or AI-driven decision-making. For example, after processing a contract, the platform can update a CRM, notify relevant teams, or autonomously decide on the next steps based on context.
By combining LLMs, RAG, and automation, work AI enables companies to:
Enhance productivity: Automate complex tasks such as drafting reports, analyzing contracts, and processing large datasets, allowing employees to focus on higher-value activities.
Improve accuracy: Leverage AI’s ability to process and cross-reference information, reducing errors in critical tasks like financial modeling, compliance checks, and legal research.
Scale expertise: Enable organizations to replicate expert-level decision-making across various teams and geographies without needing an expert present for every process.
Accelerate decision-making: Provide instant insights and recommendations by synthesizing large amounts of information in real time, helping leaders make informed decisions faster.
Reduce operational costs: Streamline workflows by minimizing manual intervention, cutting down on overhead and inefficiencies in knowledge-intensive operations.
But time is running out for organizations to adopt these tools. Leading firms are already deploying work AI to process thousands of documents daily. For example, over 50% of top insurance companies are already piloting LLM solutions for sales and underwriting.
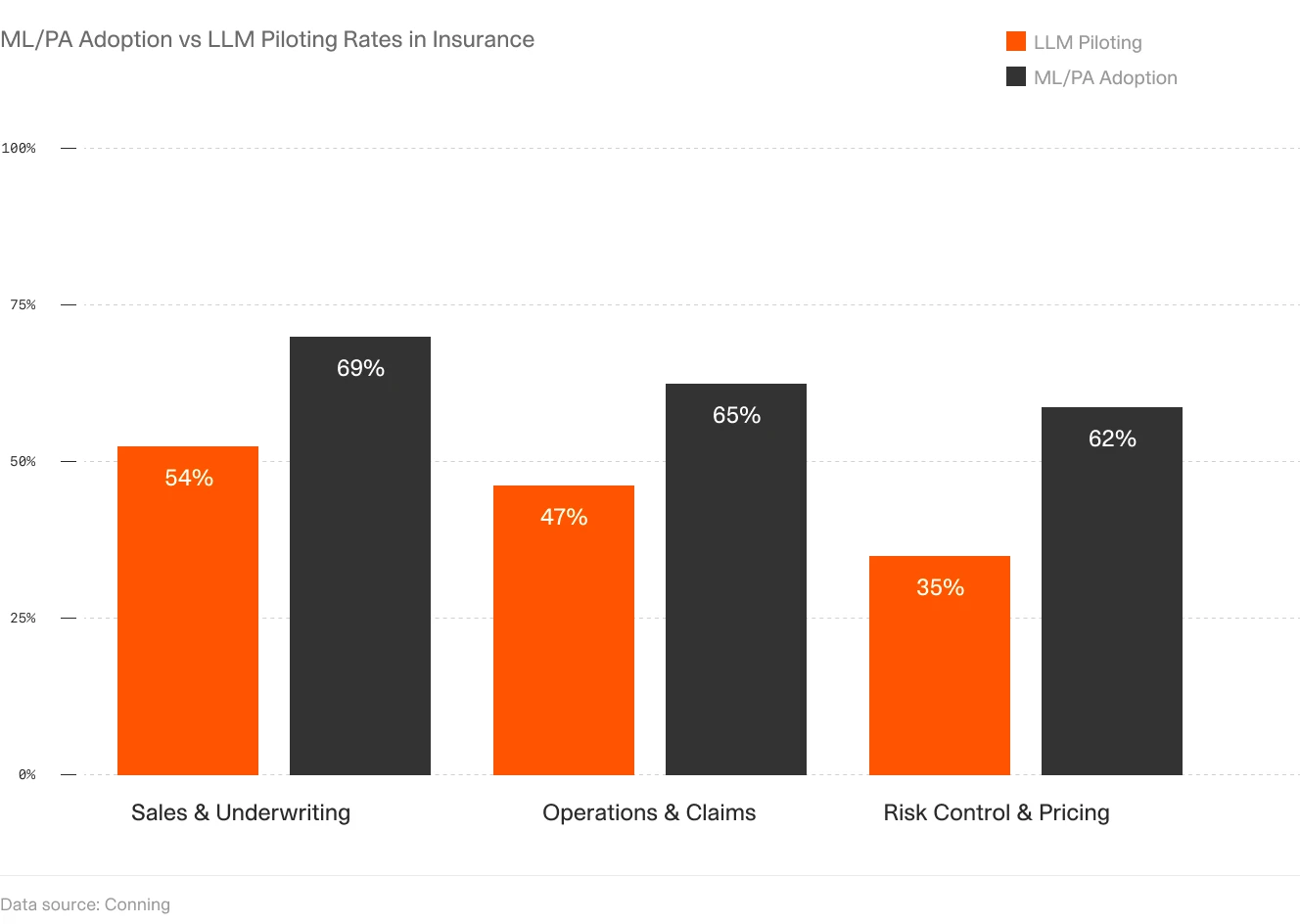
Some businesses are reluctant to implement yet another technology. To be fair, things can be sluggishly slow in corporate settings, and for many companies, Robotic Process Automation (RPA) and Intelligent Document Processing (IDP) are still the core “innovative tech of tomorrow”—often stuck in the early stages of adoption.
