Knowledge work automation
14 min read
—
Jan 9, 2025
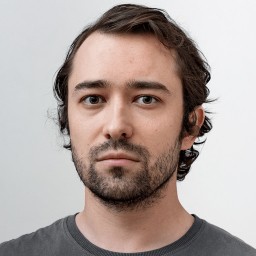
Casimir Rajnerowicz
Product Content Writer
The asset management industry stands at a fascinating inflection point. While the fundamental challenges remain familiar—market volatility, regulatory compliance, operational efficiency, and reputation management—the tools at our disposal have evolved dramatically.
The narrative you've heard about AI in asset management probably goes something like this: AI models and algorithms make better predictions, cut costs, boost efficiency. If only it were that simple. North American firms dumped $1.89 billion into AI solutions last year, with projections suggesting this figure will explode to $17.12 billion by 2033.
But these numbers, like most things in finance, hide more than they reveal.
AI (especially generative AI) is still, to a large degree, a tool in search of a problem. Adoption has seen its fair share of both success and failure. Yet, if there’s one area where AI has proven its worth, it is document processing and due diligence. Asset managers are increasingly turning to AI document automation to streamline their operations and reduce risks.
In this article:
The role of AI technology in asset management
Key automation use cases for investment firms
Best practices for successful AI implementation
The state of asset management: current landscape and emerging trends
Consider the daily juggling act of a modern asset manager: monitoring market fluctuations across global exchanges, ensuring compliance with an ever-expanding web of regulations, maintaining operational excellence, and safeguarding their firm's reputation. Each of these challenges carries its own complexity, and as McKinsey's 2024 survey reveals, 72% of organizations are turning to AI-driven solutions to navigate this intricate landscape.
The regulatory burden alone has become particularly daunting. Asset managers must now contend with the transition of Undertakings for Collective Investment in Transferable Securities (UCITS) funds to Packaged Retail and Insurance-based Investment Products Key Information Documents (PRIIPs KIDs) for retail investors, while simultaneously adapting to