AI implementation
Data Centric AI: The Secret of Successful AI Products
10 min read
—
Aug 31, 2023
Data centric AI is a discipline with the potential to profoundly impact the quality of AI products. We dive into the detail, and outline how you can embrace the methodology behind great AI.
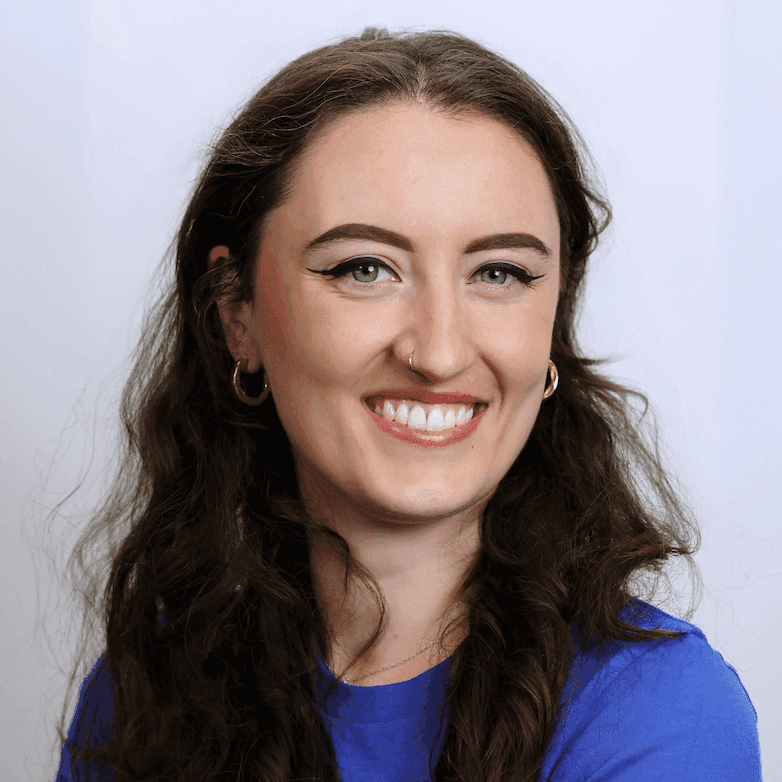
Senior Content Marketing Manager
As a wave of AI innovation takes hold, we’re witnessing a litany of new AI products, from life-saving applications in healthcare to intelligent monitoring software in retail and E-commerce. As the globe prepares to embrace AI, a distinct divide is appearing between AI solutions securing sustainable value, and those rapidly waning into obsolescence.
AI-powered products face a unique challenge, powered by models vulnerable to drift, bias, or revenue-hitting inaccuracies. Similarly, these creations are forced to combat a lightning-paced rate of change, driven by new frameworks, technologies, and competitors, continually delivering new solutions to the market.
As a result, success in this space hinges on your ability to inject new, impactful knowledge into the models that make up your product.
But how exactly can you meaningfully fuel the effectiveness of your AI?
In this article, we tackle the secret to sustainably securing long-term value from your AI products: Data centric AI (DCAI). We discuss what it is, how it works, and the importance of embracing data centric AI if you wish to create products with impact. To dive right in, click any of the below topics to get started.
What is data centric AI?
Principles of data centric AI
Challenges of data centric AI
Benefits of data centric AI
How can you use data centric AI to build better products?
What is data centric AI?
Data centric AI is a discipline first put forward by Andrew Ng, a globally respected technology entrepreneur, the former Co-Founder of Google Brain, former Chief Scientist at Baidu, and current adjunct professor at Stanford University.
Prior to data centric AI, the traditional way of approaching AI development was to focus heavily on the code that would make up a model. For the time - it was the right choice, with much work needed to elevate code to a level that would truly democratize AI use for the masses.
However, having witnessed many evolutions in AI, Ng soon saw a shift from code being the focus of development, to data. In his own words,
“In the last decade, the biggest shift in AI was embracing deep learning. In this decade, I think the biggest shift in AI might be a shift to data centric AI.”