However, this hesitation is like trying to reverse engineer a steam engine while your competitors are already building bullet trains.
How work AI differs from RPA, IDP, and ChatGPT
A few years ago, automating knowledge work meant hiring workers on platforms like Mechanical Turk to manually process everything from damaged receipts to complex documents. Even with AI, organizations needed massive training datasets and specialized workers with domain expertise to build custom models. Work AI represents a paradigm shift in how we approach task automation for knowledge work.
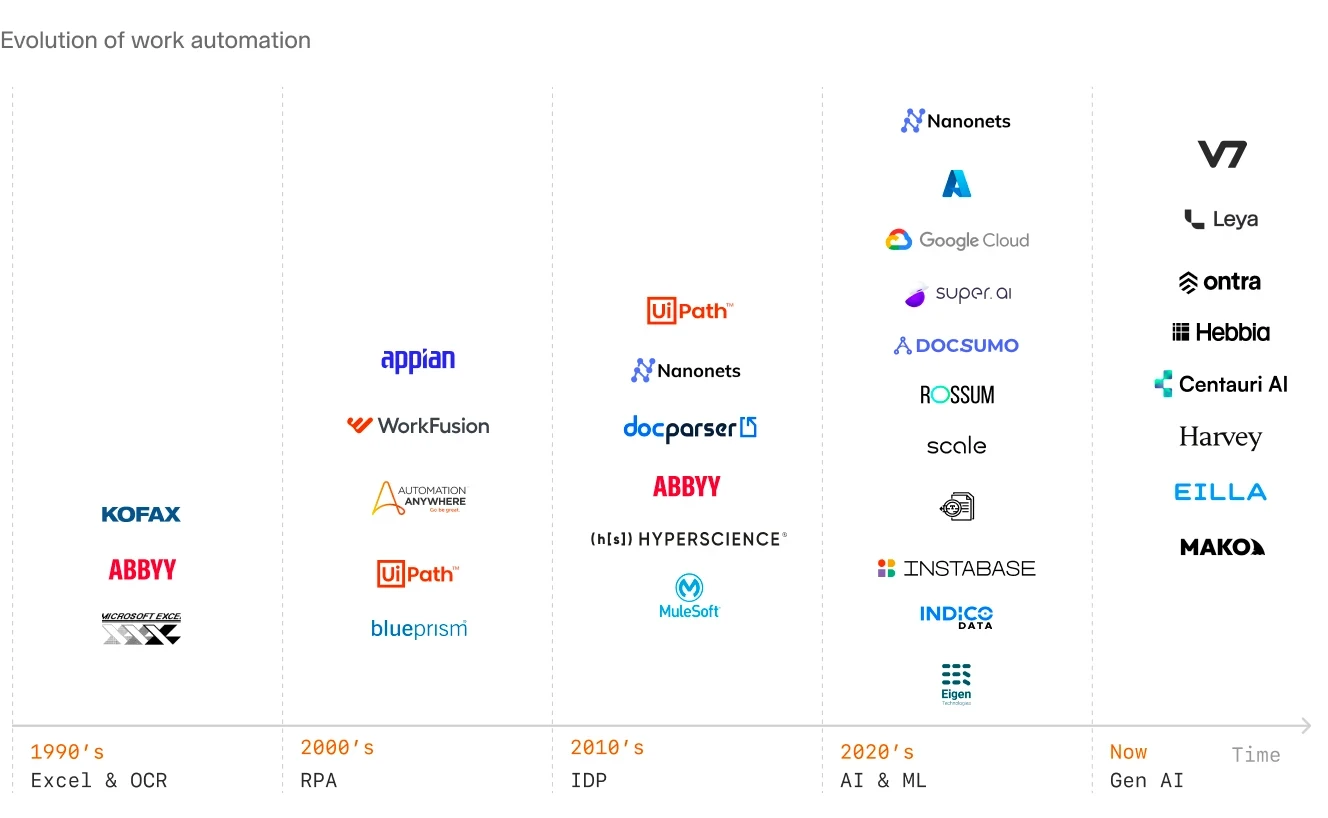
As mentioned before, there are already whole categories of software for knowledge work. It is true that RPA might help you sort documents into folders based on predefined rules. But, work AI can take it many steps further. You can use a solution like V7 Go to read, understand, extract relevant information, make decisions, and even draft responses—all while learning and improving from each interaction.
Knowledge work AI platforms powered by generative AI combine the strengths of RPA, IDP, and ChatGPT while overcoming their limitations:
RPA: Work AI automates workflows but replaces rigid, rule-based logic with adaptive, goal-driven processes powered by Large Language Models (LLMs). It handles variability across tasks and scales beyond repetitive processes.
IDP: Work AI integrates document processing capabilities but adds reasoning and context-awareness, enabling it to analyze documents for meaning, implications, and policy-driven actions rather than just extracting data.
