AI implementation
6 AI Applications Shaping the Future of Retail
12 min read
—
Oct 18, 2021
Here's how Machine Learning and Computer Vision can help AI-first retailers improve customer experience, increase profitability, and stay ahead of the competition.
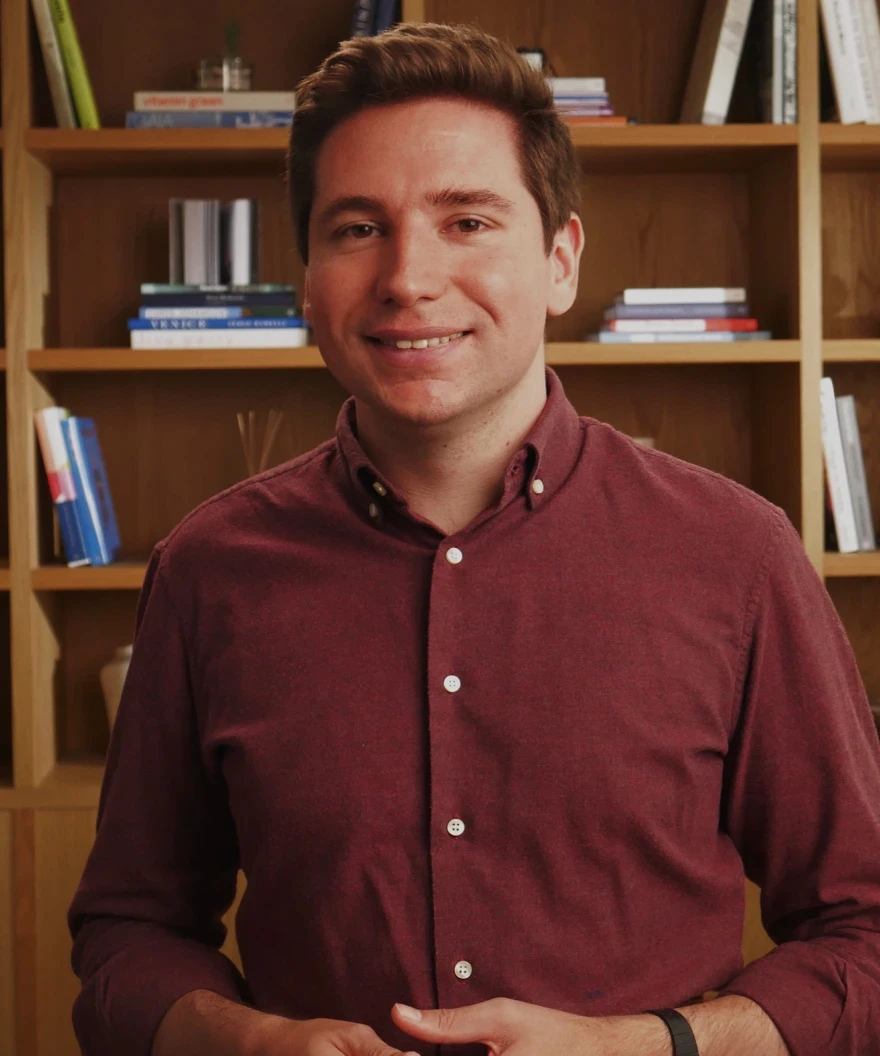
Co-founder & CEO
Retailers who want to stay on top of their game are increasingly turning to Artificial Intelligence.
AI gives them access to streams of data that improves speed, efficiency and—ultimately—their business decisions.
Indeed, more and more retailers are throwing money at AI, with global annual spending expected to reach 7.3 billion by 2022.
In 2023, meanwhile, 325,000 retailers will be using machine learning in some form.
Moreover, retailers are already well-prepared to leverage AI, with their CCTV cameras able to collect relevant visual data.
This then helps the store implement computer vision.
Techniques such as image classification, object detection, optical character recognition, human pose estimation and activity recognition are already playing a significant role in helping retailers improve both the customer and the employee experience.
In this article, we’ll look at some of the most exciting applications of AI in retail, from computer vision to document automation and describe the technology behind them.
Here’s what we’ll cover:
Cashierless checkouts
Inventory management
Customer behavior analysis
Auditing product placement and planograms
Crowd analysis
Optical Character Recognition for retail
Ready to streamline AI product deployment right away? Check out:
Now, let’s dive in!
Cashierless checkouts
A cashierless checkout is one that doesn’t require a cashier. Instead, a customer can enter the store, add groceries to their cart, pay for the groceries and exit the store without having to deal with a human assistant at any point. Awesome.
Via computer vision and big data analytics, retailers can turn traditional shopping into smart shopping very soon.