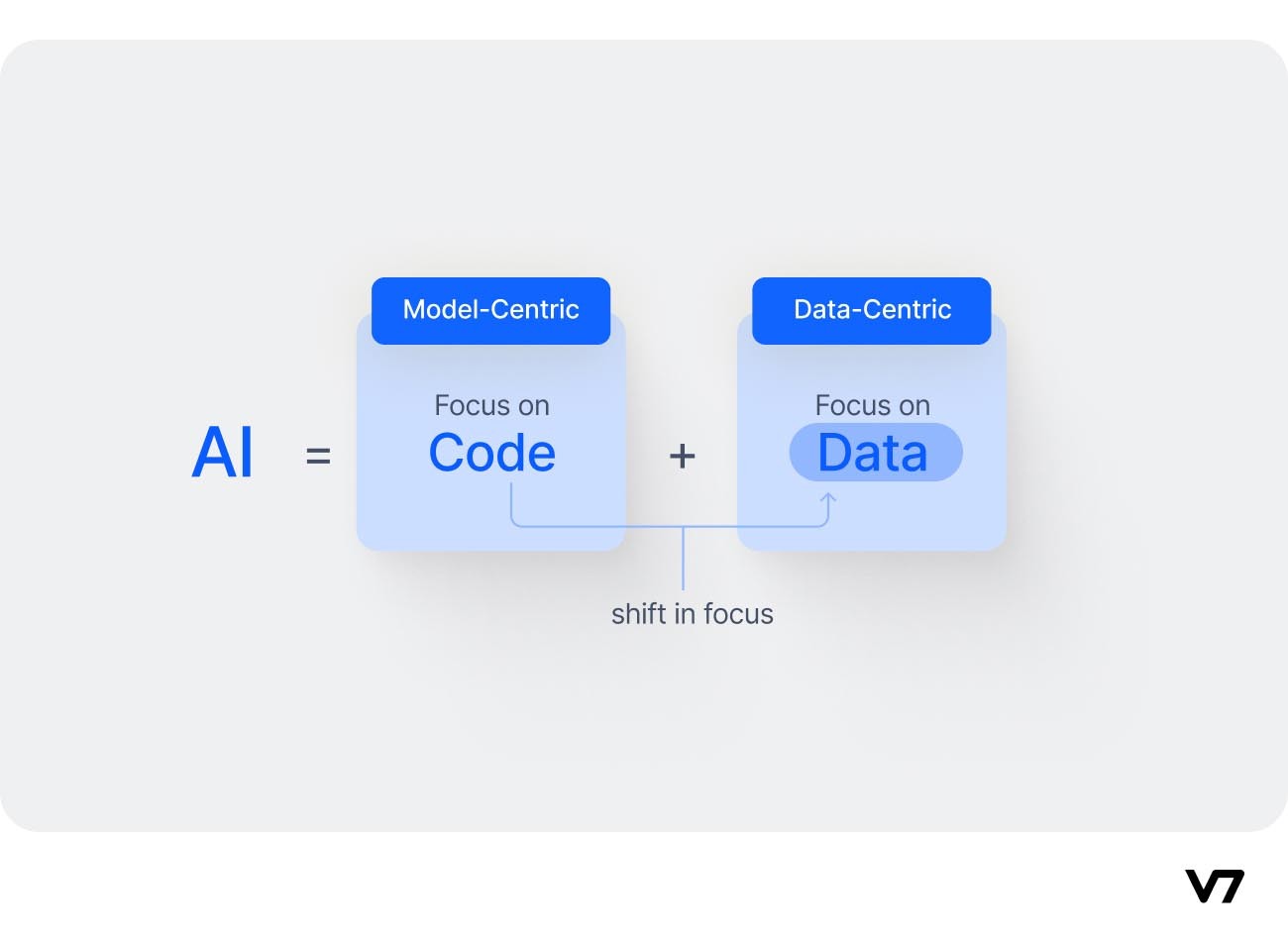
With code being, in many ways, a “solved problem”, practitioners increasingly looked to data as a means of delivering impactful changes to model development. Rather than perceiving data as something to be simply fed into the machine, it became a core means of creating better, more performant, and more reliable models.
In certain scenarios, such as AI defect detection, companies that constructed models using a data-centric approach achieved a 17% enhancement in performance compared to those using a model centric approach. Below, you can see metrics for a model-centric approach, versus a data-centric approach.
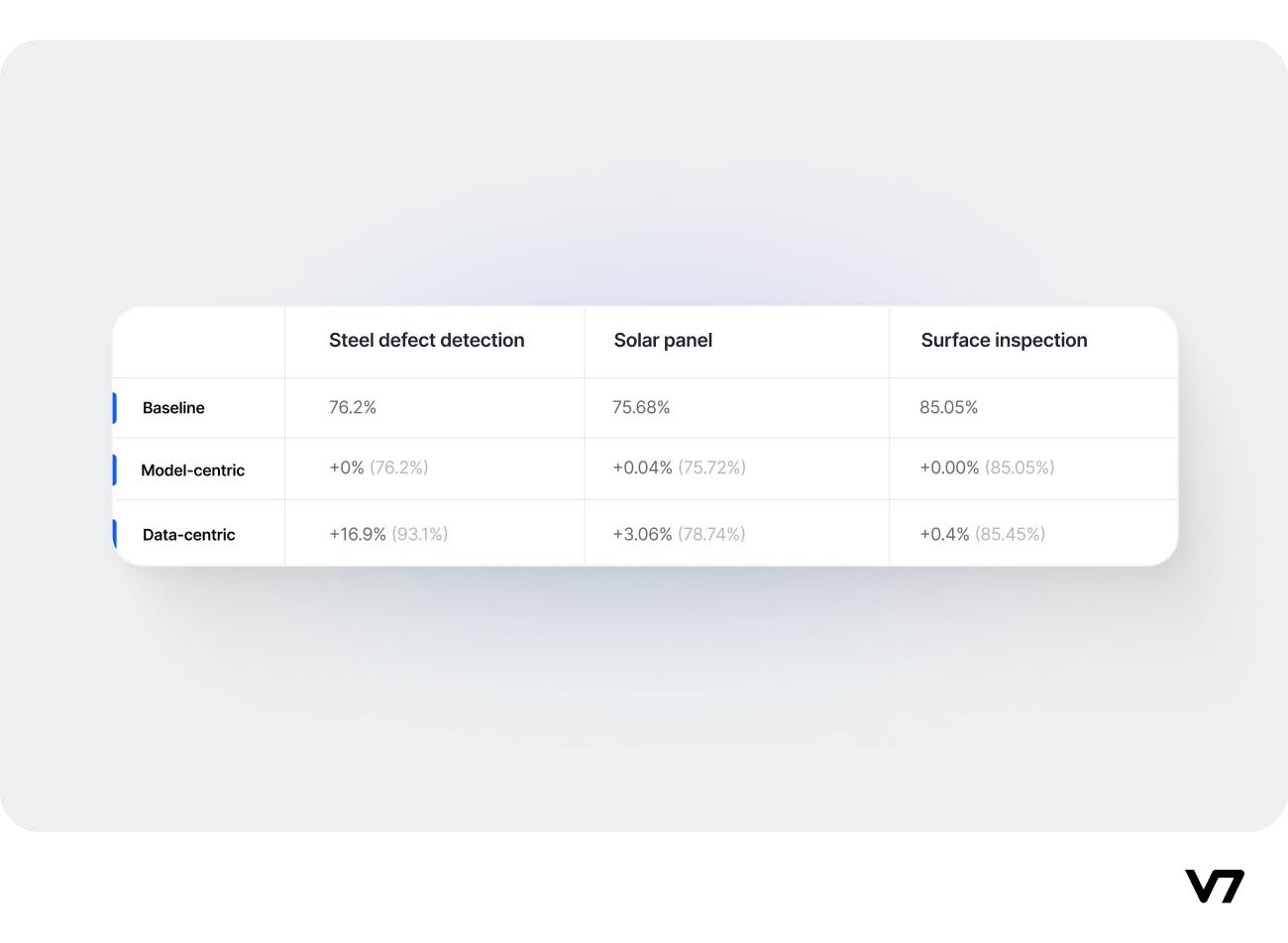
The impact of a code-centric focus versus a data centric approach
As a result - data centric AI was born, as a discipline that involves “systematically engineering the data used to build an AI system”. The rise of this discipline gave way to new tools and practices that would make the improvement of data consistent, reliable, and systematic.
Principles of data-centric AI
At a high level, data centric AI can be broken into a few principles.
The integrity of training data
As the backbone of your product, your training data will need to be accurate, representative, and sufficiently sized to reliably deliver a performant model. On the importance of data, Andrew Ng states,
“Whereas we all know data is important, we often think of data as this thing that is handed to us, rather than something that we need to monitor and engineer and fix.”
On the crucial importance of training data, V7 Founder, Alberto Rizzoli, weighs in,
“Labeling the right amount of data to contribute to AI knowledge is an exhaustive process, yet the primary correlator of model accuracy. Without the right training data, there is no engineering process that can save you".
In data centric AI, securing the “right” training data includes tackling - and if necessary - removing instances of noisy data, with DCAI prioritizing the quality of a dataset over its quantity. Below, you can see the impact removing noisy data can have on a model’s final output.