ChatGPT: Work AI incorporates conversational abilities while solving the orchestration challenge. It seamlessly integrates into enterprise systems, retrieves internal knowledge with RAG, and coordinates workflows across multiple tools and APIs.
Unlike any of these technologies considered individually, work AI provides a unified solution for automating complex, reasoning-intensive workflows while remaining flexible, scalable, and deeply integrated into enterprise environments.
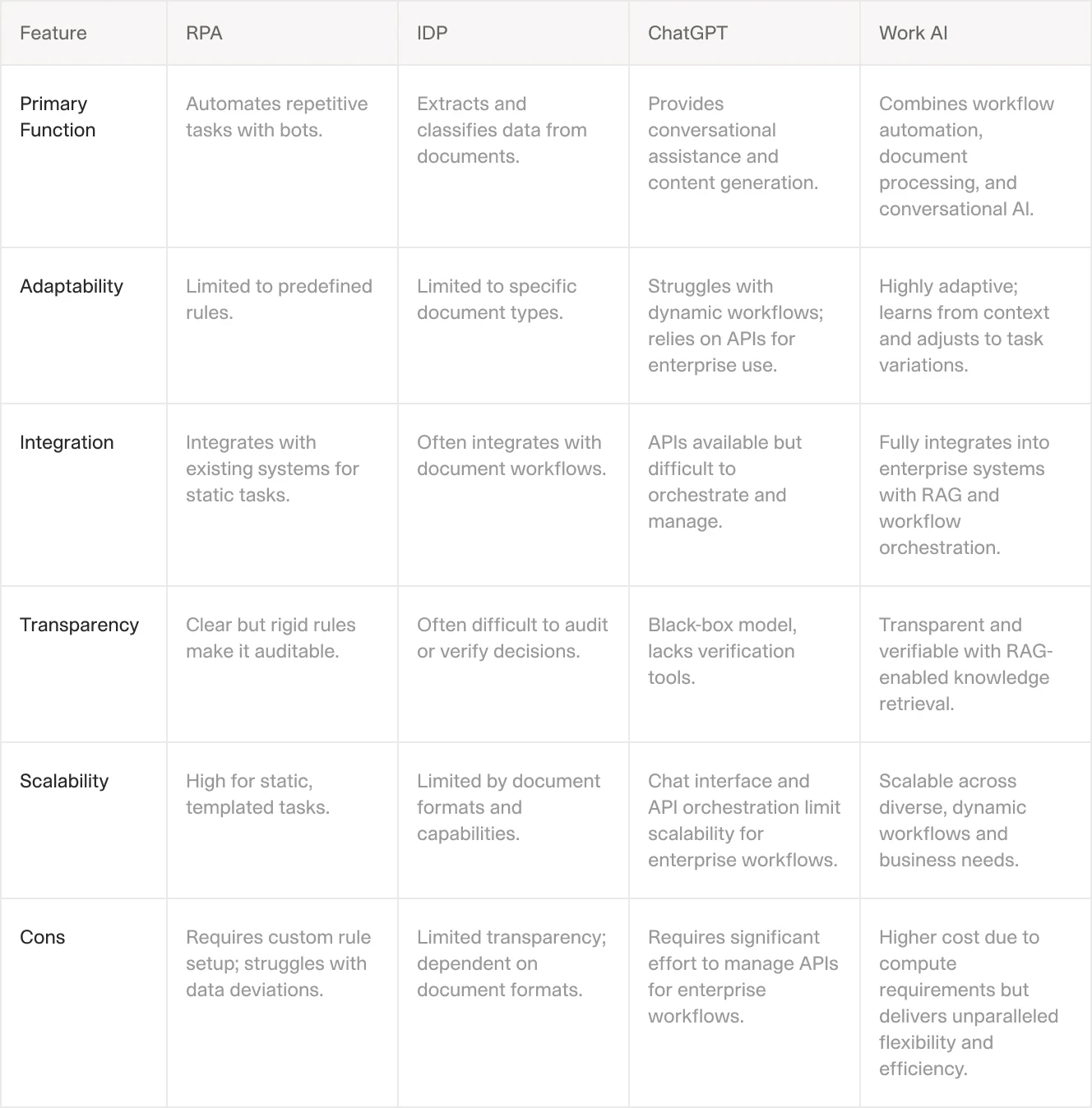
The choice seems obvious, right?
Well—
Let's be honest—while the benefits are compelling, implementing AI isn't as straightforward as vendors might suggest. Organizations need a structured approach to evaluate and deploy these solutions effectively.
Work AI implementation
Implementing work AI requires careful planning and a structured approach. While the benefits are compelling, success depends on thorough preparation across multiple dimensions.
Assessment phase
Begin by examining your organization's readiness across three critical areas. Your existing tech stack's compatibility with work AI solutions is paramount—integration challenges with legacy systems can derail even the most promising implementations. Data readiness forms the second pillar, as different AI systems have varying requirements for data structure and format. Finally, evaluate your team's technical capabilities to determine if additional training or hiring will be necessary.
Modern work AI platforms offer intuitive interfaces that simplify workflow creation and management. This can significantly reduce the need for extensive user training.
Financial considerations should be addressed early in the assessment phase. Implementation costs extend beyond software licensing to include infrastructure upgrades, data migration, and potential downtime during transition periods. For mid-sized companies, a comprehensive work AI rollout—including training, integration, and optimization—typically requires a budget of $100,000-500,000.
Deployment and change management
Perhaps most crucial is the human element of implementation. Technical excellence alone won't guarantee success. The deployment phase requires a dual focus on technical implementation and organizational adaptation.
Begin with a pilot program in a specific department or workflow to validate assumptions and identify potential challenges. This approach allows for adjustments before broader deployment while building internal champions for the technology.
A comprehensive change management strategy should address training needs, communicate benefits clearly, and ensure employees view AI workflows as an empowering tool rather than a threat to their roles.
Measuring success
The true value of work AI lies in productivity enhancement rather than simple cost reduction. Success metrics should focus on how AI augments human capabilities—enabling employees to focus on creative and interpersonal aspects while automating clerical tasks. This symbiotic relationship between AI and knowledge workers often leads to revenue growth that outweighs implementation costs.
A well-implemented work AI system typically delivers a 30% productivity increase, with some workflows achieving even greater efficiency. Financial reporting and logistics documentation, for instance, can see productivity gains of 300-800% through comprehensive automation.