A cashierless retail store combines the use of thousands of cameras, loT sensors and computer vision-based systems to:
Detect and understand customers’ interactions
Monitor the movement of products (thanks to object detection algorithms)
Automatically detect prices
OCR software is also great at reading prices and product namesI
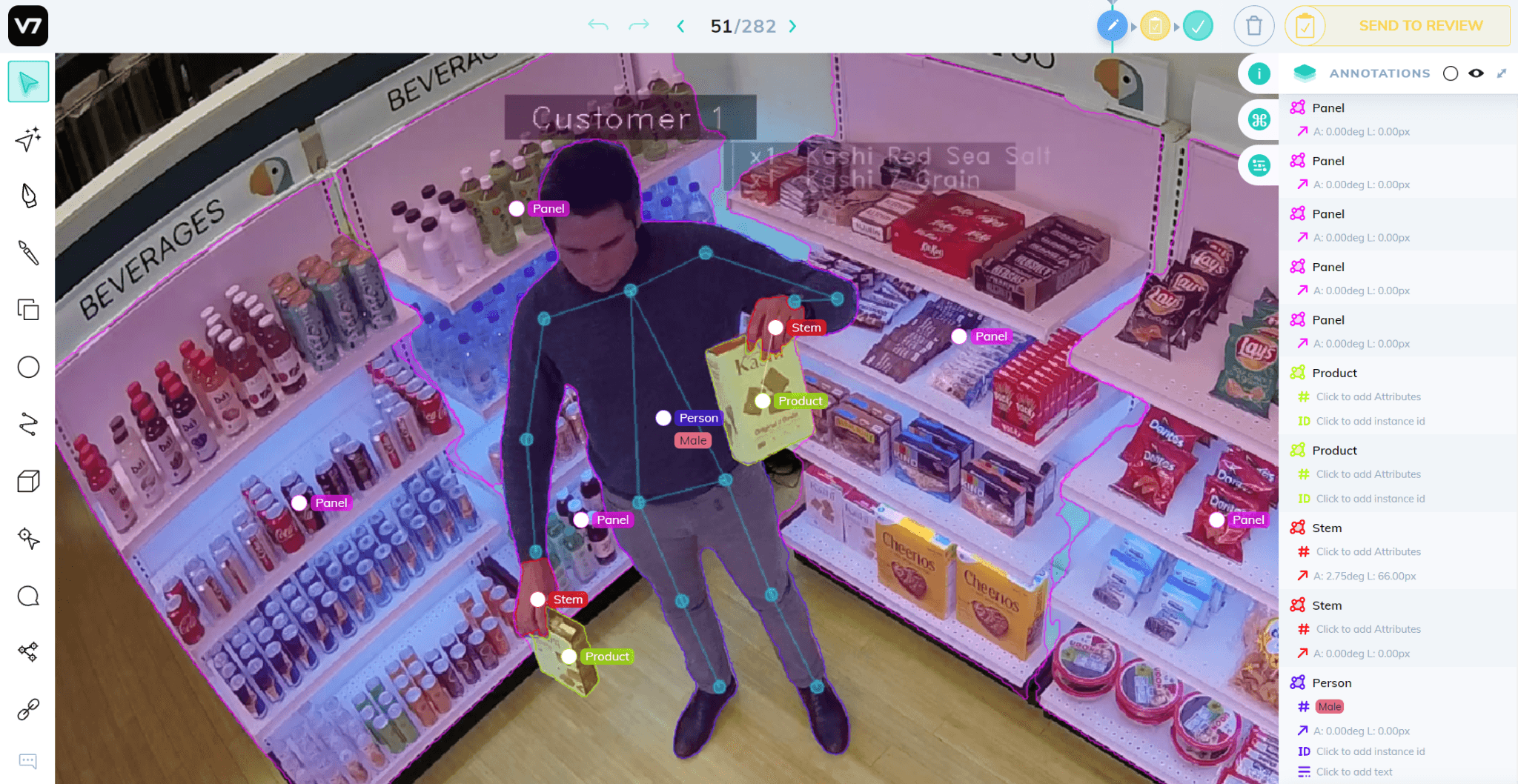
A cashierless checkout may work like this:
Before a customer enters a store, they need to download a smartphone app. Once inside the store, the app is authenticated by a QR code. If the retail store has introduced a smart shopping cart—such as the AI-powered, weight sensitive cart produced by Caper, which identifies exactly what a shopper has placed in their cart—the customer can then log into the cart, before said cart automatically scans each item with a barcode.
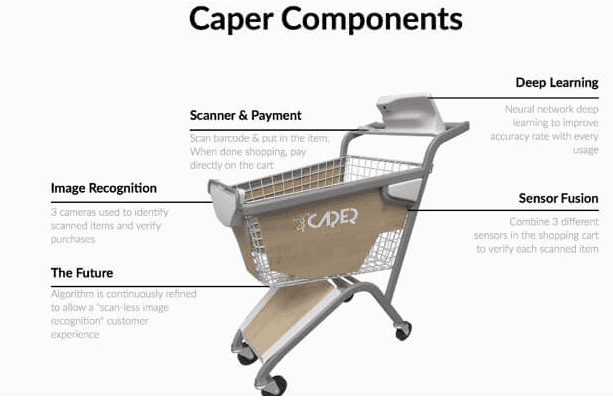
When the customer has finished shopping, they then need to enter a sensor-enabled lane which automatically charges their card.
The process doesn’t end there, however.
Using big data analytics, the smartphone app is able to record, store and use data about each individual shopper so that it can a) identify customer behaviour and b) personalize future recommendations.
Amazon has already instigated the cashierless checkout revolution with its Amazon Go cashierless stores. Other chains, including Walmart, Kroger and Sam’s Club had announced that they will soon follow suit.