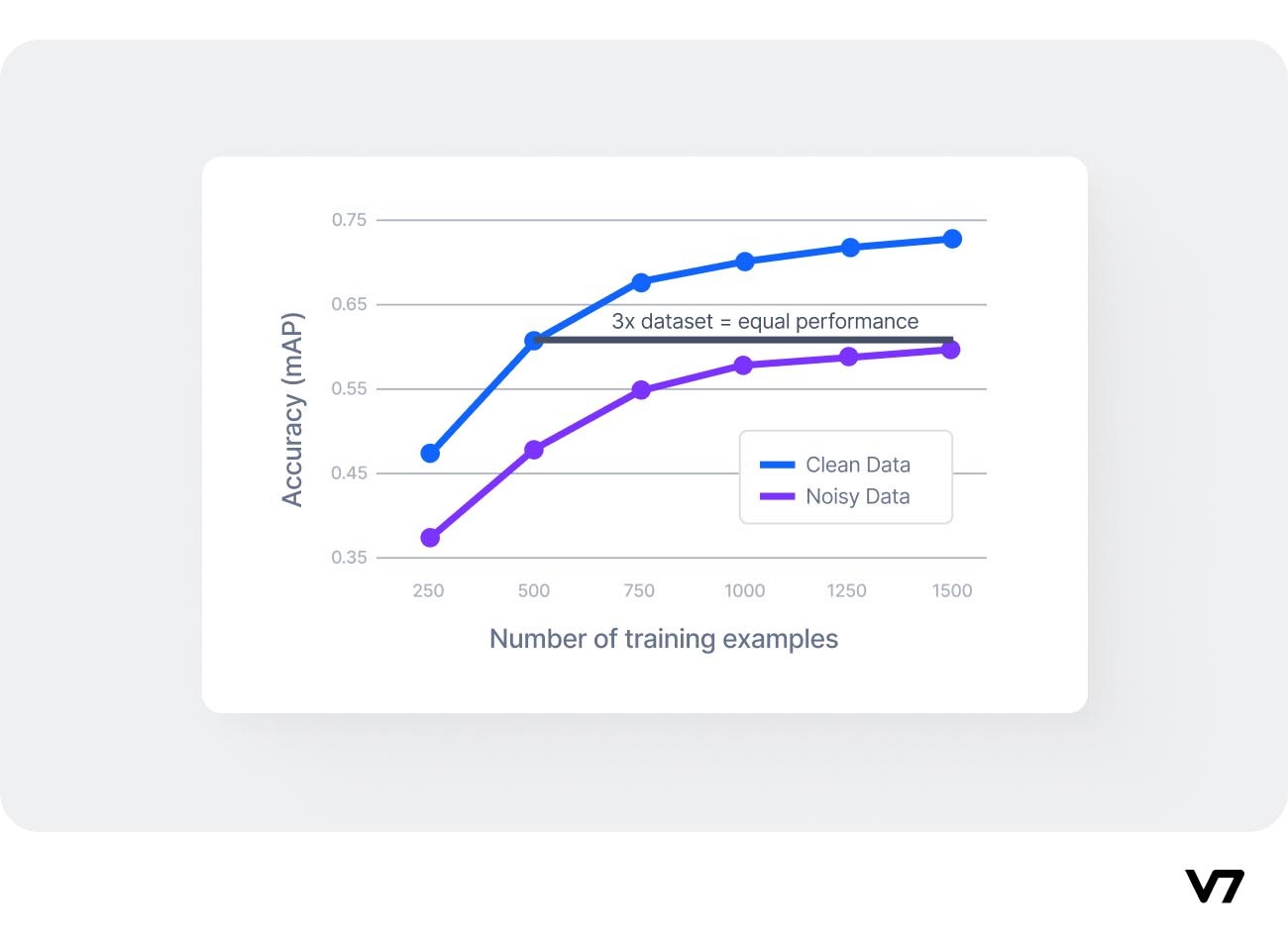
A data centric approach ensures businesses focus on the most revenue-impacting component of the AI pipeline: collecting, managing, labeling, and expanding valuable data.
Embedded domain expertise
To truly gain value within data centric AI, you’ll want to include subject-matter-experts (SMEs) throughout your development lifecycle. This includes generating datasets with the input of domain experts, for example, radiologists for healthcare use cases, and defining annotation and data management practices with the support of your SMEs.
In doing so, you elevate the quality of your datasets and embed a unified - and accurate - approach to labeling.
Pro tip: With V7 you can access over 5000+ professional annotators, from scientists to manufacturing experts. Discover our expert labeling services now.
Systemic iteration
Finally, data centric AI is all about delivering a scalable, repeatable, and effective approach to model creation. As a result, it trades the quantity of data for quality data, prioritizing processes that will create better models - and products - overall. This includes a systematic approach to continuously improving models, through error analysis, iteration, environmental conditions, and injections of new data for the AI to learn from.
As a result, you’ll need to carefully consider your training data process, from how you create a continuous learning loop, to how you build efficient training data pipelines that can be easily implemented across multiple teams. Here, intelligent tooling, workflows, and automation become invaluable.
Pro tip: Wondering whether you should buy or buy your data-centric tooling? Dive into our comprehensive Build Vs Buy guide.
Challenges of data centric AI
Despite the opportunity at hand, data centric AI can have a series of roadblocks. On this, V7 member and data centric AI enthusiast, Zach Million, weighs in,
“Since the boom of data centric AI, the importance of high-quality data is no secret to the world's best machine learning and computer vision teams.
However, the process of managing and creating this ground truth is still, even today, vastly overlooked. In fact, most machine learning teams spend around 70% of their time managing the process of ground truth creation rather than training their models.”
Due to the crucial importance of quality data in the DCAI approach, teams are likely to face three core issues:
Increased emphasis on data collection
Increased importance of annotation accuracy
Increased need for development consistency
To combat these challenges, you’ll first need to tackle how you’ll go about building a pipeline of high-quality training datasets (V7 founder Alberto Rizzoli explains exactly how in our article: “An Introductory Guide to Quality Training Data for Machine Learning”.)
Next, you’ll need to assess whether you’ll build your own training data platform, or leverage the many options on the market. A good platform will allow you to manage datasets, rapidly annotate, automate processes, conduct QA, train models, version, and benchmark models, while providing easy-to-use dashboards that make efficiency, consistency, and profitability easy to control.