Your evaluation framework should prioritize concrete outcomes, including time saved per knowledge worker, error reduction rates, process completion speed, and employee satisfaction scores. Consider also the broader implications: data privacy requirements, scalability needs, vendor stability, API flexibility, and customization capabilities.
This strategic approach to work AI implementation, combining technical assessment, clear success metrics, and thoughtful change management, provides the foundation for successful digital transformation.
Is work AI relevant to my industry at all?
ou might be wondering whether “work AI” is just more tech industry hype or if it can actually make a meaningful difference in your industry. As with most things, the truth lies somewhere in the middle. While AI's impact today varies widely across sectors, there are fascinating examples of how this technology is reshaping traditional business processes.
Insurance
Modern AI underwriting platforms analyze comprehensive risk profiles within minutes, while automated claims systems identify fraud patterns and validate documentation with remarkable precision. A major insurance provider has reported significant improvements in claims processing efficiency through AI implementation. For deeper insights into AI's role in insurance, check out AI trends in insurance.
Real estate
Real estate automation focuses primarily on commercial property assessment and documentation. AI platforms streamline key processes including lease abstraction, investment analysis, and property valuation by simultaneously evaluating multiple data points - from market comparables to maintenance records. This allows commercial real estate professionals to focus on deal strategy and client relationships rather than manual document review. Tools like V7 Go can automate lease analysis, extract key terms and conditions from complex agreements, and standardize property documentation across large portfolios. For commercial property managers and investors, this means faster deal evaluation, reduced due diligence time, and more accurate AI risk evaluation for asset management.
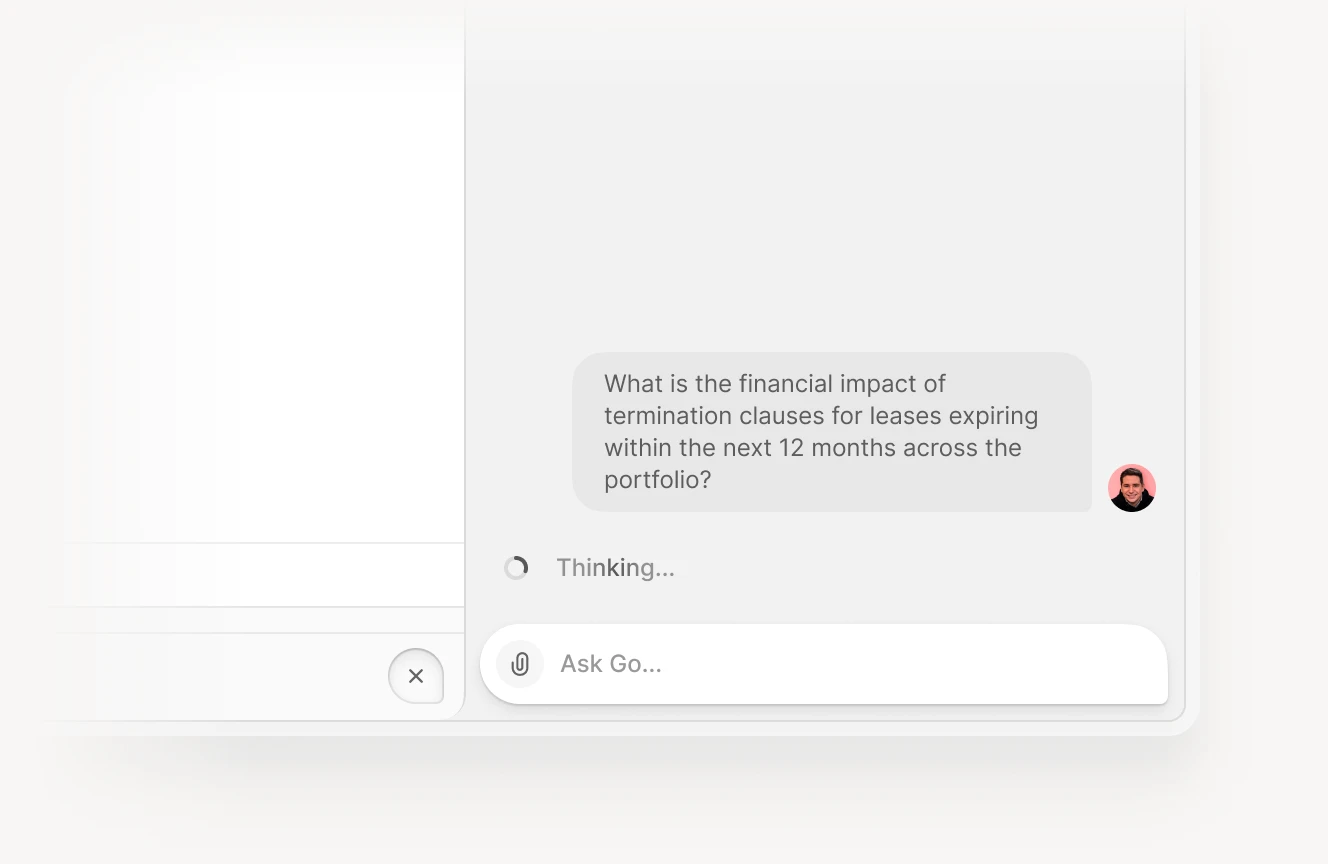
For a comprehensive overview of these innovations, explore AI in real estate use cases.