There are two major benefits to cashierless checkouts:
Reduced waiting time for the customers
Less money spent on hiring shopping assistants for the retailer
The technology behind the cashierless store, meanwhile, looks like this:
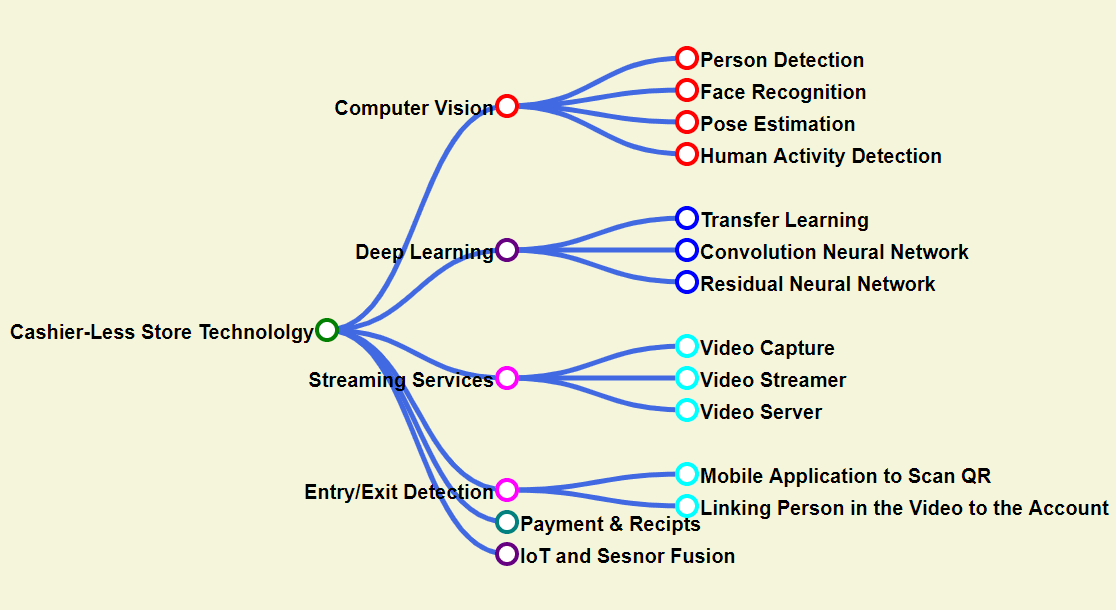
How quickly can a cashierless checkout expand?
At the moment, the development of the technology is limited by the fact that so much labeled data is needed for most computer vision problems (post estimation and activity detection especially).
There are also concerns in regard to the ethics of using facial recognition (data security).
Inventory management
Inventory management is hugely important because it helps you understand what you need, at what level, at what time—and at what price.
Inventory management in retail has been undergoing a digital revolution for the last few years, and now that retailers are starting to use AI, they can better optimize their:
Supply chain
Pricing
Promotional planning
Computer vision systems are used to capture images, before employing image segmentation and object detection algorithms to track items on shelves and carry out an entire inventory scan.
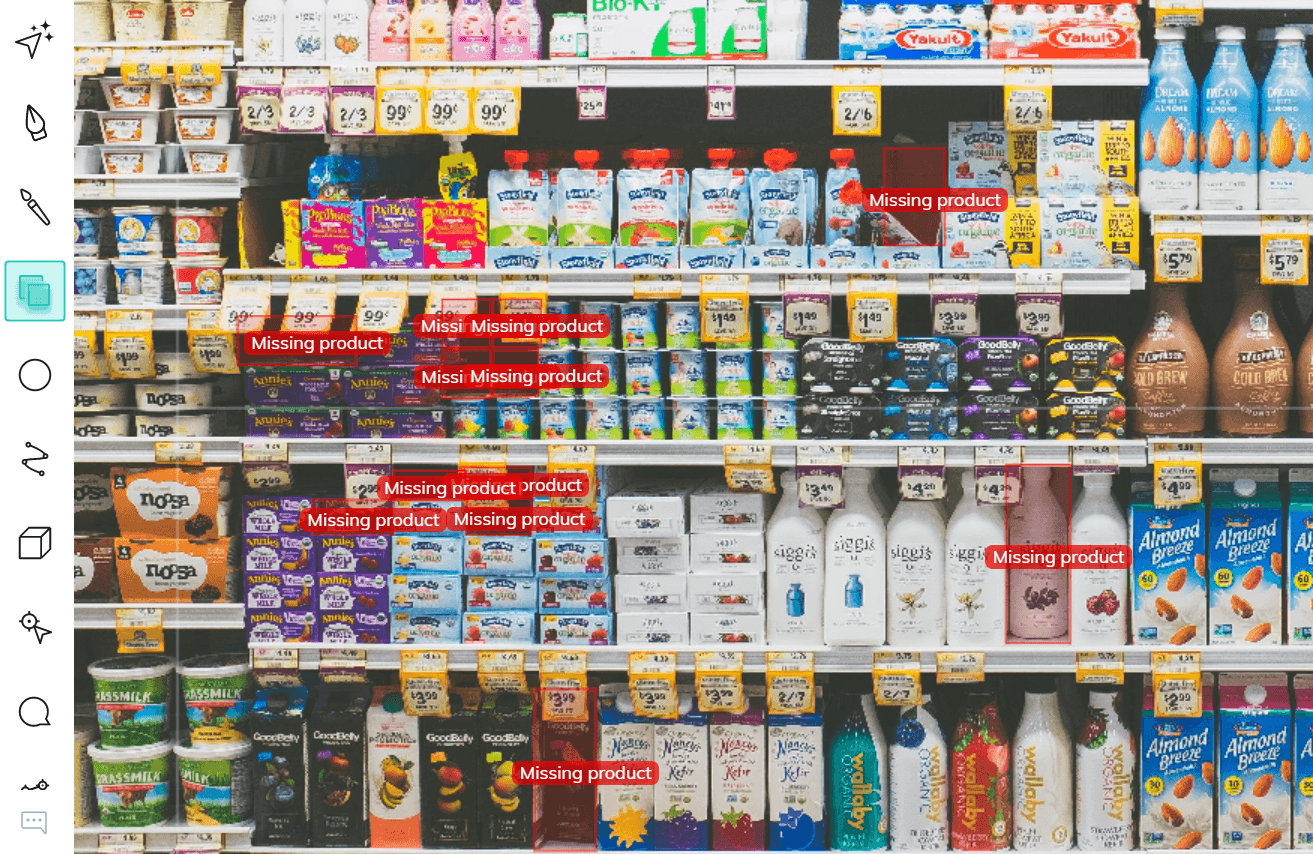
Computer vision systems also:
Provide instant notifications whenever there’s a sale or a stock-out
Boost the layout of a store
Speed-up A/B testing
Produce heat maps
Forecast how much time a product will remain on the shelf
The upshot is that AI is able to assist human workers and help to get products onto the shelves as soon as possible.