Below, you can see a comparison of the best image annotation tools on the market, and how they can help you achieve data centric AI development.
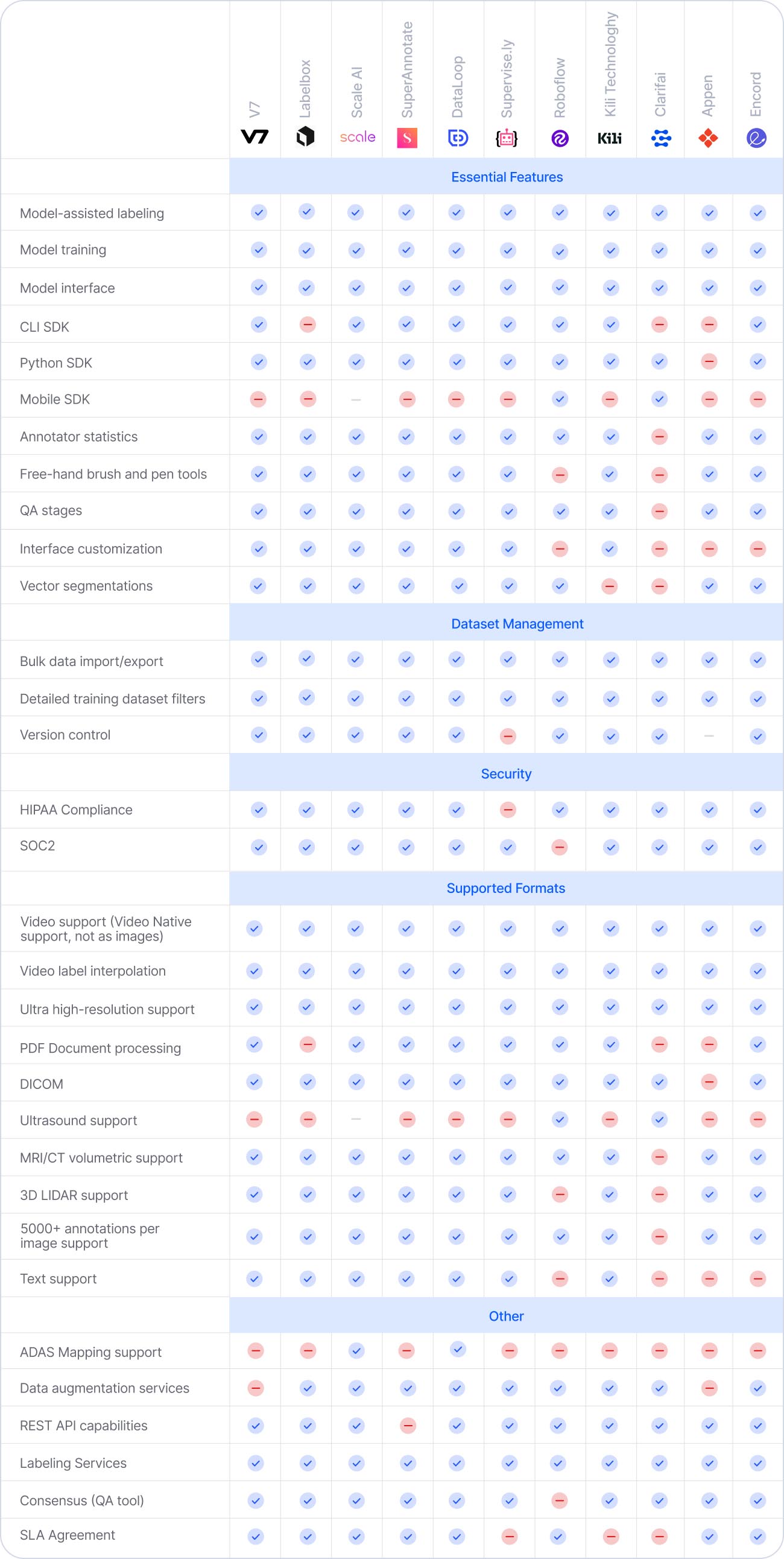
Benefits of data centric AI
In many ways, data centric AI is a future-focused approach, with emphasis on the continued performance, reliability, and sustainability of your AI models. Below, we tackle some of the core benefits of a data centric approach to AI.
Greater model performance and efficiency
As the well of knowledge from which an AI learns, your data, and your ability to continually add to it, is the most impactful thing you can focus on to create better AI products. By focusing intensely on the data that feeds a model, businesses come face to face with one of the most impactful components of their AI product’s success.
By embracing a data centric approach to AI, teams embed a systemic approach to data engineering that standardizes AI product development. This includes prioritizing easy-to-use UI, clear workflows, seamless dataset management strategies, and rapid, fit-for-purpose annotation tooling.
This strategy shift results in more performant models (bolstered by quality data that is accurate), a more rapid route to market (thanks to pipelines that deliver value faster), and an agile product development process that can quickly evolve to meet evolving demands (thanks to repeatable processes).