Finance
Perhaps the most striking impact is in corporate due diligence. AI systems now process vast document repositories during M&A transactions, identifying potential risks and patterns across contracts, financial records, and compliance documentation. One private equity firm's implementation shortened their initial due diligence window from weeks to 48 hours, while uncovering three previously undetected material risks. You can learn more about the capabilities of AI due diligence tools in this guide.
These platforms integrate natural language processing with specialized industry expertise to deliver consistent accuracy during document reviews—a critical edge over human reviewers who might overlook key details buried deep within complex documents. For example, a clause on page 847 of a contract can be easily missed. This level of reliability is especially valuable in analyzing extensive legal and financial documentation.
Best work AI platforms
You probably won't believe this, but the work AI platform landscape is far more nuanced than the typical "AI solutions" flooding the market. Let me show you why.
V7 Go simplifies complex document processing and workflow tasks for regulated industries such as finance, legal, and insurance. Its advanced document parsing, visual grounding, and enterprise-grade security features allow organizations to meet strict compliance requirements while improving operational efficiency. With SOC 2 Type II certification and robust data governance controls, V7 Go empowers businesses to automate sensitive workflows confidently. The platform's capability to accurately process intricate documents—ranging from prospectuses to policy documents—combined with seamless system integration, makes it indispensable for sectors where precision and auditability are critical.
To learn more about the platform, visit V7 Go and book a demo with the team.
Other noteworthy platforms include:
Glean stands out in the mid-market segment, not just as another document search tool, but as a specialist in making institutional knowledge actually useful. Their approach to document search goes beyond simple keyword matching—it understands context and user intent, making it particularly effective for companies drowning in internal documentation.
Harvey AI has become the quiet powerhouse in legal tech, and for good reason. It's not trying to replace lawyers (a common misconception), but rather augments their capabilities in contract review, legal research, and document analysis. The platform's deep understanding of legal terminology and precedent makes it particularly valuable for firms handling complex cases.
Hebbia has taken an interesting approach by focusing exclusively on financial services. Their platform excels at parsing complex financial documents, extracting key metrics, and identifying patterns that might escape even seasoned analysts. It's particularly adept at handling the nuanced language of financial reports and regulatory filings.
Palantir AIP might be among the most intriguing ones. While others chase after commercial applications, Palantir has doubled down on defense and enterprise operations. Their platform handles sensitive data with military-grade security while maintaining the agility needed for modern enterprise operations.
The real question isn't whether these platforms work—they do—but whether your organization is ready to embrace them.
The future of knowledge workers
Let's circle back to our original question: will knowledge work be fully automated by the 2030s?
The short answer? Not quite, but that might be missing the point entirely.
What we're seeing isn't a replacement of knowledge workers, but rather a fundamental shift in how knowledge work gets done. Think of it like the introduction of calculators and computers in accounting—they didn't eliminate accountants, but they certainly changed what accountants spend their time on. Similarly, work AI is reshaping knowledge work in ways that amplify human capabilities rather than replace them.