Retailers can use computer vision to create autonomous robots to help with this, too. These robots go as far as automating the stock replenishment process.
However, there are still limitations and success isn’t a guarantee just yet. If a robot is to accurately and completely cover the entire store, they need access to huge swathes of accurate visual data.
In fact, Walmart entered into a contract with Bossa Nova Robotics back in 2017, with the idea being that robots would use machine learning to identify which products were on the verge of a stock-out. And while Bossa Nova had big plans to cover 1,000+ stores, Walmart—for reasons undisclosed—pulled out of the contract.
Check out Quality Best Practices for Annotating With Bounding Boxes to learn more.
Customer behavior analysis
When it comes to customer behaviour, a few things matter to retailers:
Suspicious activity
Dwell time (time spent in front of a shelf)
Gaze time (time spent looking at a product)
Suspicious Activity
AI-powered systems are able to analyse CCTV camera footage that allows them to a) identify potentially suspicious activity and b) signal to the staff. The staff can then review the footage instantly and take the next step.
Computer vision-based systems (which use techniques such as activity recognition, object tracking, and pose estimation) have been developed also for theft detection. Because they let staff analyse movements and behaviours in real time, they are an accurate method for catching thieves.
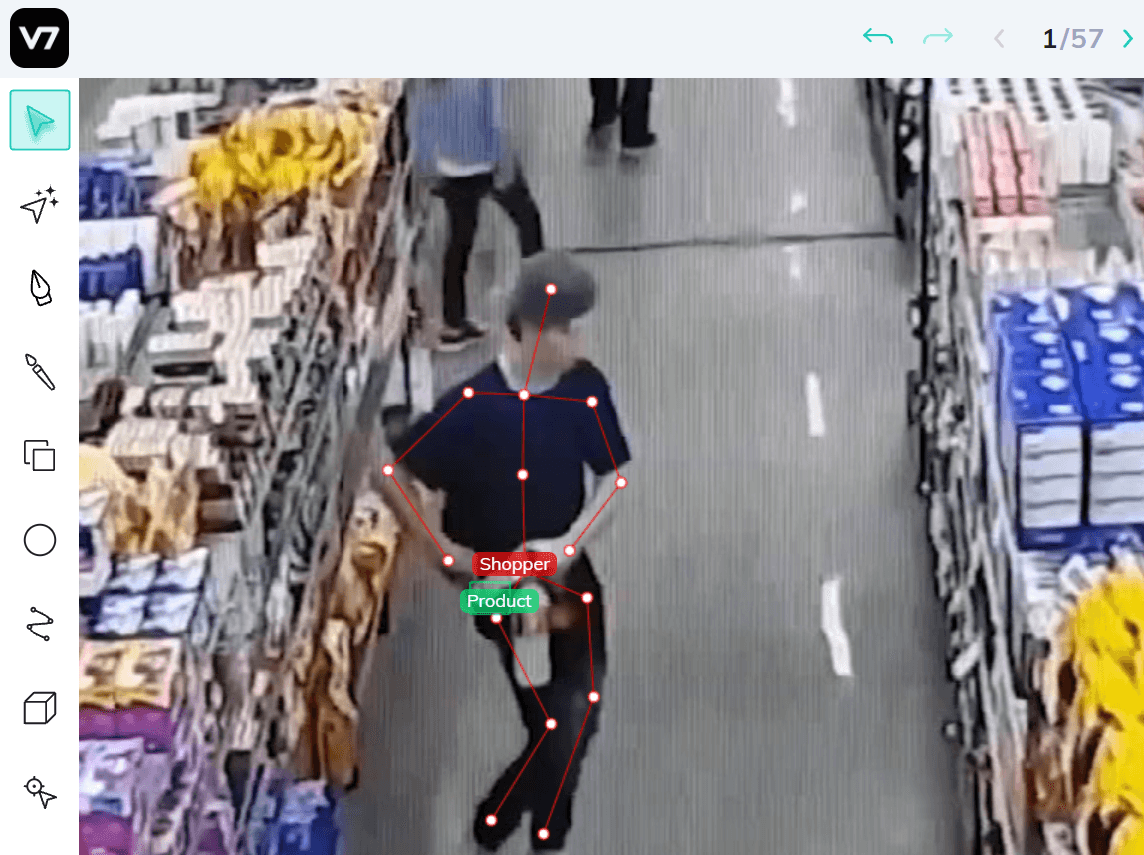
What’s more, an AI-powered camera can be used to identify other types of wayward in-store behaviour, including a physical assault. This helps to protect the reputation of the retailer, as well as provide more customer safety.