new Environmental, Social, and Governance (ESG) regulations and maintaining compliance with existing frameworks like the Alternative Investment Fund Managers Directive (AIFMD), Markets in Financial Instruments Directive (MiFID), and European Market Infrastructure Regulation (EMIR). These regulations require sophisticated data management and reporting capabilities, where AI can streamline compliance processes through automated monitoring and reporting systems. Add to this the pressure of rising operational costs in an inflationary environment, and the need for technological innovation becomes clear.
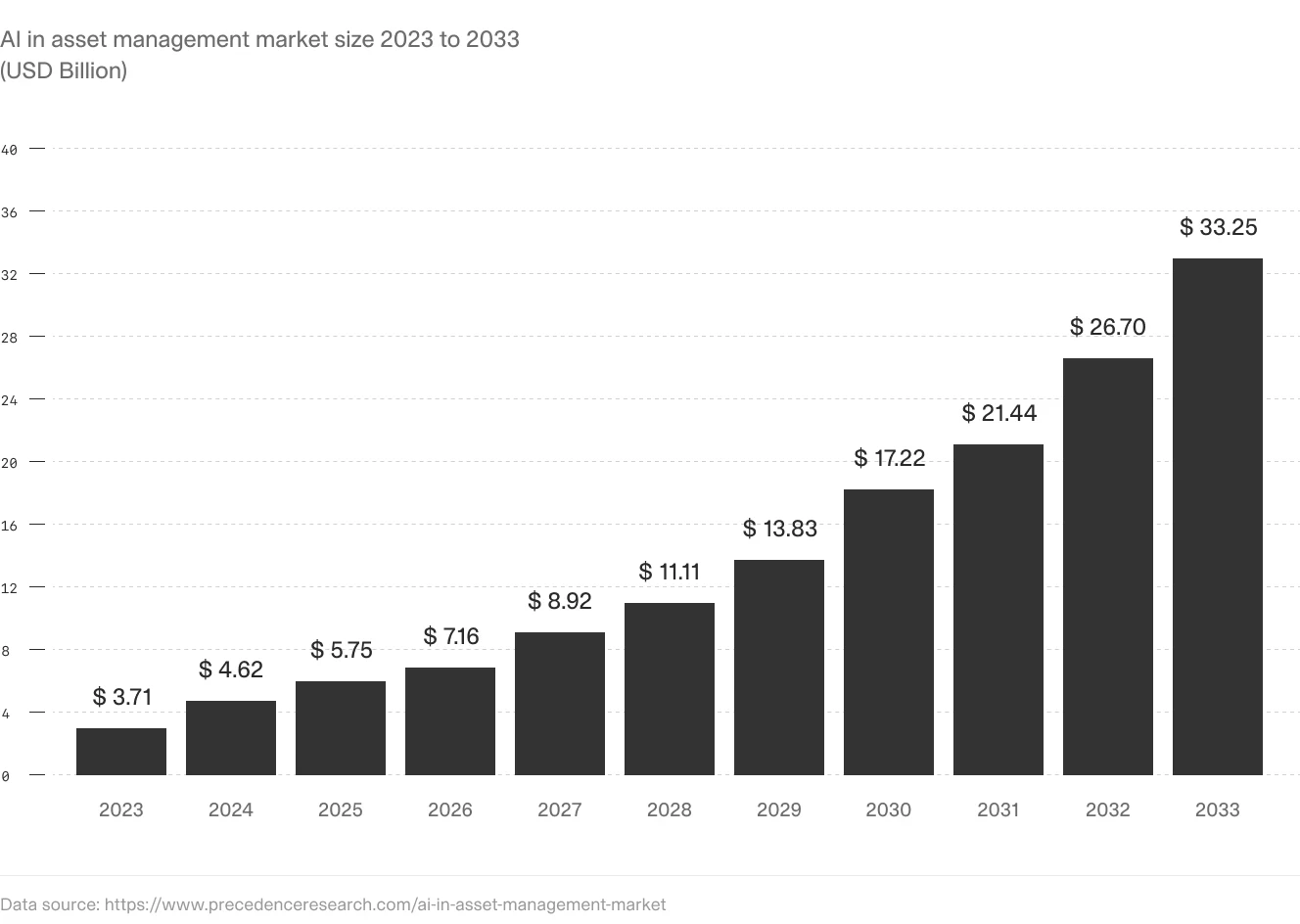
Yet amidst these challenges lies opportunity—over 90% of asset managers are already leveraging AI technologies to enhance their investment performance. These aren't merely experimental implementations. We're seeing practical applications in areas like customized client insights, sentiment analysis, and market intelligence summarization.
However, this technological transition isn't without its hurdles. Data quality issues, interpretability concerns, and ethical considerations continue to challenge widespread adoption. The key lies in finding the right balance—leveraging AI's capabilities while maintaining human oversight and judgment.
The industry's response to these challenges will likely define its trajectory for years to come. As operating costs continue to climb, firms are increasingly re-evaluating their processes and operating structures. This introspection, combined with technological advancement, suggests we're entering a new era of asset management—one where data-driven strategies and human expertise combine to create more resilient, efficient, and effective investment approaches.
Role of AI in de-risking asset management
In today's asset management landscape, de-risking isn't just about playing defense—it's about leveraging technology to create a more resilient and responsive investment strategy. As KPMG's 2024 Asset Management Industry Pulse Survey reveals, 60% of asset managers identify data integrity risks as a significant barrier to AI adoption, highlighting the delicate balance between innovation and prudence in the industry.