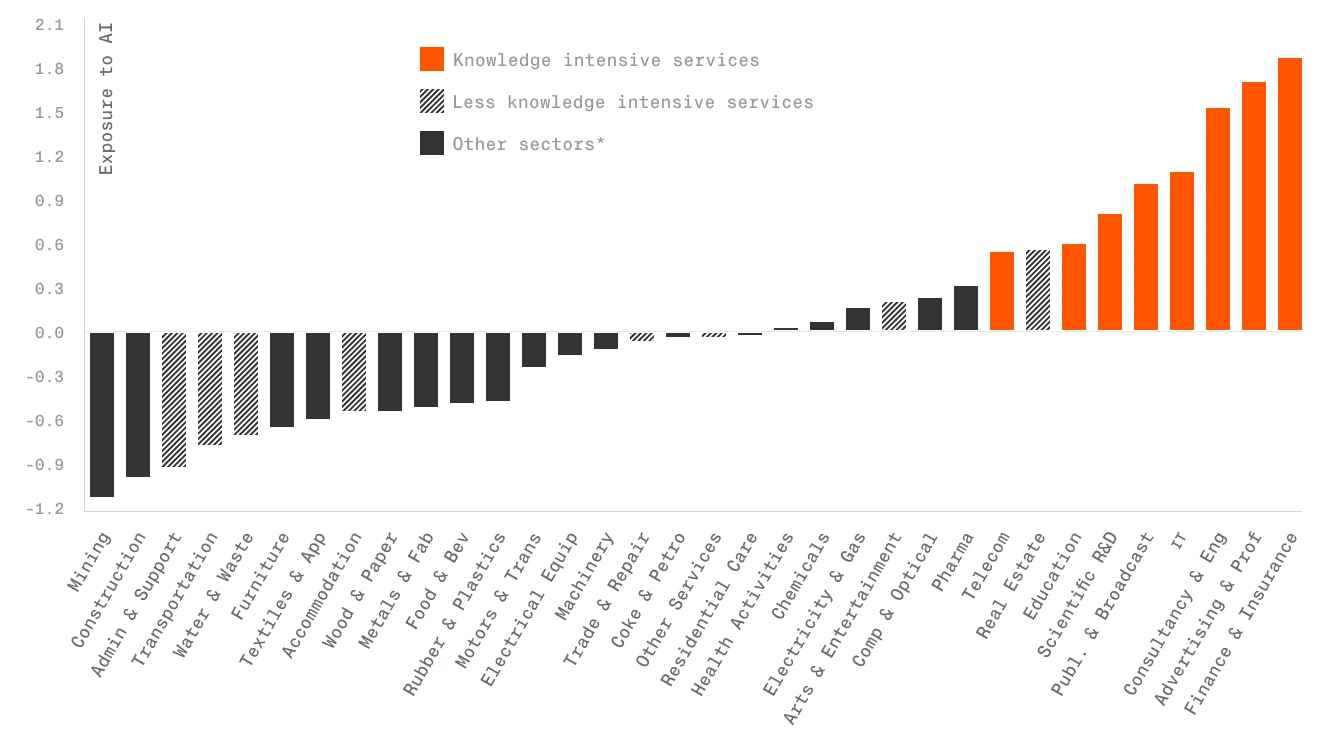
By the 2030s, we'll likely see a workplace where AI handles the heavy lifting of document processing, initial analysis, and routine decision-making. But the uniquely human elements—strategic thinking, relationship building, creative problem-solving, and ethical judgment—will become even more valuable.
Where do I start?
Rather than forcing AI into existing processes, successful organizations redesign their workflows around AI capabilities. Countless implementations fail because teams try to replicate manual processes exactly, rather than leveraging AI's unique strengths. Use AI workflow templates as a starting point to streamline adoption and avoid redundant efforts.
The key is to begin where it makes the most sense for your team. Take a look at your daily operations - what tasks make your colleagues sigh with frustration? Which processes have them muttering "there has to be a better way"? Those pain points are often your best starting places.
Ready to explore how AI could work for your specific needs? Let's have a conversation. Book a demo with our team, and we'll help you chart a path forward that makes sense for your organization.