Dwell time and gaze time
O2 is one high-street store that has started to use AI technology and video analytics to measure dwell times. The overall aim is to understand more about the in-store customer experience so that they can boost conversion rates on specific products.
Improving both dwell time and gaze time allows retailers to understand how shoppers interact with different items. Using data insights, the retailers can then make more informed decisions that ensure customers add more items to their carts, thus benefiting the retailer by increased profitability.
By leveraging AI technology, a retailer can further improve the in-store experience by delivering personalization which includes—among other things—cross-selling and upselling.
By analysing data a retailer can determine the marketing value of a retail shelf versus another. In understanding this, they can better decide which products to place where, at what eye level and so on.
They can also A/B test packaging design and therefore serve the customer in better ways.
Auditing product placement and planograms
For years, performing an audit of the retail shelf has been a time-consuming process, simply because it was always done manually. Moreover, a manual audit is never fully accurate, and error rates are high.
Indeed, a planogram—which defines where products should be located and displayed in-store, as well as in what quantities—has for a long time been an imperfect process.
This is where image recognition and object detection techniques come in.
Together they are able to automate the process—check prices, the location of products and so on—with the upshot being that retailers get to understand much more about their shelf conditions and make the necessary amendments in terms of store layout improvements.
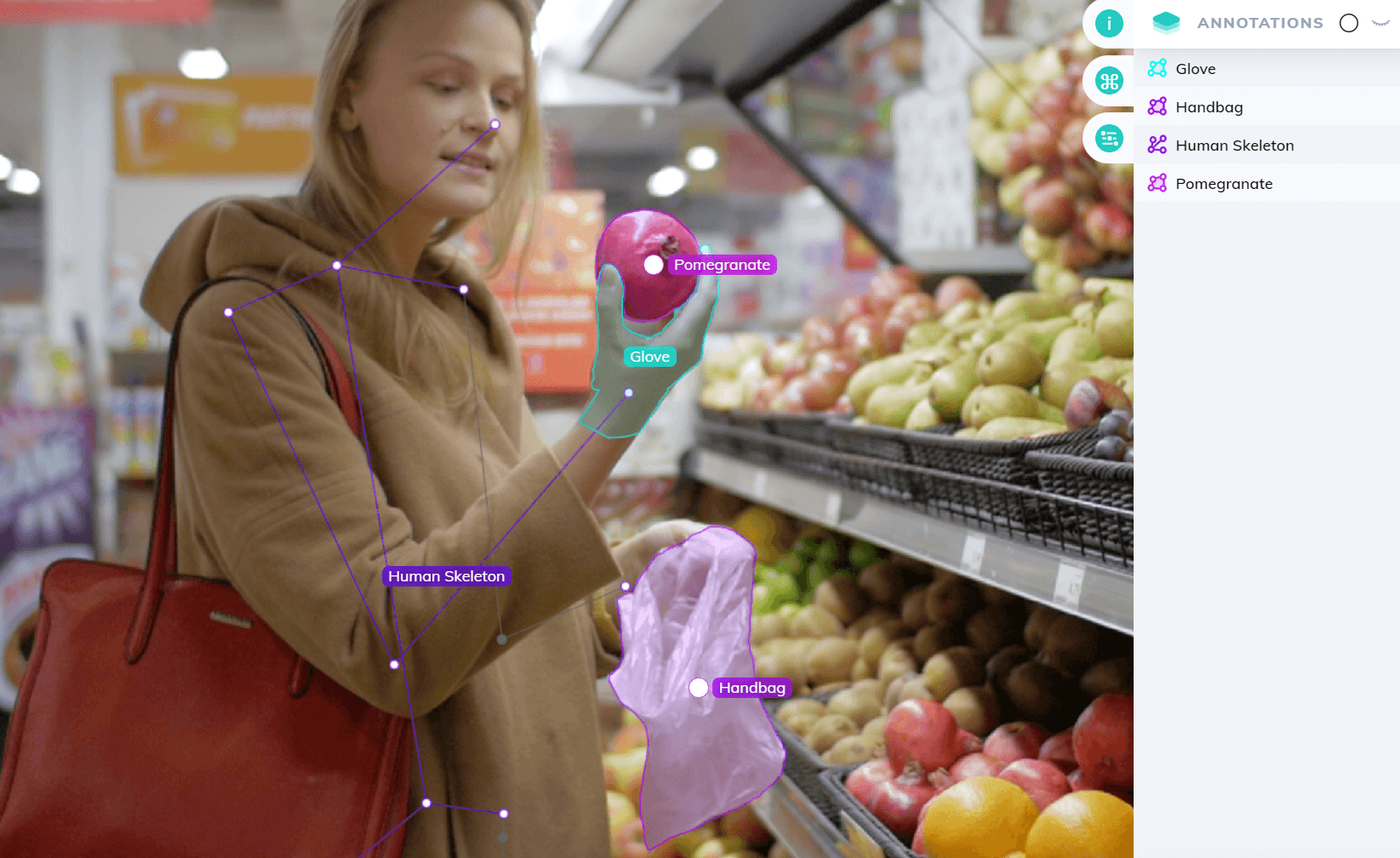
Computer vision is also being used to analyse which goods are being well-consumed and moving fast, as well as those that aren’t. This helps the store management to make a better decision in regards when to refill their stores and which items to focus on—and which ones to ignore.