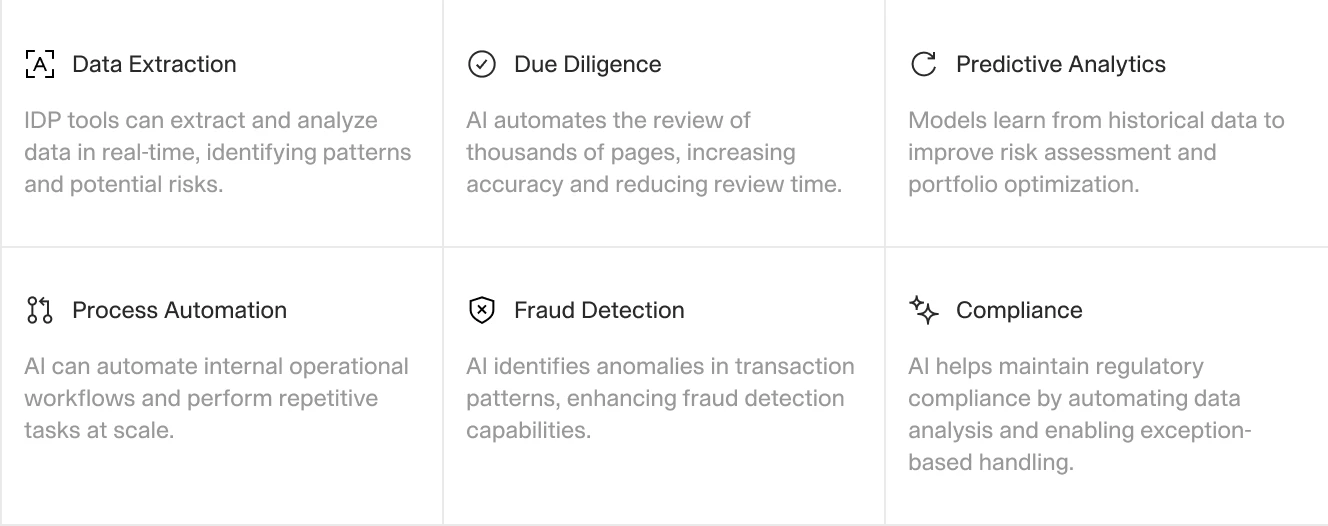
AI's role in de-risking asset management operates on multiple levels, each addressing specific challenges that traditional approaches struggle to handle effectively. The evolution of intelligent document processing has transformed how firms handle complex financial documents. All major institutions, including the International Monetary Fund, now acknowledge that AI/ML techniques enhance the efficiency of cash flow and liquidity management, automate asset class rebalancing, improve valuation and forecasting methods, and determine capital requirements tailored to individual needs.
Data extraction and analysis
AI systems can swiftly process and analyze complex financial documents, identifying patterns and potential risks in real-time—capabilities that would take human analysts significantly longer to achieve. This enables financial institutions to detect suspicious activities and optimize portfolios more efficiently. By leveraging AI's ability to handle vast amounts of both structured and unstructured data, organizations can enhance risk management strategies and improve decision-making processes.
Predictive analytics
The real magic happens in predictive analytics, where AI doesn't just process historical data—it learns from it. As Mikhail Dunaev points out, unlike traditional rules-based systems, AI models can learn and adapt over time, fine-tuning their parameters and knowledge as they are exposed to more data. This adaptive capability means risk assessment becomes increasingly sophisticated and accurate over time.
Process automation
Perhaps most importantly, AI enables personalization at scale. AI enables the creation of personalized portfolios at scale, tailoring the client experience based on individual financial goals, tax situations, and income prospects. This means risk management isn't just more efficient—it's more precise and personalized than ever before.
What makes this technological evolution particularly fascinating is how it's changing the very nature of risk assessment. Rather than simply flagging potential issues, AI systems are becoming sophisticated enough to suggest mitigation strategies and alternative approaches. This proactive stance represents a fundamental shift from reactive risk management to predictive risk prevention.
The integration of AI into asset management isn't just about adding another tool to the toolkit—it's about fundamentally reimagining how we approach risk in financial markets. As Deloitte highlights, AI provides new opportunities for enhanced decision-making in investment management, marking a shift from traditional risk management to a more dynamic, data-driven approach.