Reduced costs
The shift from model-centric to data-centric AI is profoundly impactful, both on the quality of your product, and the overall development costs of the project. While a data-centric approach does increase the investment required in data preparation, cleaning, and labeling - it delivers long-term benefits (such as model accuracy) that often far outweigh initial upfront costs.
Beyond the cost benefits of more accurate models, data-centric AI also has a higher propensity to deliver models that are more robust and easier to maintain. This in turn curbs maintenance costs, while making scaling a feasible reality.
Similarly, the collaborative approach of data-centric AI development (through leveraging the expertise of domain experts and engineers), can often fuel more innovative solutions within the overall project - impacting the model, and the bottom line, for the better.
Finally, data centric AI’s intuitive approach to development can also mitigate overall cost of AI production. Development time, otherwise spent wading through code, is saved only for the most impactful tasks, while model development is streamlined with human-in-the-loop inputs, and accelerated by tooling such as workflows, dataset management, task assignments, and consensus stages.
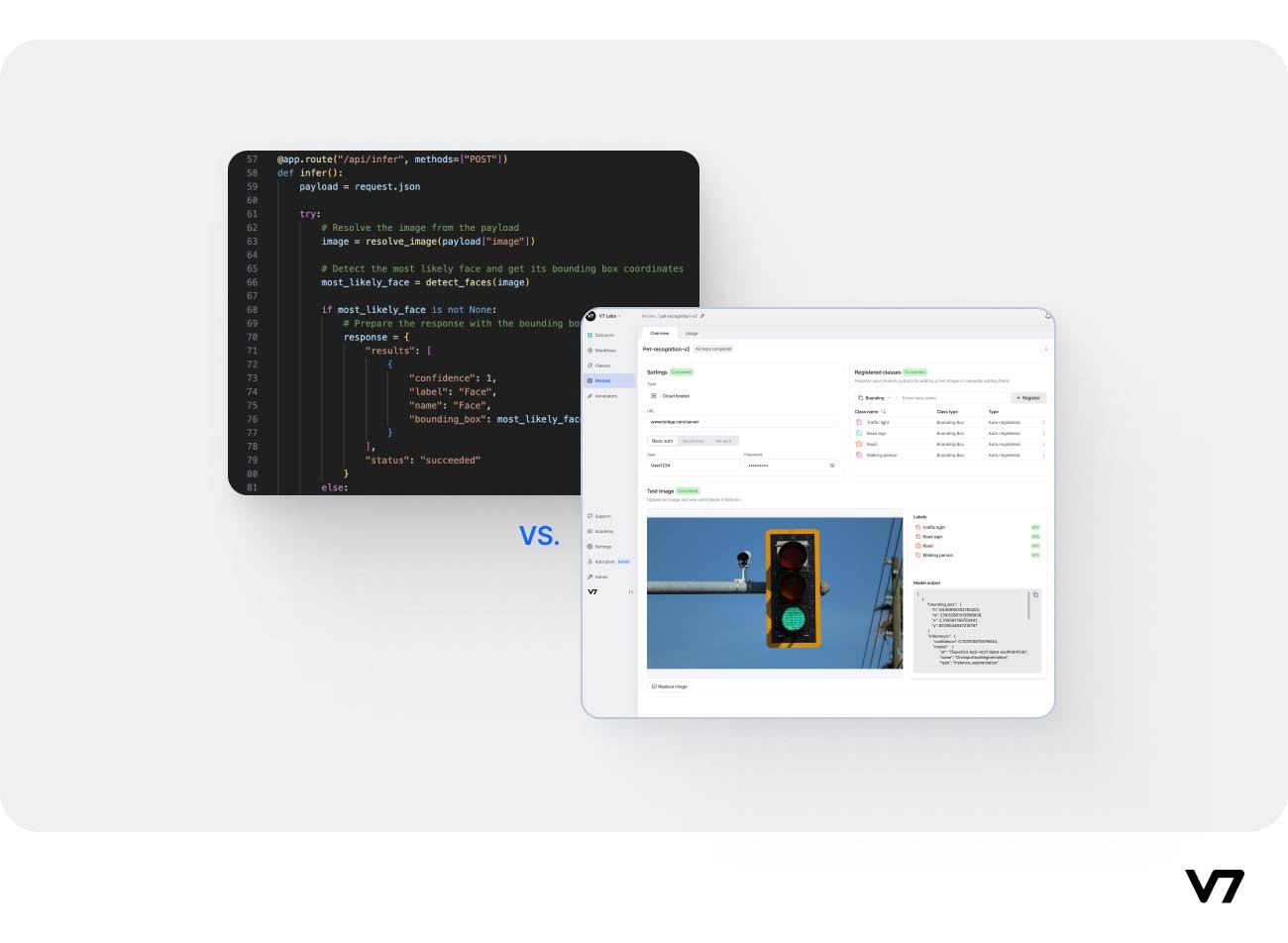
De-risks AI development
The AI that makes up your product can be vulnerable to model drift, which refers to a decline in a model’s performance as a result of environmental shifts. This is broken down into two types, concept drift, and data distribution drift. Concept drift happens when patterns or relationships surrounding the data evolve, resulting in the model needing to adapt its underlying understanding. Data drift is when the data the model is trained on, shifts and differs from the data that the model is used on.