Essentially, AI software will be able to offer two key KPI’s to retailers:
Planogram compliance (how well individual size distribution/quantity of products is implemented in-store)
On-floor availability (how many available products are currently on the shop floor, and not in the backroom)
When either of the two KPI’s falls below a defined threshold value, the staff are informed and can then take action.
The technology can quickly identify empty shelves, too, which for years have been the bane of both retailers and consumers. Using fixed cameras along with object detection and image recognition algorithms, retailers can instantly alert staff each time an empty shelf is found. The technology is able to identify both the shelf’s location, and the exact description of the SKU.
Startups like Fellow AI, BossaNova and Trax are already using robots and cameras to audit planograms, allowing retailers to get more accurate with specific size distribution and quantities for their in-store products. This will save time, while the technology will adapt to in-store dynamics so that, when changes to the original plan need to made, they can be made almost instantly.
Not just that, but it’s worth remembering that consumer goods companies pay good money for premium shelf space—and bots can ensure they’re fairly rewarded.
Crowd analysis
Object detection is nowadays used to detect customers entering and exiting the store. Retailers can then use this footfall data—counting people—to analyse metrics that allow them to understand why people purchase their products, their overall in-store behaviour and reactions to products (sentiment analysis), well as customer preferences.
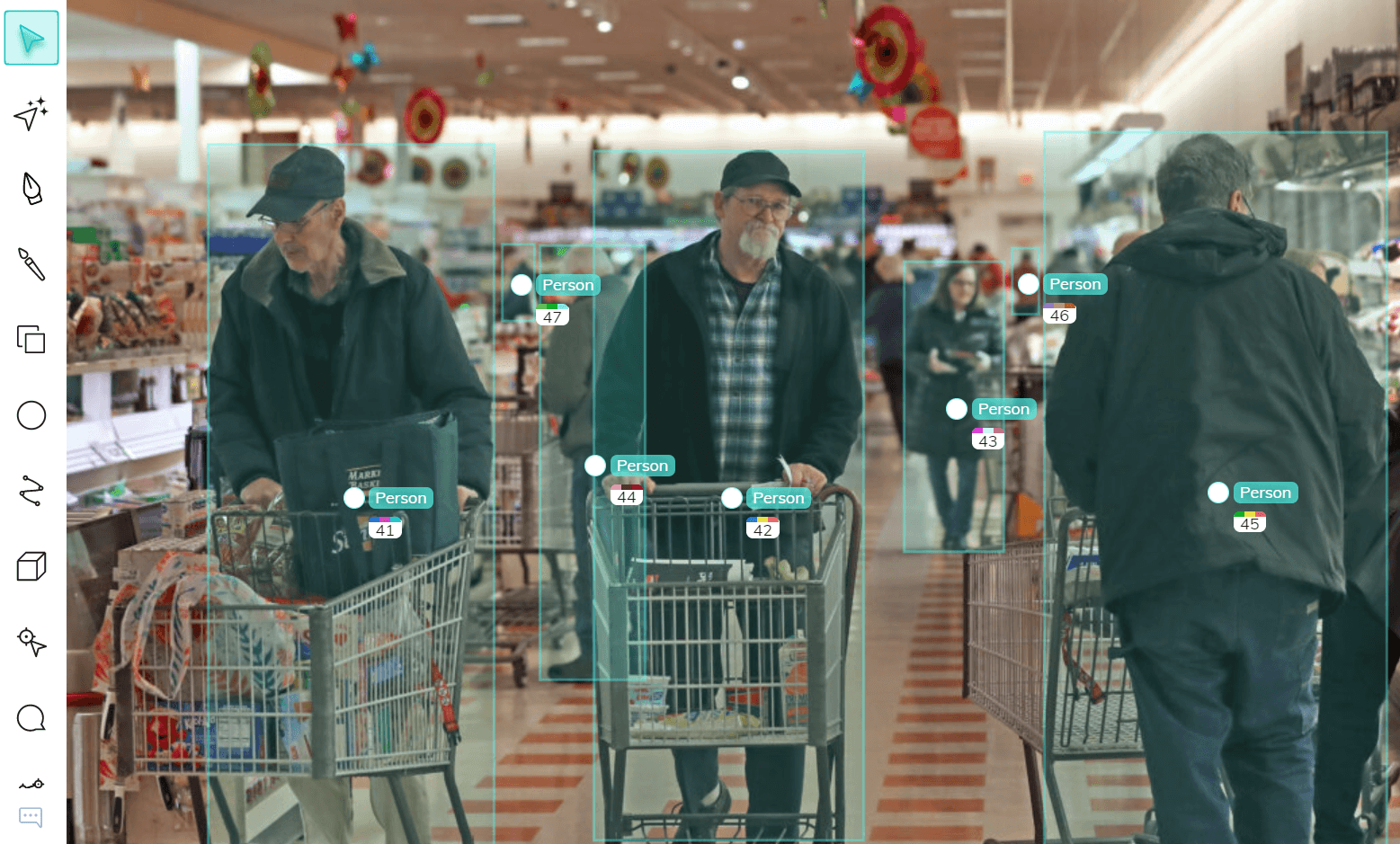
Moreover, crowd counting has other uses. It can be used to better understand and manage queues, staff changes, and it overall provides analytical data that helps the retailer improve their store management.