Automating due diligence with AI
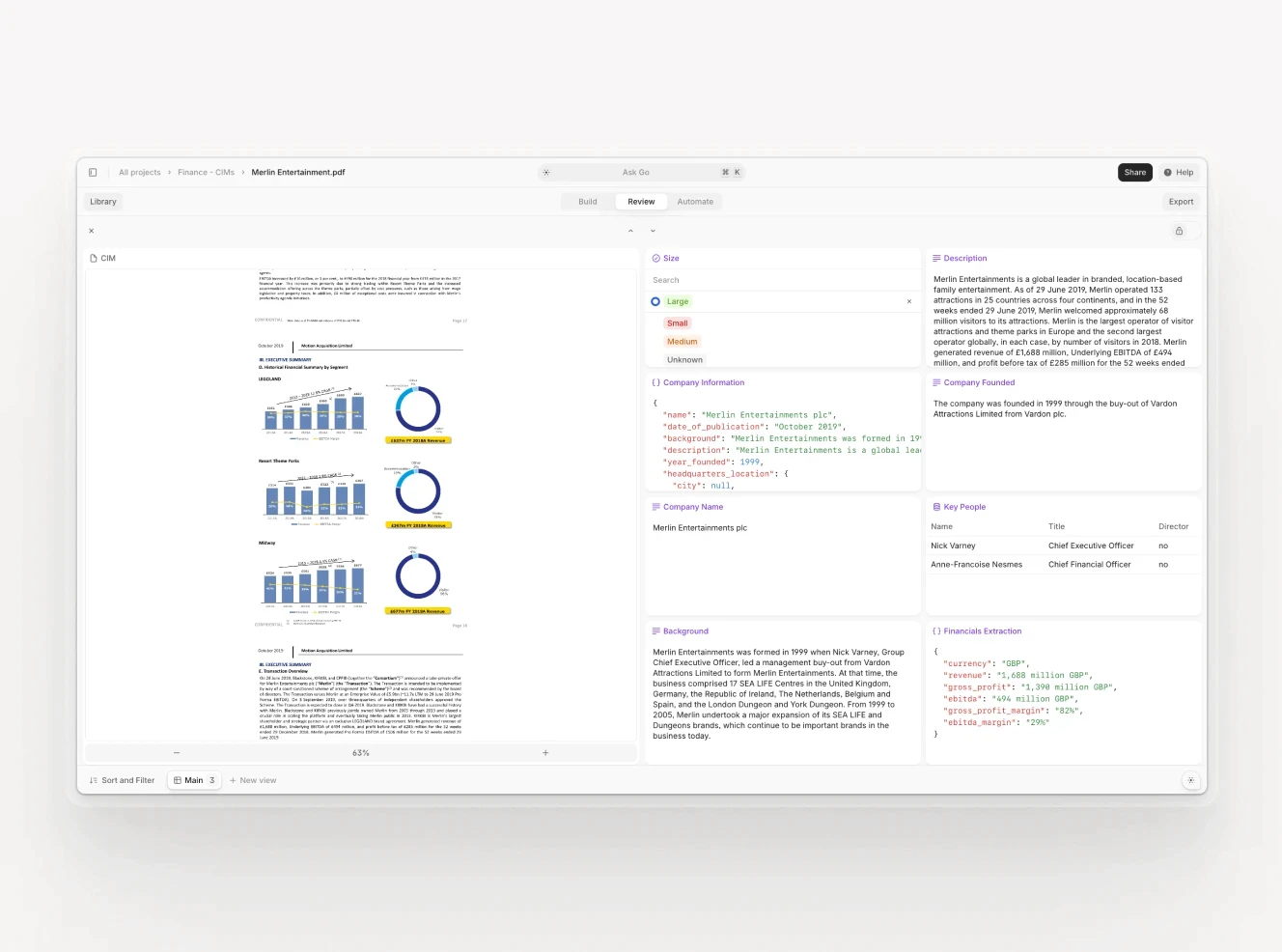
The automation of due diligence processes has transformed how asset managers approach compliance and document review. Modern AI due diligence solutions can process thousands of pages in minutes, dramatically reducing review time while increasing accuracy. AI systems can now process thousands of pages of company filings and contracts in minutes, extracting relevant information with remarkable accuracy. This capability isn't just about speed—it's about consistency and thoroughness in risk assessment.
Predictive analytics for risk assessment
Predictive analytics has evolved beyond simple trend analysis. Modern AI models process vast amounts of data to generate nuanced risk scores, incorporating everything from market volatility to macroeconomic indicators. AI algorithms can process vast amounts of data, including historical records, transactional behavior, and external information sources, identifying subtle patterns and correlations that traditional methods might miss.
Perhaps most intriguingly, AI sentiment analysis has emerged as a crucial tool in the risk assessment arsenal. AI-powered systems now scan social media, news articles, and financial blogs to gauge market sentiment in real-time. This helps in understanding market emotions and trends, which can be critical for financial risk management.
Portfolio optimization
Portfolio optimization has become increasingly sophisticated through AI implementation. The technology enables dynamic portfolio adjustments based on real-time market conditions, moving beyond traditional quarterly rebalancing approaches. AI systems continuously monitor market conditions, credit risks, and portfolio performance, suggesting adjustments before human analysts might spot potential issues.
AI tools, like automated fund performance analysis systems, can process and interpret complex financial documents, performance charts, and allocation graphs in a fraction of the time it would take human analysts. They identify actionable insights from vast datasets, flag underperforming assets, and recommend rebalancing strategies to align with evolving market trends.
Moreover, these systems improve decision-making by integrating data from multiple sources and ensuring data accuracy and consistency, which is critical for effective portfolio management. By reducing manual reviews by up to 80% and increasing productivity, asset managers can focus on high-value activities such as strategic planning and client engagement.
Fraud detection and security
In fraud detection, AI's pattern recognition capabilities have proven invaluable. The technology can identify subtle anomalies in transaction patterns or document submissions that might indicate fraudulent activity. AI can detect inconsistencies in transaction frequency, timing, or geographic origin that deviate from historical norms, flagging potential issues before they escalate.
Furthermore, AI-powered fraud detection systems contribute to enhanced security by integrating with other tools like biometric verification or KYC solutions that combine computer vision and OCR technology.
These advancements in AI-driven risk management aren't just theoretical—they're delivering measurable results. Asset managers using these tools report significant improvements in risk detection rates and substantial reductions in manual review time. The technology continues to evolve, with each iteration bringing more sophisticated capabilities to the risk management toolkit.
AI for asset managers: solutions
Asset management firms have traditionally faced challenges when implementing AI solutions, requiring extensive model training on proprietary data. While many software platforms now integrate Large Language Models (LLMs) and AI workflow automation tools for document processing, handling complex financial documentation remains challenging. V7 Go addresses these challenges by combining LLM capabilities with specialized features for financial document analysis. The platform's ability to process unstructured data—including financial statements, handwritten annotations, complex financial tables, regulatory filings, investment reports, and compliance documentation—is enhanced by features that increase accuracy and reliability. This makes it particularly suitable for asset management workflows where precision and trustworthiness of extracted information are paramount.
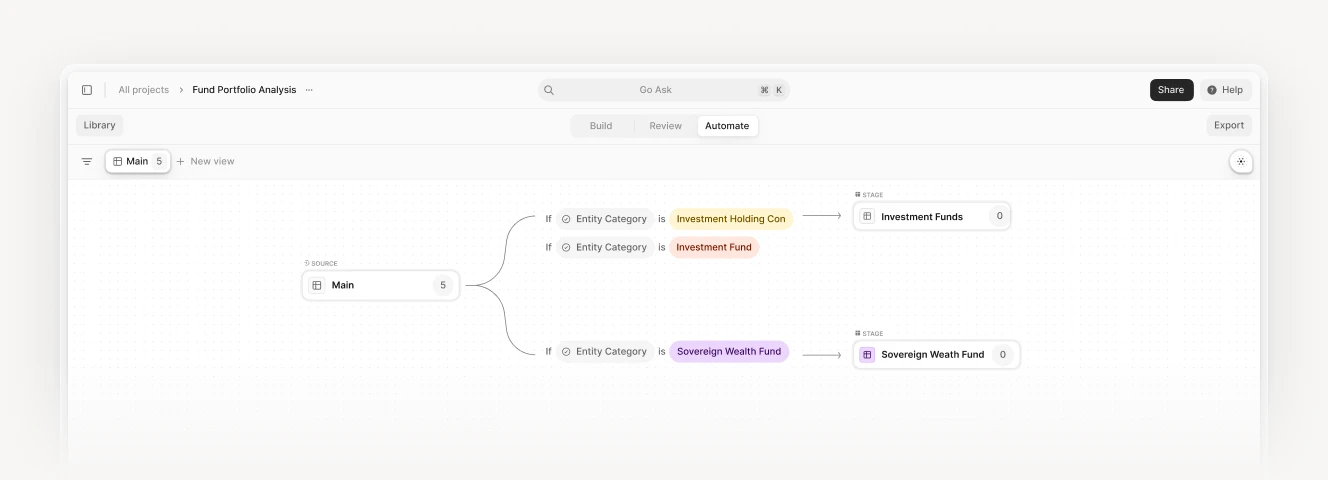
What sets V7 Go apart is its remarkable accuracy—achieving 95 to 99.9% on one-shot benchmarks, significantly outperforming other leading LLMs, RPA, and IDP software. This level of precision isn't just a technical achievement; it's a game-changer for asset managers who can't afford errors in their document processing workflows. As a leading AI platform, V7 Go delivers unprecedented accuracy in document processing without requiring extensive training data.
The platform's multimodal capabilities create a sophisticated ecosystem where OCR, Python code, and LLM compatibility work in concert. This adaptability isn't merely a technical feature—it's a strategic advantage that major players identify as crucial for asset management firms looking to stay competitive.
By incorporating advanced features and AI technologies, V7 Go stands out as a powerful tool for de-risking asset management, offering significant efficiency gains and enhanced decision-making capabilities.
Consider the practical implications: V7 Go processes financial statements three times faster while boosting accuracy by 54% compared to human analysts. This efficiency gain translates into tangible benefits for asset managers, particularly in risk management and compliance operations. The platform's ability to handle complex documents without training means teams can deploy solutions immediately, addressing urgent operational needs without the typical AI implementation delays.
The ROI becomes even more compelling when considering the broader operational impact. As Deloitte notes, AI can transform traditional cost centers into efficient operations by streamlining middle and back office activities—from trade support to reference data analysis and customer onboarding.
In practice, this means asset managers can automate the processing of diverse document types—from prospectuses to financial statements—while maintaining high accuracy levels. The ability to handle both PDF/DOC files and photos/scans of handwritten notes proves particularly valuable for processing analyst annotations and meeting notes, documents that traditionally required manual review. This automation-first approach doesn't just save time—it creates a more robust risk management framework by reducing human error in document processing and data extraction.
The impact of AI on deal-side and public markets
Private equity firms are facing a curious paradox. While only 24% currently leverage AI effectively in their operations, a striking 57% acknowledge its critical role in achieving their business objectives over the next three years. This gap between current adoption and recognized potential presents both a challenge and an opportunity for deal-side professionals.
Consider a typical private equity scenario: A mid-market firm evaluating multiple acquisition targets simultaneously. V7 Go's document automation capabilities streamline the due diligence process by analyzing financial statements, contracts, and market research—tasks that traditionally consumed weeks of analyst time. The system doesn't just extract data; it identifies patterns and potential red flags that might escape human attention during initial screenings. Private equity firms can leverage pre-built automation templates to streamline their document review processes.
The impact extends beyond mere efficiency. As KPMG notes, generative AI technologies are revolutionizing due diligence by optimizing data gathering and preparing comprehensive financial analyses. For instance, when evaluating a manufacturing company, V7 Go can simultaneously assess operational metrics, supply chain agreements, and market positioning documents, providing a holistic view that helps identify both risks and opportunities.
On the public markets side, hedge funds and traditional asset managers face their own set of challenges—particularly regarding regulatory compliance. The landscape is becoming increasingly complex, with 40% of managers expecting a 5-10% increase in compliance budgets, and another 18% anticipating the increase of 10% or more.
Many platforms for asset management address these challenges by providing AI tech capable of financial crime risk detection and compliance solutions. Their ability to adapt to regulatory changes in real-time proves particularly valuable for hedge funds managing global portfolios across multiple jurisdictions.
Moreover, the capabilities extend to ESG integration—a growing priority for institutional investors. AI providers now assist in embedding Environmental, Social, and Corporate Governance factors into investment decisions while improving reporting accuracy. This functionality becomes increasingly crucial as investors demand more robust ESG policies and compliance infrastructure.
The AI's value proposition isn't just about processing speed—it's about risk mitigation through comprehensive analysis. Whether it's a private equity firm conducting due diligence on a potential acquisition or a hedge fund ensuring compliance across multiple jurisdictions, For example, V7 Go provides a level of analytical depth and regulatory awareness that traditional methods struggle to match. Firms can now implement AI CIM review workflows that dramatically reduce analysis time while improving accuracy.
Common concerns and limitations in using AI for risk management
Experts from McKinsey note that "AI and machine-learning algorithms are often embedded in larger AI application systems, such as software-as-a-service (SaaS) offerings from vendors, in ways that are significantly more complex and more opaque than traditional models," which highlights a critical challenge in AI adoption.
This opacity—often referred to as the "black box" problem—presents a significant hurdle for financial institutions where transparency and accountability are paramount.
The complexity of AI systems shouldn't equate to inscrutability. While some platforms offer appealing user interfaces that mask underlying complexity, this simplification often comes at the cost of control and transparency. Financial institutions need solutions that balance usability with visibility into AI decision-making processes.
Also, consider reliability metrics: modern AI systems achieve impressive accuracy rates in anomaly detection and risk scoring—often exceeding 95% in controlled environments. However, these statistics only matter when institutions can verify and validate them independently. V7 Go's approach stands out by providing detailed performance metrics and allowing users to understand how the system arrives at its conclusions, rather than presenting a mere "trust us" interface.
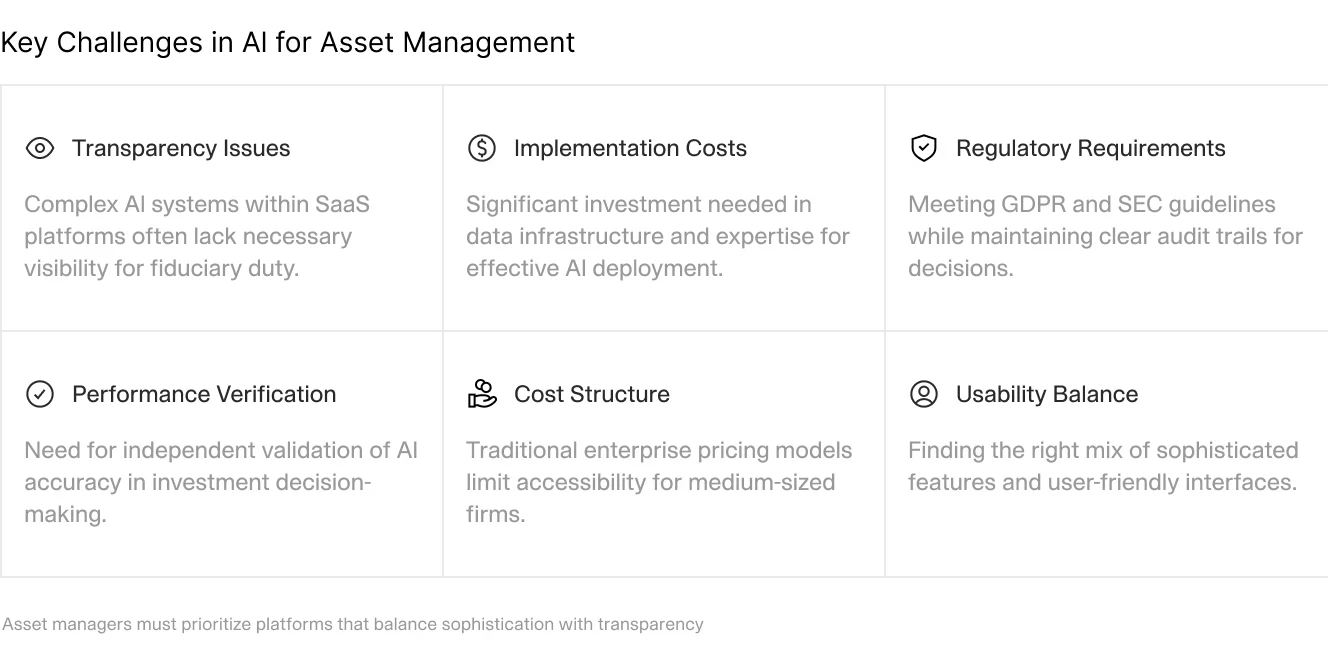
The cost barrier to AI adoption remains significant, with traditional implementation requiring substantial investments in data infrastructure and expertise. A recent innovation in this space is the "no-training-data" approach, which V7 Go pioneered to address this challenge. This methodology allows institutions to deploy AI risk management solutions without the traditional overhead of collecting and labeling vast datasets—a process that typically consumes 60-80% of AI project timelines.
Pricing models for AI solutions often reflect legacy enterprise software paradigms, with rigid tiers and long-term commitments. V7 Go's scalable pricing represents a departure from this approach, offering usage-based pricing that allows institutions to start small and scale as needed. This model particularly benefits medium-sized firms that previously found themselves caught between oversimplified consumer solutions and enterprise platforms priced beyond their reach.
The ethical and regulatory landscape adds another layer of complexity. Financial institutions must navigate frameworks like GDPR in Europe and emerging SEC guidelines for AI deployment in the United States. These regulations increasingly emphasize explainability—the ability to understand and articulate how AI systems arrive at their decisions. This requirement aligns with the broader industry trend toward transparent AI systems that can be audited and validated.