Understandably, model drift is a challenge for a number of reasons: It can outdate your product - or make it obsolete, it can increase the risk of your model displaying unwanted behaviours, it can cause reputational damage to your brand, or it can simply degrade the performance of your model over time. Fortunately, data centric AI prioritizes data monitoring and retraining as an intuitive part of its process, with a laser focus on continually improving your AI model.
In a data centric approach to AI, you would:
Continually monitor your model output, with a commitment to iterating the model throughout its lifecycle.
Continually source new data to retrain the model.
Implement rigorous QA processes that make model drift easy to spot and fix.
Leverage feedback loops, RLHF, and user testing to maintain the performance of your model.
Implement a human-in-the-loop process to catch low-confidence results or poor user feedback.
Put simply, data centric AI not only delivers better models overall - it cements a process that continually ensures your AI product remains accurate, reliable, and valuable.
How can you use data centric AI to build better products?
As we’ve covered, data centric AI, in many ways, is considered the future of AI product development. To implement this approach, you’ll need to embrace clear-to-use, repeatable, and systematic approaches to your data engineering. Below, we’ve collated our top ten best practices for implementing a data-centric approach to model development.
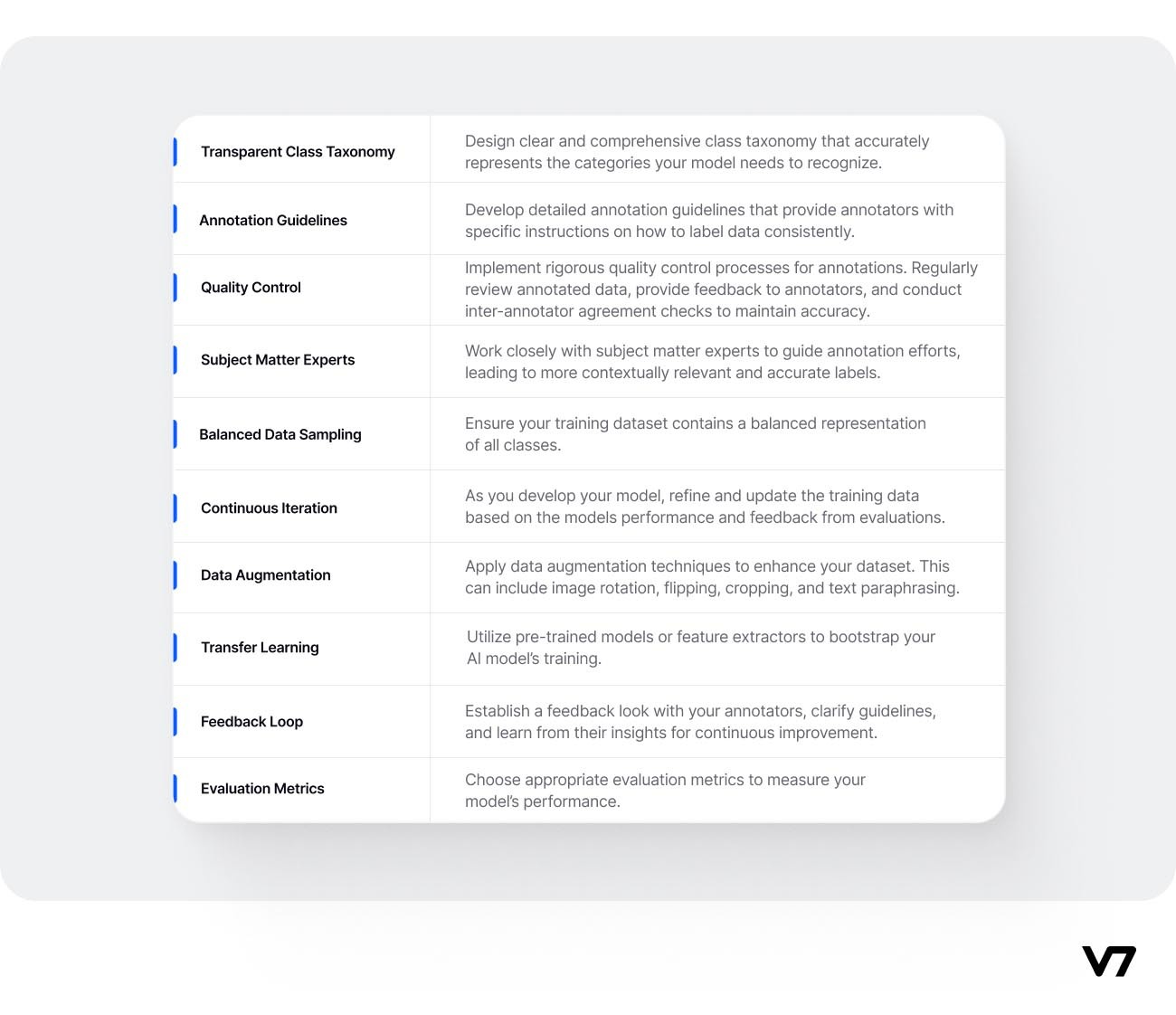
One of the most impactful ways of doing this is to leverage a powerful training data platform that is built with data centric AI in mind. A DCAI-focused platform will prioritize simple workflows, easy to navigate UI, bleeding-edge accuracy for annotation tooling, and infrastructure that fuels the interpretability, fairness, and robustness of your models. Finally, these platforms will embed a loop of development that drives the accuracy, sustainability, and profitability of your models.
Below you can see an example of how V7’s Darwin embeds a data centric loop of development that keeps enterprises like Boston Scientific, Huawei, and Wanzl on top.
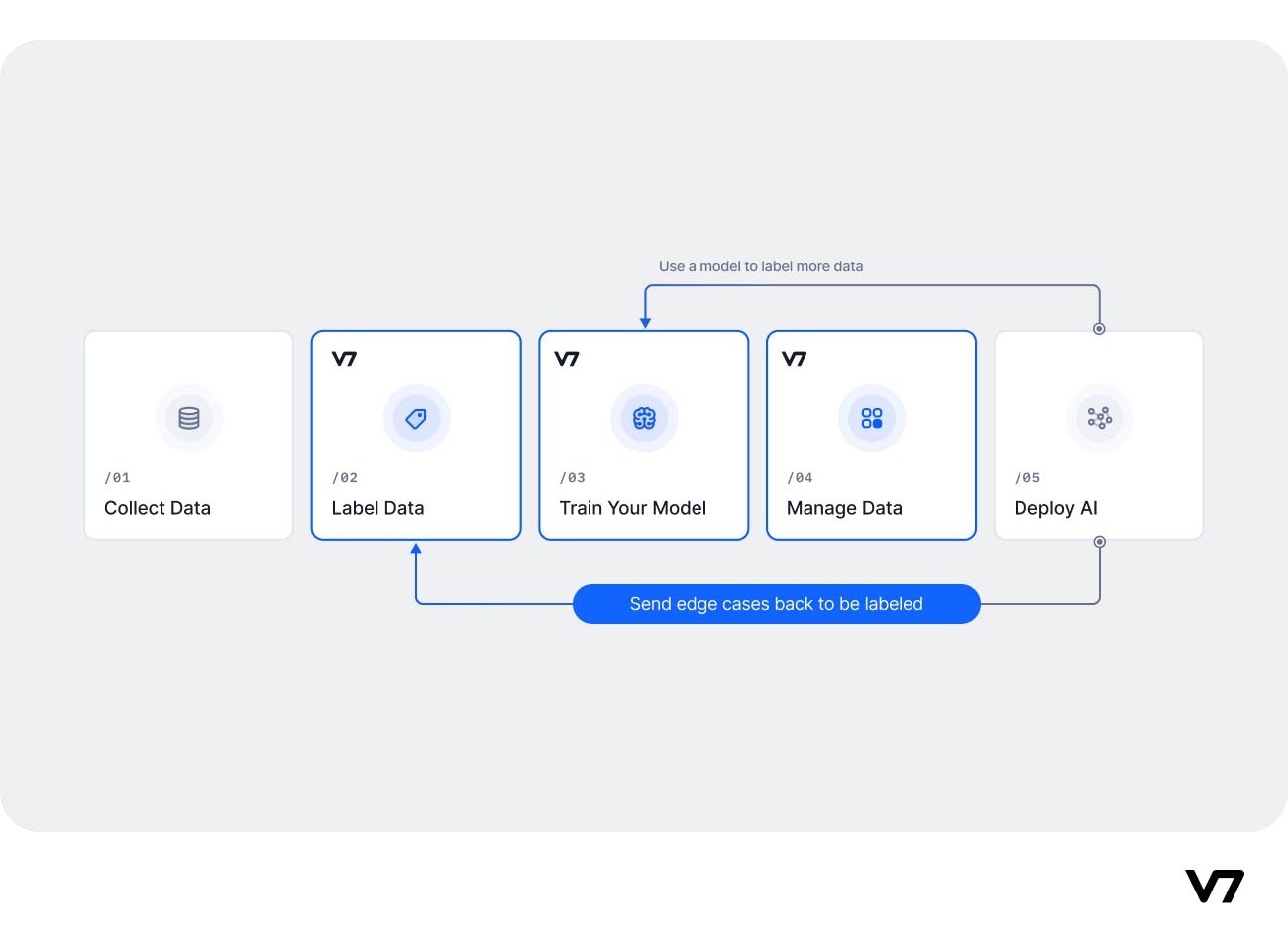
Wondering how V7 can help you make better AI products? Try Darwin now or request a demo.