For example, it provides demographic analysis that shows the store manager which products are popular with which demographics. Decisions can then be made in regards product listings that help to boost conversion rates.
Retailers can also use computer vision to ensure that health and safety protocols—such as maximum occupancy—are being followed. And if a store is enforcing COVID-19 protocols, the technology can detect face masks and ensure social distancing rules are being followed.
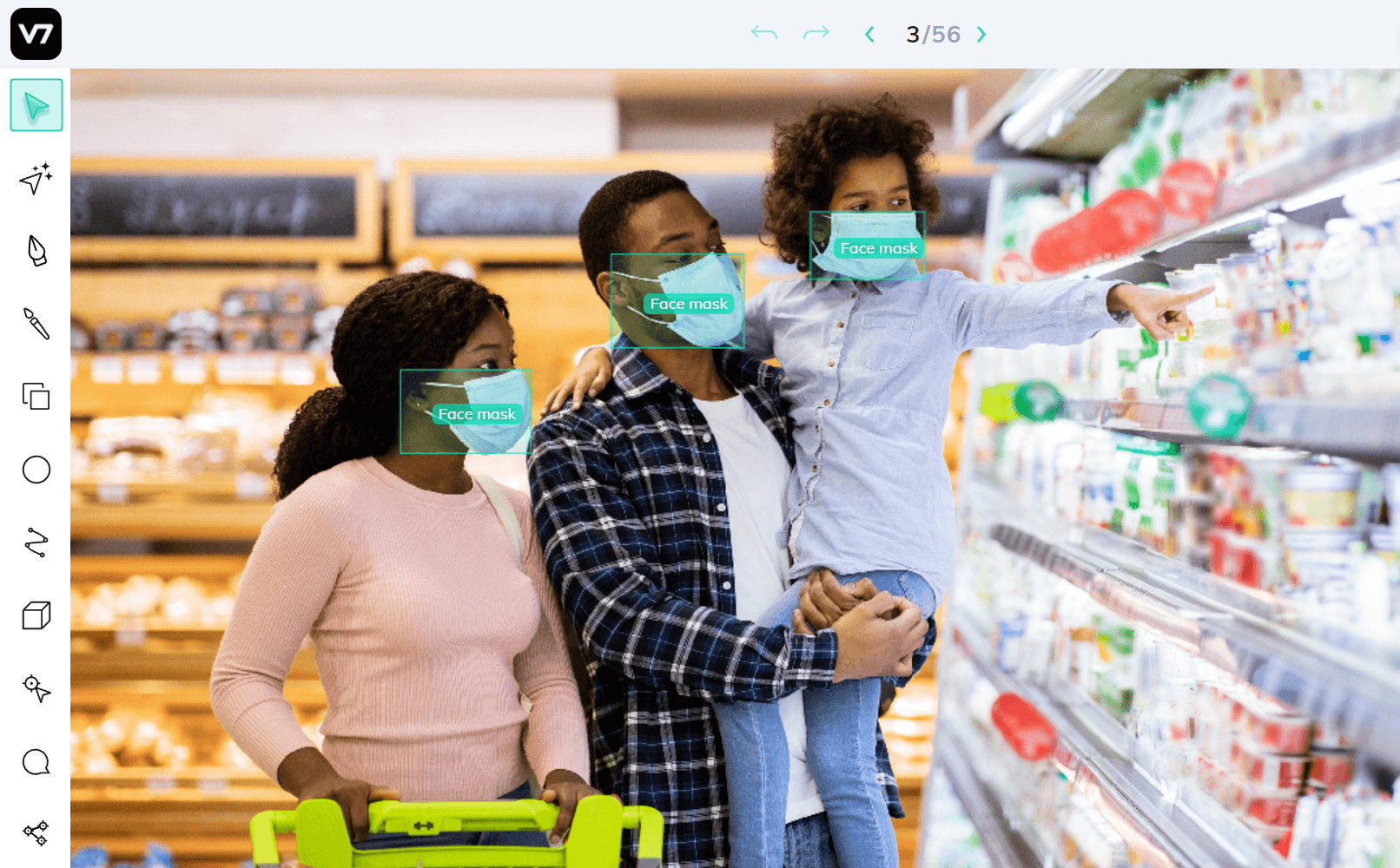
Speaking of COVID-19: The demand for assisted shopping rose greatly during the pandemic. And thanks to the object detection capabilities of CCTV cameras, gig economy workers can now enter a store and know exactly where to head straight away for any sort of product. This saves time and restores consumer trust and confidence.
The technology typically includes manual tracking, pressure mats, thermal counters and video counters. Object detection is a fundamental component in video counters, as it detects objects in each frame, while object tracking assigns unique IDs to each individual shopper detected, before tracking their in-store movement.
Of course, there are still problems that the retailer will need to overcome in order for computer vision to successfully track footfall data. The angle of the CCTV cameras needs to be just right, while good lighting is essential for accurate object detection.