Compliance frameworks aren't just regulatory checkboxes; they're essential guardrails for responsible AI deployment. Asset managers, in particular, must ensure their AI systems align with fiduciary responsibilities. This means implementing systems that not only perform well but also provide clear audit trails and decision justification—features that distinguish professional-grade platforms from consumer-level AI tools. Modern AI contract review solutions provide transparent decision-making processes that satisfy regulatory requirements.
The path forward lies in selecting platforms that offer the right balance of sophistication and transparency. While the allure of simple, turnkey solutions is strong, financial institutions should prioritize platforms that provide:
Detailed performance metrics and monitoring capabilities
Customizable risk thresholds and decision parameters
Clear audit trails for regulatory compliance
Scalable pricing that aligns with institutional growth
Robust documentation and support for implementation teams
These considerations ensure that AI adoption in risk management remains both practical and responsible, avoiding the pitfalls of opaque systems while maintaining the efficiency gains that make AI adoption compelling in the first place.
How to get started with AI for de-risking investments
The path to AI implementation in asset management isn't about chasing the latest tech buzzwords—it's about methodically identifying and addressing your firm's specific risk factors. As many experts point out, risk management should be integrated into the entire lifecycle of AI model development, rather than being an afterthought.
Begin with a comprehensive risk assessment. Your checklist should include:
Market volatility exposure
Fraud vulnerability points
Regulatory compliance gaps
Data quality and integrity risks
Operational inefficiencies
Model bias potential
When selecting an AI platform, prioritize solutions that offer seamless integration with your existing infrastructure. Ensure the AI platform can integrate seamlessly with your existing Enterprise Asset Management (EAM), Resource Planning (ERP), and Field Service Management (FSM) systems," advises Virtualitics in their implementation guide.
The NIST AI Risk Management Framework provides a structured approach to implementation. It recommends establishing a cross-functional team and developing a strategy for framework adoption through four core functions: Govern, Manage, Map, and Measure.
Staff training deserves particular attention. Your team needs to understand not just how to use AI tools, but how these tools fit into their existing workflows. Consider implementing:
Staged rollouts to minimize disruption
Regular feedback sessions
Hands-on training workshops
Clear escalation protocols
Watch for potential bias in your AI models. As many specialists point out, AI models can be prone to bias, which can lead to unfair decisions. To mitigate this, it is essential to implement controls during the analytics-development process.
Remember: successful AI implementation isn't about replacing human judgment—it's about augmenting it with data-driven insights while maintaining robust risk controls. Teams can begin with workflow templates designed specifically for legal document processing before developing custom solutions.
Conclusion
As we've explored the transformative capabilities of AI in asset management, one thing becomes crystal clear: the technology isn't just another tool in the box—it's reshaping the very foundation of how firms operate and manage risk. GenAI can facilitate true cross-enterprise knowledge sharing, reducing key person risk, and improving information sharing across asset classes. This fundamentally alters how asset management firms handle their most precious resource: information.
The implications are particularly striking for smaller firms. As BCG notes, GenAI creates a "synthetic army of research analysts at a low marginal cost," which effectively means democratizing capabilities that were once the exclusive domain of industry giants. This levels the playing field in ways previously unimaginable.
However, success with AI isn't guaranteed without correct identification and understanding of tasks that can be automated.
Whether you're looking to automate due diligence processes, optimize portfolio management, or enhance risk assessment capabilities, V7 Go’s AI document processing software offers a risk-free pathway to explore these possibilities. We invite asset managers to schedule a consultation or demo to discover how our platform can address your specific needs—from streamlining document processing to implementing sophisticated risk management protocols.
The future of asset management is here, and it's powered by AI. The question isn't whether to embrace these technologies, but how quickly you can implement them to stay ahead of the curve.
References
AI in Asset Management Market Size, Share, and Trends 2024 to 2033
Derisking AI by Design: How To Build Risk Management Into AI Development
The Generative AI Tipping Point: Global Wealth and Asset Management 2023 Report
Generative AI in Asset and Wealth Management: The Art of Possible
Advances in Artificial Intelligence: Implications for Capital Market Activities
The Good, Bad and Ugly of Using AI in Financial Risk Management
Artificial Intelligence: The Next Frontier for Investment Management Firms
AI in Risk Scoring – the Next-Gen Risk Management Paves a Path for Businesses
Transforming Deal Execution With Generative AI
The Impact of Digital Transformation and Global Regulatory Changes on Fund of Funds Strategies