Where the technology itself is concerned, object detection needs to be rapid if it’s to capture accurate traffic data, especially in super busy stores.
Optical Character Recognition for retail
Gathering together huge swathes of pricing and product data has been a major challenge for retailers. They need the data to be accurate, but for years they’ve had neither the processes nor the time.
Optical Character Recognition (OCR) uses computer vision to let retailers turn written text, receipts and other documents into a digitalised form so that machines can then a) scan it, b) read it and c) use Natural Language Processing algorithms to data process it and turn it into something meaningful super fast.
This allows for easier editing and searching, and it also smooths over—among other processes—the invoice and returns processes.
Pro Tip: Compare the best OCR software here!
For example, retailers can use OCR to search from huge repositories to quickly find the right document, as well as enter said document and search within it for the right information. It can be used to scan and extract data from a Bill of Lading, purchase orders, packing lists and more. Moreover, by leveraging the OCR invoice system, retailers can create templates for thousands of invoices without the need for user interaction.
Ultimately, this all saves time, boosts accuracy and improves customer satisfaction.
Meanwhile, replacing barcodes with OCR saves even more time, and allows the retailer to scan products and inventory (such as boxes) to accelerate the process, automate the process, and centralise it.
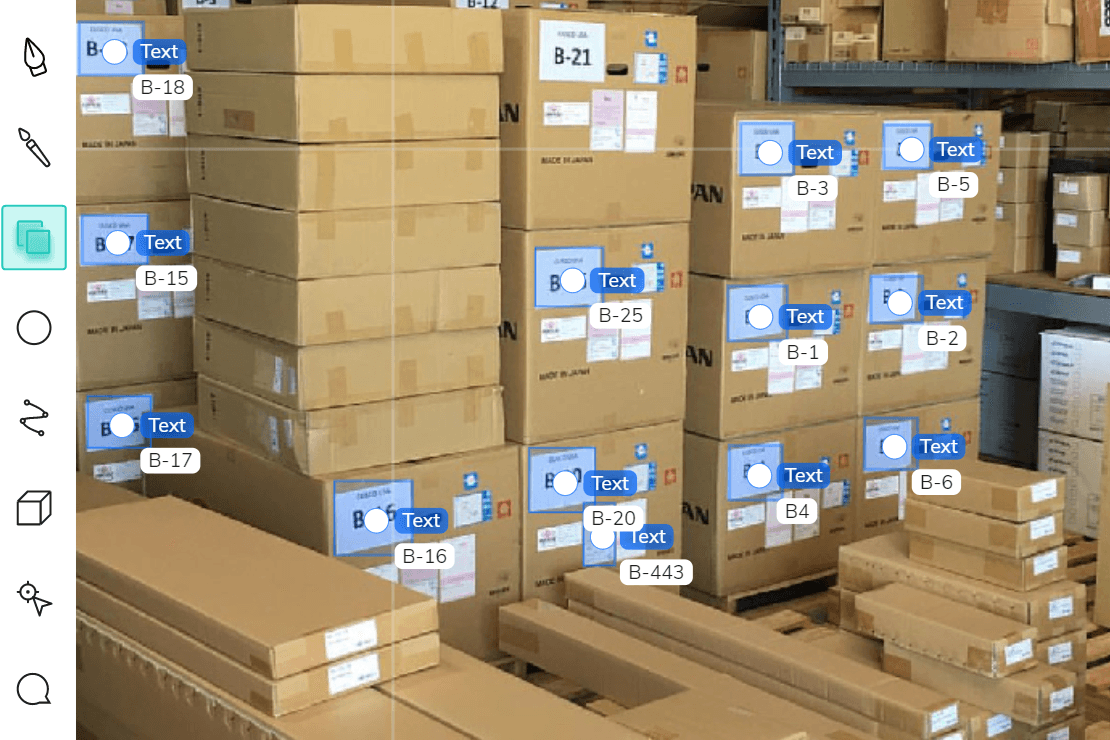
OCR is used in multiple industries, but its supermarket receipt scanning is especially useful in retail as it provides automated cash-back processing, as well as an automated loyalty points system.
What is supermarket receipt scanning exactly? It’s the process of using OCR to read receipts and identify the essential data fields, before transforming any text into a data format that’s usable (and meaningful).
Because the process is 100% automated, the retailer saves huge amounts of time while gaining access to an unprecedented amount of data to help them with better product research. They can also understand buyer behaviour better, make tweaks to their inventory and even mix up their pricing strategies.
OCR can also be used to improve services such as “click & collect” by speeding up collections so that the customer doesn’t have to wait for the necessary paperwork to be sorted.
The Future of AI in Retail
The future of retail lies with AI. The way retailers research their products, price their products and manage their inventory will depend more and more on AI, as will the way the consumer shops.
Retailers have already started to adopt AI to provide a better customer experience. Stores are using cameras to learn more about dwell and gaze time and spot thieves, and the likes of Amazon have already adopted a no checkout system.
Naturally, things might not always work out straight away, as Walmart’s partnership with Bossa Nova showed us.
But the fact that AI is efficient, accurate and predictive means that it’s only a matter of time before the likes of smart shelf sensor systems, cashierless checkouts and better planograms are adopted on a wide scale to improve the retail experience for the customer—and the retailer themselves.
If you are interested to learn more about AI applications across other industries, check out: